Securing AI Clusters, Juniper’s Approach to Threat Protection with Juniper Networks
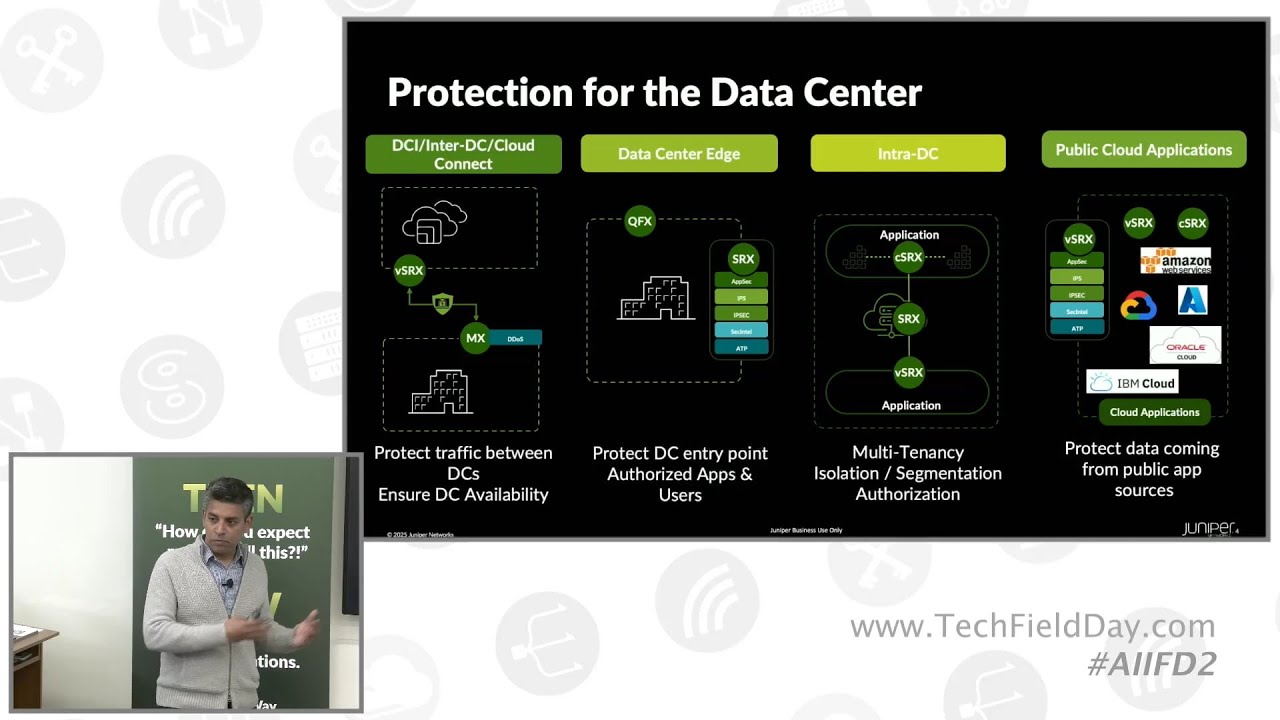
Securing AI Clusters – Juniper’s Approach to Threat Protection
AI clusters are high-value targets for cyber threats, requiring a defense-in-depth strategy to safeguard data, workloads, and infrastructure. Kedar Dhuru highlighted how Juniper's security portfolio provides end-to-end protection for AI clusters, including secure multitenant environments, without compromising performance. The presentation addressed the challenges of securing AI data centers, focusing on securing WAN and data center interconnect links and preventing data loss, which are amplified by the increased scale, performance, and multi-tenancy requirements of these environments.
Juniper's approach to securing AI data centers involves several key use cases. These include protecting traffic between data centers, preventing DDoS attacks to maintain uptime, securing north-south traffic at the data center edge with application and network-level threat protection, and implementing segmentation within the data center to prevent lateral movement of threats. These security measures can be applied to traditional data centers and public cloud environments with the same functionalities and adapted for cloud-specific architectures. Juniper focuses on high-speed IPsec connections to ensure data encryption without creating performance bottlenecks.
Juniper uses threat detection capabilities to identify indicators of compromise, including inspecting downloaded software and models for tampering and detecting malicious communication from compromised models. Their solution employs multiple firewalls, machine learning algorithms, threat feeds to detect and block malicious activity, and a domain generation algorithm (DGA) and DNS security to protect against threats. The presentation also highlighted Juniper's new one-rack-unit firewall with high network throughput and MACSec capabilities, along with multi-tenancy and scale-out firewall architecture features.
Presented by Kedar Dhuru, Sr. Director, Product Management, Juniper Networks. Recorded live in Santa Clara, California, on April 23, 2025, as part of AI Infrastructure Field Day.
You’ll learn
How to safeguard AI infrastructure, data, and workloads
Securing multitenant AI cloud environments
Who is this for?
Experience More
Transcript
0:00 good morning everybody my name is Kedar
0:02 Zuru and um I'm here to talk to you uh
0:06 through some of our security use cases
0:08 for uh AI data centers um I lead product
0:11 management for uh Juniper security um
0:14 both product development as well as um
0:17 helping with um you know building
0:20 security strategy for Juniper security
0:22 as well as how we embed security across
0:25 um all of our different product lines to
0:28 ensure that we can deliver things like
0:30 secure data centers um to our customers
0:34 um part of the agenda is to look into
0:38 some of the use cases when it comes to
0:40 AI data centers uh you know what um
0:43 Proful uh covered a few things this is
0:45 about how do we help secure the VAN and
0:48 data center interconnect links uh we'll
0:50 talk about um uh you know using the
0:53 example of a couple of um uh threats how
0:56 do we how can we help focus on the data
0:58 and prevent data loss uh and then one of
1:01 the challenges we have when it comes to
1:04 uh AI data centers is the fact that um
1:07 you know you're everything gets
1:08 amplified got more scale more
1:10 performance um more uh tenency um
1:14 requirements and so how can what have we
1:17 done to kind of help uh address some of
1:19 these challenges so that's kind of the
1:22 structure of what um um you know we'll
1:25 we'll follow today
1:27 i think that's key can you say that
1:28 again more scale more latency and what
1:30 was the third one multi-tenency okay um
1:32 and I think this is not new i think both
1:34 Vicram and Prul have covered these to a
1:36 large extent in in the first two
1:37 sections so um they they apply to
1:40 security as well and then we've done a u
1:42 we've we've implemented um higher
1:45 performance higher scale and
1:47 multi-tenency capabilities into um our
1:50 security use cases too
1:54 um so when it comes to uh AI data
1:57 centers it's about uh being able to
2:00 deliver or or run data centers closer to
2:04 uh where your data where your users are
2:06 where your uh applications are running
2:08 you want to have um uh data centers be
2:12 uh local and regional sometimes you you
2:15 want to be able to train your data in
2:16 one location move it closer to where you
2:19 can um you know uh deliver um
2:21 inferencing may maybe it's through a
2:23 public cloud or it's in a collocation
2:25 facility but essentially you have uh
2:28 different locations where your data is
2:30 located different locations where your
2:32 data centers are going to run um and and
2:35 um um access this data um from a
2:39 protection and a security standpoint um
2:42 essentially you have three or four
2:44 different um use cases many of these
2:47 apply to standard um uh data centers and
2:50 when we look at AI data centers they uh
2:53 don't necessarily uh I mean they they
2:55 evolve uh but we break them down into
2:57 four different areas you've got data
2:59 centers that need to connect to each
3:00 other and so you want to be able to
3:02 protect traffic um between those
3:05 different data centers you want to
3:06 ensure that the data centers are up and
3:08 running um you know somebody's looking
3:10 to uh bang on your door and take that
3:13 data center service down you want to uh
3:15 ensure that that doesn't happen so
3:17 things like DOS protection become
3:19 important uh the next use case is um as
3:23 you are allowing traffic users uh or
3:27 other um um kinds of traffic to get in
3:29 and look at your applications you want
3:31 to be able to secure that knocks out
3:33 traffic so you've got the data center
3:34 edge or data center parameter firewall
3:36 which is where you want to focus on the
3:38 application you want to focus on network
3:40 level threats you want to be able to
3:42 weed out the bad stuff with things like
3:44 um you know uh threat feeds you want to
3:46 be look um able to identify uh zero day
3:50 uh attacks or zero day threats in in
3:52 some way and so how do we help do that
3:55 um the third one is once you get inside
3:58 the data center you want to be able to
4:01 um uh ensure that you can do um uh
4:04 segmentation if you've got something
4:06 compromised it is not moving laterally
4:08 within your organization and um um you
4:11 know compromising other applications um
4:14 you know or or I think one of the
4:16 examples Proful used earlier was um
4:19 going from one tenant to another tenant
4:21 from one user's data compromising
4:23 somebody accessing somebody else's data
4:25 so you've you've got things like the
4:27 data center core firewall uh addressing
4:30 your interdc use cases and then as we um
4:35 you know when we looked at the
4:37 architecture or the um the highle
4:39 diagram for uh data center um and how
4:42 they're distributed you may also want to
4:45 take some of your data into the public
4:46 cloud and then how do we bring these
4:49 same use cases into the public cloud i
4:51 don't think it's anything
4:53 um new it is still the same data center
4:55 parameter firewall data center core
4:57 firewall uh functionality um it just
5:01 needs to uh it it needs to be adapted to
5:03 operate in a public cloud environment um
5:05 break up between using the AWS
5:07 terminology break up between different
5:09 VPCs um ensure that you've got secure
5:12 connectivity into the cloud and so on
5:14 same thing applies to Azure GCP or
5:16 wherever else you're running your data
5:18 um and workloads
5:20 um let's look at u two things one is
5:24 from a VAN and data center interconnect
5:26 standpoint you want to ensure that every
5:29 u data center that is connected or all
5:31 of the data centers that you have
5:33 connected um have a link that is in some
5:36 way protected so the two things we do
5:38 here and what we have done is um we have
5:42 ensured that um if you are doing some
5:45 kind of a uh connection between let's
5:48 say a firewall the srx is our product uh
5:51 our firewall product line the MX is our
5:53 data center kind of gateway and routing
5:55 uh product line if you do have a
5:57 connection how do we ensure that that uh
6:00 data center is up and running so we we
6:02 integrated uh TDOS mitigation uh
6:05 capabilities into our uh data center
6:08 gateways whether that's MX or the PTX
6:10 product line uh we can detect uh
6:13 somebody doing things like volutric DOS
6:16 attacks meant to take down your
6:17 applications or your um um data center
6:21 links as a whole um identify those and
6:24 then um start blocking against those DOS
6:27 attacks there the second thing is when
6:30 you do have these connections many times
6:32 those connections may not have a
6:34 dedicated link they may be going out
6:36 over a high performance public link um
6:39 the public internet and so you want to
6:41 ensure that when you're moving data your
6:43 customers are bringing data to a uh one
6:46 question before you go on to that Jack
6:47 Paul paradigm technic so you you're
6:50 making a distinction there on public
6:52 links is there a reason you're doing
6:53 that or is she behind a firewall are you
6:55 looking at zero trust at all in this
6:57 envir so we are looking at zero trust
6:58 and I'll come to that um but in this
7:01 particular case we're just looking at
7:02 the van link uh you're looking at an
7:05 open public link uh where you if you're
7:07 transferring data you may want to bring
7:09 in a level of um encryption that's where
7:12 the IP sec piece comes in PP and IP sec
7:15 and um many times and you know when it
7:18 comes to uh AI data centers it's moving
7:21 large volumes of data uh some of that
7:23 needs to happen at high performance but
7:25 if you're IP sec links are few gigs
7:28 you're going to act as a a choke point
7:31 so some of the things we did was um
7:34 whether it's the SRX which is running as
7:36 a firewall or things like the MX which
7:38 are your data center gateway we can do
7:41 uh high high speeded IPS connection um
7:43 you know all the way up to um single
7:45 flow being 300 gigs right um so whether
7:49 you have a public link or even if it's a
7:51 a private link if you do have a need for
7:54 encryption you do get um that high-speed
7:56 capability all right does that answer
7:59 your question yeah I just you know in
8:01 the context of AI Is it performance
8:04 that's driving the need for not using
8:07 encrypted links versus encrypting your
8:09 data all the time right so from a
8:11 general data security point of view we
8:12 want to encrypt all links all the time
8:14 you want to link encrypt links all the
8:15 time right yes yes and and we can help
8:19 do that right um I was just being you
8:24 know it it's up to the it's up to the uh
8:27 customer um the the operator how they
8:30 want to do the encryption i I would
8:32 recommend doing it all the time but
8:34 that's me you know you might have a
8:36 different design okay so it's flexible i
8:38 just want to make sure that that that
8:40 there wasn't some specific reason we
8:42 were doing No no no it's uh it's a
8:45 choice but uh we made it easy and we
8:48 built it in the product so um if you had
8:51 to turn it on it's it's it's it's a
8:53 couple of lines code yep right so it's
8:56 it's there and um I I'll come to this in
9:00 the last slide but essentially um if if
9:03 you're doing a few gigs of encryption
9:05 most products and most firewalls can do
9:07 that yeah um if you need um you know
9:11 what happens with um AI data centers
9:13 you're moving large volumes of data you
9:16 your strain on your um IPSec connection
9:19 is also not a couple of gigs could be
9:20 tens could be you know multi- tens of
9:23 gigs and so you need higher performance
9:26 that's available as well gotcha thank
9:28 Right so like I said single flow I think
9:30 because we are using um uh we built this
9:33 into the Juniper ASC to be able to do um
9:36 IPSec that's looking at 300 gigs of um
9:40 uh uh single tunnel performance so
9:44 you've got that wide range
9:47 um when it comes to um security for AI
9:52 data centers uh I think um um you know
9:56 I'm just using this slide uh to level
9:58 set you've essentially got your backend
10:00 networks you've got your private
10:01 front-end networks and and things like
10:03 your public frontend networks um when we
10:06 break these down and we look at uh some
10:08 of the different um security use cases
10:12 that show up there you've got things
10:13 like data loss data contamination in the
10:15 backend networks you may have um you
10:18 know you don't want uh intermingling of
10:20 data that's where the multi-tenency
10:22 piece comes in on the private front-end
10:24 networks you've got obviously you know
10:26 if you've got something compromised if
10:27 you've got a compromised machine
10:29 learning model which is what the example
10:30 we'll see you have um u IP theft or you
10:34 know data loss that that can potentially
10:37 happen uh and and you've got tampered
10:39 models that you want to be able uh to
10:41 detect and then finally on the public
10:43 front end you want to allow you know the
10:45 right kind of user authentication you
10:47 want to do uh intrusion prevention you
10:49 don't want somebody to come in and you
10:52 know um uh um
10:55 uh waste your resources uh so these are
10:58 the different use cases that we we uh
11:00 were looking at uh from a security
11:03 standpoint for the different kinds of
11:05 networks um we're going to use
11:07 explaining these at a higher level so
11:09 backend those could be hosted they could
11:11 be in your data center they could be in
11:12 your data center if you want to run
11:14 training models they you could be using
11:16 somebody else's data center where you
11:17 move data run uh or you're running your
11:19 training models right so you could be
11:21 using an Google cloud um AI data center
11:25 as a service in videos and then so
11:27 private fronted what do you mean by
11:29 private front end and public fronted
11:31 okay uh so private uh front end is
11:34 essentially uh your data centers where
11:36 you bring you you've already got um you
11:38 you you're using models you're bringing
11:41 them uh down an LLM model and you're
11:43 running it against your data set to um
11:46 you know um um whe whether it's within
11:49 your organization to be able to um uh do
11:52 analysis uh for example on the threat
11:55 research side uh we may we may be using
11:57 a uh LLM model for you know doing threat
12:00 analysis um we we may bring different
12:02 kinds of data we may bring data from uh
12:05 our honeypot information we may bring
12:06 data from customer um submitted
12:08 information we may bring publicly
12:10 available information so that's where
12:12 we're running all of our uh inferencing
12:14 and and um um to get the results and
12:18 then public front end is if you're
12:20 running um uh an application that you
12:23 want to um have as a front-end app this
12:27 could be just any other kind of public
12:30 app that's connecting to um uh an LLM
12:34 based service on the back end uh and you
12:37 allow a large number of users or um even
12:41 um publicly registered users access to
12:43 uh those applications I guess so either
12:46 coming in or going out to the public
12:47 okay like a chat front end interface and
12:50 private is onrem intraco company intra
12:54 company or within your organization okay
12:56 thanks so other than trly bait other
12:59 than
12:59 tracking movement of data
13:04 um how else are you detecting the data
13:09 threat um um especially when you talk
13:12 about things like model t correct so
13:16 that's the example I was going to use uh
13:19 in the next slide so next slide okay
13:21 well that set it up well done way to go
13:24 camera we we we talked about this
13:27 earlier um but um essentially I mean the
13:30 example we've I think Proful brought
13:32 this up we've probably heard about
13:33 you've probably heard about this in the
13:35 news is um you know things like publicly
13:37 available marketplaces like hugging face
13:40 um um are are um exist to be able to
13:44 share and and you know um different
13:46 kinds of models what you um are doing is
13:49 a user is probably downloading some kind
13:51 of a model to their um private front end
13:54 in this particular case to be able to
13:55 run against their data sets um and let's
13:58 say the model is compromised i think
13:59 that's one of the use cases that's one
14:01 of the things you were asking right you
14:04 Yes i'm
14:05 more saying okay so that's I'm bringing
14:08 a model that's already been compromised
14:09 in that's a totally different thing than
14:13 one of the things that I'm very worried
14:14 about is that once I have created the
14:17 model and I've deployed the model
14:19 somebody goes in and alters that code
14:22 and how would you know how would you
14:24 know that's the one that scares the crap
14:26 out of me is because I'm like "Okay so I
14:28 I'm putting out these models that are
14:30 running let's say at the edge correct?"
14:33 You know water monitoring water
14:35 monitoring electricity or whatever and
14:38 it's at that edge that somebody goes in
14:40 there and does something i'm going to
14:42 about somebody right whatever um and
14:45 that's kind of a place that so when
14:48 somebody goes in and tries to tamper
14:50 with a model or any application there's
14:52 there's potentially a few things
14:55 happening one of those is um Oh I'm
14:59 sorry i wasn't even tapping all that
15:01 brilliance away
15:04 still here a little bit
15:07 go ahead do you want to reask the
15:09 question okay so the the question is
15:11 you've got a model you've trained the
15:13 model you've deployed the model and
15:15 somebody goes in and alters the model
15:17 and um how do
15:19 you protect against that right so um
15:24 from from a network standpoint that
15:25 basically means uh a couple of things is
15:28 when you've got a model uh that is um uh
15:31 tampered on that look that's looking at
15:34 um your your your data and if it's doing
15:37 nothing else one of the challenges there
15:39 is um is is that uh giving you the right
15:42 outcomes for um the data that you had
15:45 trained the model for the second one is
15:47 if you have tampered and played around
15:49 with the model somebody's looking to do
15:50 something with it potentially
15:53 exfiltrate data or or uh communicate
15:56 with and give access to somebody outside
15:58 the network um uh a backdoor into the
16:01 network and so those are things that we
16:03 can look at um and we can uh identify to
16:07 see um malicious activity or malicious
16:09 behavior we can either block those or we
16:12 can report on those that allow you to go
16:14 in and investigate further um so that's
16:18 what we're looking at from both of these
16:19 use cases in this particular case I'm
16:21 bringing in a compromised model i bring
16:23 that onto my uh network and essentially
16:26 when I install that that uh model is um
16:30 looking to open a back door to somebody
16:33 that's giving them access to my network
16:36 so how do we detect some of those
16:37 different things uh so that we can stop
16:40 you from bringing in a bad model um
16:43 identify a bad model if it has already
16:45 been brought in and be able to um um
16:48 minimize uh the impact of that um keep
16:51 it contained so those are the two
16:53 different use cases and essentially the
16:55 first thing is if you're bringing in a
16:57 bad model itself u we can have so we
17:00 have two firewalls in this particular
17:01 example you've got firewall is it is it
17:03 really is it fair to say that what
17:04 you're really doing is you're detecting
17:06 that your your threat detection what
17:08 threat protection is looking for
17:10 specific IOC's indicators of compromise
17:12 in this case you're looking at things
17:14 that are network related yes as
17:16 indicators of compromise so you're
17:17 looking for if you see somebody coming
17:20 in to look at the model that isn't doing
17:22 it or the that shouldn't be looking at
17:25 the model coming across the network so
17:26 in this case you're downloading a model
17:29 right and um just to set this up uh we
17:32 using two of those four use cases I
17:34 mentioned earlier you're using a data
17:36 center parameter firewall and you're
17:37 using a data center core firewall right
17:40 right and if you're downloading a model
17:41 that is compromised um that firewall is
17:44 capable of detecting uh a compromised
17:47 model you know we we've built in um a
17:50 machine learning algorithm onto the
17:51 firewalls itself that is capable of
17:53 looking at um um indicators in the file
17:57 that is being transferred so if you've
17:58 got a model that uh somebody has
18:00 tampered with um or whether it's an AI
18:03 model or it is an application that has
18:05 been tampered with um there are certain
18:08 signatures in the file that is being
18:11 downloaded itself that um indicate um
18:15 that
18:16 a somebody has tampered with uh with it
18:20 for example if they're using a
18:23 um old unsupported version of Visual
18:26 Studio to make some of the changes
18:28 that's one example and then you've got
18:30 other markers that we can look for so
18:32 when you aggregate all of those that
18:34 gives you know there's less chance that
18:36 somebody good is using bad software to
18:40 create you know a product so you're
18:42 doing more than just looking at network
18:45 yes intrusion you're looking at the
18:46 actual more than just network effects
18:49 network intrusion detection and some of
18:50 those types of things so you're actually
18:52 doing an inspection of the data being
18:54 downloaded and saying this is being
18:57 downloaded in this I'm sorry of the
18:58 software software sorry yeah software
19:00 being downloaded and saying we expect a
19:03 model to look like this and this model
19:04 has signatures in it that it shouldn't
19:07 have of one form or another that
19:08 indicators that it's been tampered with
19:10 yes and that can give you um a lower
19:14 level of probability which you may want
19:16 to alert on and a higher level of
19:18 probability that you may want a
19:19 threshold that you may want to block so
19:21 that's one use case yep bringing in
19:24 something now let's say you brought it
19:26 in anyhow before you had security in
19:29 place or through another mechanism and
19:32 you've now got um that model that is
19:35 compromised it's trying to connect and
19:38 comm that's it i have five minutes okay
19:41 um great let's speed up then solution
19:45 uh so that model that's trying to
19:46 communicate with um u a thread actor
19:49 infrastructure this typically happens i
19:51 mean why is somebody compromising your
19:53 model they want your data they're not
19:55 doing it for fun they they they want
19:56 your data they want to be able to get
19:58 into your network so that they can move
20:00 laterally so they can get to something
20:02 else uh if it is uh critical
20:04 infrastructure for example they may want
20:06 to you know take over more and more
20:08 applications in your critical
20:09 infrastructure so if that's going out
20:12 then um that same data center parameter
20:15 firewall um uh has um several things
20:18 it's got direct signatures like command
20:20 and control feeds that we know where
20:22 there are known thread actors IP
20:25 addresses or infrastructure IPs that we
20:27 can block against um typically you know
20:30 these models are looking at different
20:31 IPs looking at connecting to different
20:34 kinds of um uh domains uh so things like
20:37 domain generation algorithms can or DNS
20:40 security can be used to help protect
20:41 that we built another machine learning
20:44 um um algorithm called encrypted traffic
20:46 insights even if your connection to um
20:49 uh the the thread infrastructure is uh
20:52 encrypted there are a lot of markers in
20:55 that encrypted tunnel um the IP address
20:57 it's connecting to the handshake the
20:59 certificates all of these the beaconing
21:01 behavior that is there all of this can
21:03 be used to save the high level of
21:06 confidence that that model is
21:07 compromised is talking to some kind of a
21:09 threat um uh actor's infrastructure and
21:12 we need to block that or we need to
21:13 report on that and then finally um one
21:16 of the things um uh that happened with
21:19 the hugging face um model um corruption
21:22 was a lot of the models put in um uh
21:25 rever um like a reverse shell connection
21:28 and so being able to detect those uh can
21:31 help you identify a compromised model in
21:33 place or compromised application in
21:35 place and help uh block
21:38 that the third thing everything's come
21:41 in everything's running now you've got
21:43 uh traffic flowing from this model not
21:45 just to your data but you know trying to
21:47 go somewhere else that's where the data
21:48 center core firewall comes into uh play
21:51 and uh it is able to um um you know uh
21:54 identify traffic moving from one uh
21:57 model to a different data set uh that
21:59 movement across um multiple tenants uh
22:02 of data and you're able to help bring
22:04 that in uh if we have detected that my
22:08 model is compromised we have a feed that
22:11 we um that we can share directly with
22:13 the QFX switches in the data center
22:15 called the infected host feed so if I've
22:18 detect um um my firewall here has
22:21 detected my uh IP address to be
22:23 malicious I can share that with the QFX
22:25 itself and uh you can help contain and
22:28 block um the the compromised uh IPs or
22:31 servers uh within that environment so
22:33 that's more about um you know reducing
22:37 the spread there uh and finally um you
22:40 know we have introduced uh things like
22:43 uh multi-tenency capabilities on the
22:44 firewall itself so uh we can help
22:47 prevent um uh or or block an
22:50 unauthorized application from talking to
22:52 somebody else's uh data so again this is
22:55 more about containment how would this
22:57 protect against um like the people who
23:00 want to tamper with your models not to
23:02 exfiltrate data not to go other places
23:04 but to poison your business decisions
23:07 and that kind of stuff internally and
23:09 then that sort of also relates to the
23:10 second question about what about insider
23:13 threats doing all the same so um the
23:16 insider of threads again that's where
23:17 you have the data center and so I'll
23:19 just take that one that's where you have
23:20 the data center core firewall which
23:22 essentially is you have access to this
23:26 application not to this application so
23:28 um that's more about where you have
23:30 access to that's implementing things
23:32 like a zero trust um security approach
23:35 uh so I'm the insider I have access here
23:38 but I'm trying to go in here but I don't
23:39 have uh access to an application X um
23:43 that's where um zero trustbased policies
23:47 would uh apply so things like uh like a
23:51 universal zero trust architecture uh
23:53 integrating with your um active
23:56 directory so you can set the right kind
23:58 of permissions that's where it would
23:59 apply and I think the other one is I do
24:02 have a compromised model i'm giving you
24:04 bad data i'm giving you bad response um
24:07 that one's uh a little bit harder from a
24:10 network and firewalling point of view uh
24:12 but we are looking at ways where um um
24:16 you know to to we're kind of that's in
24:19 the research phase we we understand
24:21 that's a challenge but we you know um a
24:24 networkbased approach necessarily can't
24:26 solve that that's more between the model
24:28 and the data so you need to look at the
24:30 data level you need to understand model
24:32 context so those those are a little bit
24:34 different so to get the hook u will we
24:37 have time to get more qu ask more
24:39 questions it it's I I did have a
24:42 question following up on uh Karen's
24:44 question which is uh how do you handle
24:47 um what would be unprotected Ethernet
24:48 traffic like RDMA traffic um can we take
24:53 that later i don't have enough right now
24:56 okay um can I get two more minutes to
24:59 finish up you need to finish up in the
25:01 next 30 seconds okay 30 seconds um so
25:04 everything gets amplified in an AI data
25:06 center we we talked a little bit about
25:08 this it's much higher performance it's
25:09 much higher scale and obviously
25:11 multi-tenency um so we have been
25:15 thinking about these challenges for a
25:16 while we did um take some steps to get
25:20 there the first thing is we started
25:22 shipping uh a new uh one rack unit
25:24 firewall that's uh that can do 1.4
25:27 terabs of uh network throughput with
25:29 multiple 400 gig 100 gig links to allow
25:31 you to connect into your data center
25:33 fabric itself or AI data center fabric
25:35 all of those ports can do max um and
25:38 then the other thing we did was uh we we
25:42 uh introduced machine learning and uh
25:44 algorithms uh into the software code
25:47 itself to be able to detect um um you
25:50 know AI based um threats things like
25:53 using AI to be able to detect malicious
25:55 um um or compromised models uh we
25:59 introduced uh integration directly into
26:01 an IP fabric like EVP and VXLAN so we
26:03 can make it easy to integrate security
26:05 uh into your data center fabric that
26:07 allows for multi-tenency that allows for
26:09 multi-tenency across a data center
26:11 stretch and then finally I heard a lot
26:14 of questions earlier about scale up
26:15 versus scale uh out uh that is um so we
26:19 introduced a concept called scaleout
26:21 firewall where you can connect multiple
26:23 of these firewalls together um they act
26:25 and function as one logical firewall
26:28 rather than having to um treat them
26:31 individually separately from an
26:33 operations point of view from a um CLI
26:35 management point of view and everything