AI Unbound, Your Data Center Your Way with Juniper Networks
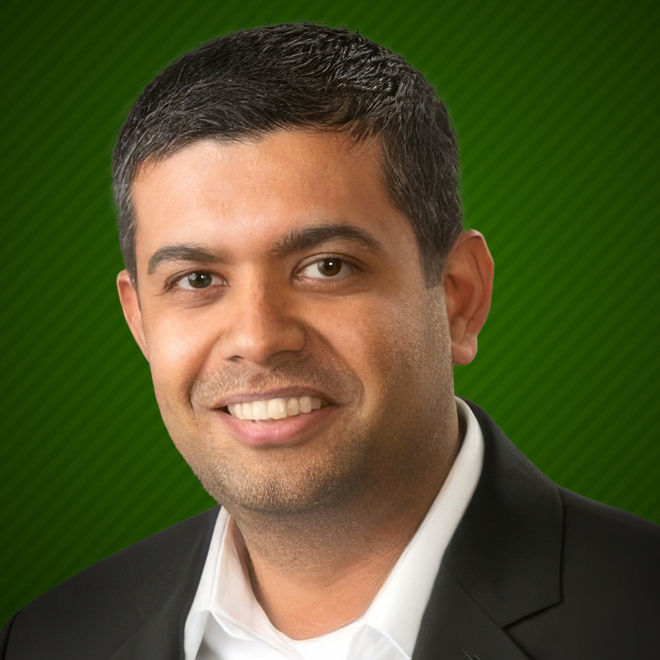

AI Unbound: Your Data Center Your Way
Praful Lalchandani, VP of Product, Data Center Platforms and AI Solutions at Juniper Networks, opened the presentation by highlighting the rapid growth of the AI data center space and its unique challenges. He noted that Juniper Networks, with its 25 years of experience in networking and security, is uniquely positioned to address these challenges and help customers meet the demands of AI. Juniper is experiencing maximum momentum in the data center space, with revenues exceeding $1 billion in networking alone in 2024. The presentation then dove into the increasing distribution of AI workloads across hyperscalers, Neo Cloud providers, private clouds, and the edge, emphasizing Juniper's comprehensive portfolio of solutions spanning data center fabrics, interconnectivity, and security.
Lalchandani focused on the critical role of networking in the AI lifecycle, particularly for training and inference. High bandwidth, low latency, and congestion-free networking are essential to optimizing job completion time for training and throughput and minimizing latency for inferencing. The discussion highlighted Juniper's innovations in this space, including developing AI load balancing capabilities such as Dynamic Load Balancing, Global Load Balancing, and RDMA-aware load balancing. Juniper was the first vendor in the industry to ship a 64-port 800-gig switch, showcasing Juniper's commitment to providing the bandwidth needed for AI workloads and achieving a leading 800-gig market share.
The presentation also emphasizes AI clusters' operational complexity and security challenges. Juniper's Apstra solution offers lifecycle management, from design to deployment to assurance, providing end-to-end congestion visibility and automated remediation recommendations. Security is paramount, and Juniper advocates a defense-in-depth approach with its SRX portfolio, protecting the edge, east-west security within the fabric, encrypted data center interconnects, and security for public cloud applications. The presentation concluded by addressing the dilemma customers face between open, best-of-breed technologies and proprietary, tightly coupled ecosystems, and that Juniper offers validated designs with AI labs to show customers they don't have to make a trade-off and can get the best of both worlds.
Presented by Praful Lalchandani, VP of Product, Data Center Platforms and AI Solutions. Recorded live in Santa Clara, California, on April 23, 2025, as part of AI Infrastructure Field Day.
You’ll learn
Emerging trends in the AI infrastructure space
The latest innovations from Juniper
Who is this for?
Host
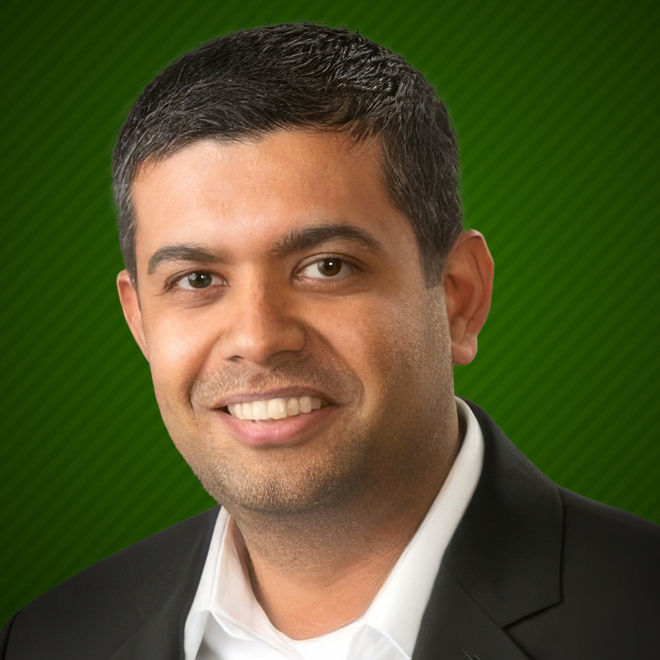
Experience More
Transcript
Intro
0:00 my name is Proful Landani and I lead product management at Juniper for our data center business unit so the
0:05 delegates in the room and for everybody else who's tuning in uh welcome to Juniper and thank you for being here we
0:11 do appreciate the valuable amount of time that you spend with us today now if you've been in the
Who is Juniper
0:17 networking domain for a while I would suspect you know a little bit about Juniper but those of you tuning in and
0:23 wondering who the hell is Juniper about this is a onelide primer on our history right so we've been around for 25 years
0:31 uh we have deep roots in networking and security a global customer base and we
0:37 serve enterprise cloud and service provider verticles customer segments we
0:43 have products and solutions that power networking and security in multiple places in the network starting with
0:49 wired and wireless in campus and branch van routing number two and number three which is the focus of today's discussion
0:56 is we have networking and security solutions for data center as well in fact data center is where Juniper is
1:03 seeing the maximum momentum these days these in the last few years with our revenues in data center crossing $1
1:09 billion in networking revenues alone that's not even including security in 2024 and we have more than 10,000 happy
1:17 customers who have deployed Juniper in the data center some of those logos in fact just a fraction of those logos are
1:23 on the screen uh in front of you who are some public references that we have but today uh the focus of today's
AI is increasingly distributed
1:30 session we're going to dive into the realm of AI data centers and from our
1:35 vantage point in the industry as an infrastructure provider for AI clusters we see one very clear trend that AI is
1:44 increasingly distributed let's explain how why so right so if you're an
1:49 enterprise you're going to have some of your applications that you're going to host with your favorite hyperscaler
1:55 but in the new world of AI there's a breed of GPU as a service providers and
2:00 AI as a service providers that are coming out there with niche offerings and we call them neocloud providers so
2:06 your application your AI workload may be hosted with your favorite hyperscaler but very likely is also hosted in some
2:13 other cloud provider like some of these neo cloud providers now if you're an enterprise for various business reasons
2:19 whether it is cost whether it is data privacy reasons or whether it is regulatory reasons you may also decide
2:25 that some of these applications you want to host them in your own private cloud or onrem and then there is also edge AI
2:32 because for some inferencing applications latency matters and you want to be as close to as possible to
2:38 the end user for those inferencing use cases now at Juniper we believe that in
2:44 order to support this distributed architecture we have we are uniquely positioned with a comprehensive
2:50 portfolio that spans data center fabrics it spans data center interconnect to connect all of these various clouds
2:56 together and security natively built in so wherever your AI is happening whether
3:02 it is in the cloud whether it's in private cloud or at the edge Juniper is right there to provide solutions for you
What makes data centers exciting
3:10 now this is what really makes us excited about data centers in general because this new wave of AI if I take an example
3:18 of a generalpurpose server right for general purpose computing reasons even today two ports of 25 gig for a total of
3:26 50 gig bandwidth per server is considered more than sufficient somebody may be going to 100 gig but
3:31 predominantly 50 gig per server you look at an Nvidia node even the last generation of hopper series with eight
3:37 GPUs you're looking at 400 gig connectivity per GPU you look at
3:42 multiply that by 8 GPUs front-end networking storage networking that's a total of 4 terabs of terabits per second
3:49 of networking per server that's 40x sorry 80x more than what you need for a
3:54 typical general purpose server right so this is what really makes us exciting because AI is lifting all boards and
4:01 networking is no exception to the rule over here and that doesn't stop in fact it
AI is lifting all boards
4:07 goes on fire in the next few years this is a slide straight from Nvidia's GTC uh Jensen's presentation and what Jensen
4:14 showed is that in 2025 when the Blackwell 300 series of GPUs comes out
4:19 is going to be powered with 800 gig of networking with the CX8 nicks and doesn't slow down there if you fast
4:25 forward another you know 12 to 18 months in 2026 2027 when the Reuben GPUs come
4:30 out they're going to need 1.6 terabs per second of networking per GPU from 400
4:37 gig today to 1.6 6 terabytes per second in just the next few
Why networking is important
4:43 years and so now I want to kind of impress upon why networking is important
4:48 in your entire AI life cycle and no rocket science in this slide so far over
4:53 here i think people understand the life cycle of an AI model but just to baseline it I'll list a little bit right so you start with what you call as
4:59 foundational model training this is the purview of large cloud providers or large enterprises who have resources of
5:04 thousands and thousands of GPUs to train a model from scratch but most enterprises are essentially going to be
5:10 doing fine-tuning where you take a generalized model which has been trained on a generalized data set and fine-tune
5:16 it for your with your proprietary data so that it can be customized for your own specific use case and then the
5:22 rubber hits the road in the case of inferencing where you take that trained model and deploy it in a distributed way
5:29 across many many AI clusters so that it can serve end users now we know that training was always a multiGPU problem
5:36 but what I want to impress upon today is that with the rise of things like reasoning models and agentic AI uh even
5:42 inferencing is becoming a multi-GPU communication problem uh while networking is important
5:48 for all of them what the the metrics tend to change between training and uh
5:54 inferencing in training the most important metric is job completion time how much time did it take to train my
5:59 model and with inferencing the metrics are slightly different you're looking at throughput uh which is my I have an AI
6:05 factory that is doing inferencing how many tokens per second or what's my throughput that I can generate for all
6:10 of my users and the second metric is because there's a realtime user sitting behind that LLM waiting for a response
6:17 the latency or the time to first lo token is a important metric for inferencing as well and like I said
6:23 networking plays a critical role in maximizing all of these metrics and we'll you know today we'll show you
6:29 how now diving Moving a little bit into training uh you know the training
Training cluster
6:36 cluster the green box in the middle think of it as a cluster of GPUs typically has three different networks
6:42 involved there's a front-end network there's the backend storage network but the important one that we want to focus
6:47 on today is that backend GPU training network that provides GPU toGPU connectivity across that cluster and
6:54 this is where you want to provide high-speed low latency congestion-free networking because any congestion in
7:01 these in this infrastructure can lead to traffic drops and anything that slows
7:06 down GPUs due to retransmission of packets or any other reason is really criminal in a in the case of AI
7:11 infrastructure because these GPUs are expensive you're spending millions and millions of dollars for these GPUs uh
7:18 for your AI infrastructure and having them sit idle is not something that you want so the networking plays a critical
7:23 role in improving the job completion time for training jobs
Inferencing
7:28 you look at inferencing like I said it's is no different you start with the left hand side the most simplest case is
7:34 single node inferencing right single client request comes in a GPU response sometimes it may even be a CPU right but
7:41 as I said with the rise of new types of models for example reasoning models where there's an internal dialogue
7:47 happening in the model before even the first token is sent out to the user there's a lot more east west traffic
7:53 happening as the model tries to reason its output before the first token or the first response goes out to the user uh
8:01 again there the you need that becomes a multiGPU problem the same thing with agentic AI frameworks so what we are
8:06 seeing is that increasingly uh inferencing is becoming a multi-GPU problem as well and requires a need for
8:12 a back-end inferencing network for east west communication and la lastly we have rag or retrieval augmented generation
8:20 where idea behind retrieval augmented generation is that you're supplementing an LLM's output to give it to enable it
8:27 to give more precise responses by giving by supplementing proprietary data that
8:32 it can use to to generate a response uh again in the case of rag you're basically looking at an environment
8:38 where you need to deliver very low latency because again there's the end user sitting at the end of the response
8:44 and you need to have you know those shared storage nodes that can be accessed at very very high speed so
8:49 networking again is plays a very important role over there now you know stepping one step
Challenges
8:57 above uh you know what are the challenges that anyone who's deploying an AI cluster faces i hopefully by now I
9:04 have stressed upon the fact that performance is important because GPUs are a critical asset but these AI
9:10 clusters are increasingly more operationally complex to manage as well think about it i told you that it was 4
9:16 terabs per second of networking per server that's so many more optics that can fail so many more links that can
9:22 bounce so many more BGP sessions that can flap and on top of that you have the need in AI clusters to have realtime
9:29 visibility of congestion events and the ability to manage and remediate those congestion events as well so
9:35 operationally way more complex than typical data center networks security was always important in an AI cluster
9:42 now in the world where your proprietary data is being used to train these models you face new rest new threat vectors
9:48 around model excfiltration data excfiltration etc and we're going to spend some time on that and then finally
9:53 in the in the world of AI clusters what we are seeing is that customers are facing this new dilemma should I pick
9:59 open best of breed technologies based on standard Ethernet or am I locked into
10:05 proprietary technologies just because they offer uh you know a closed ecosystem or a tightly coupled ecosystem
10:10 and we're going to spend some little bit time on that aspect as well how much are you seeing the shift these days from
10:16 scale up mega clusters to more scale out uh more efficient ones that only use
10:21 resources as needed is that something that that you all are seeing and you're planning for or do you already have that
10:27 so we are in the scale out domain right now and uh the scale up domain today
10:33 with Nvidia is a pretty locked in and we switched architecture but as AMD GPUs become more relevant that scale
10:40 up scale up opportunity opens up to other vendors as well so today we are mostly scale out but we are also looking
10:47 at scale up with non- Nvidia GPUs so it seems to be opposite you have the dense
10:52 models that people started with and the that are vertically but you've started out smaller with the more efficient LLMs
10:58 so I mean look until NVL72 came out uh most of the hopper class of GPUs was
11:06 sold as discrete at DGX and NGX systems right it's only with the blackwell where the NVL72 is coming out right so most of
11:12 our customer deployments even there's a customer who's deployed us for 200,000 GPUs this was all scale out it was not
11:19 deployed with scaleup scaleup is in fact is emerging recently since NVL72 became more uh popular starting in 2024 GTC
11:28 And do you have at some point a slide and maybe I missed it earlier that shows the whole topology of a of a AI network
11:34 yeah we're going to spend some time on that later okay great thank you
Performance
11:40 so uh starting with performance uh no shame in saying the first thing that we did was to throw bandwidth at the
11:46 problem right and we were the first vendor in the industry to ship a 64 port 800 gig switch we were the first OEM
11:52 vendor in the industry to ship a 64 port 800 gig switch that can uh in a two
11:57 compact form factor that can actually have two ports of 400 gig connectivity to the hopper class of GPUs and is
12:03 futurep proofed for the Blackwell class of GPUs as well so you can actually build a pretty large size cluster 8K
12:09 GPUs cluster in a three-stage design with just the QFX 5240 in a leaf and spine roll but if you want larger we did
12:16 not really stop there we also introduce a PTX 10,0008 chassis that has 288 ports
12:22 of 800 gig so you can build clusters again in a three-stage design going up to 32,000 uh
12:28 GPUs and now because the PTX is also a deep buffer system like I said there are
12:34 multiple AI clouds they need to be interconnected together using data center interconnect this deep buffer
12:39 platform is a is a superior choice even for your data data center interconnect use cases in fact we were the first
12:45 vendor in the industry to have a deployment of 800 gig using coherent optics for ZR lens so we were first with
12:53 800 gig on the fixed switches we were first with 800 gig on on the PTX with the debuffer switches both for data
12:58 center fabric as well as data center interconnect uh use cases now um now we were obviously busted to
Market Share
13:07 the market like I said with 800 gig and that's hopefully a testament to our engineering innovation in inity but
13:14 doesn't hurt that we got some market recognition out there as well this is something that c you know nobody expects
13:20 to see but as reported by 650 group just a few months ago in the year 2024
13:26 Juniper had a leading 800 gig market share the reason for that is we had the products first to market uh but
13:32 obviously this AI way was right picking up right at that time so it was a good product market fit but we had a market
13:38 share that was larger than Nvidia larger than Arista larger than Cisco in this data right here are we looking at any
13:44 specific verticals or regions or would that be a slide talking about global market share this is global market share
13:50 but data center alone it's not outside of data center it's just focused on data center
Dynamic Load Balancing
13:59 now like I said first thing we did was throw bandwidth at the problem but we all know that standard Ethernet was not
14:05 designed to be lossless uh it is not standard Ethernet without any
14:11 advancements advancement that we're going to talk about today is not suitable for AI workloads and the reason
14:16 for that is that Ethernet switches make a random hashing decision on which path
14:21 a flow should take so if you look at a picture on the top right two two flows two 400 gig flows are coming in from the
14:27 leaf from GPUs hitting the leaf and even if I have built up enough capacity between my leaf and spine it's a
14:34 possible that that Ethernet switch makes a decision that both those flows go to the same path to the spine and that link
14:40 is going to get congested so you have a situation where some links are underutilized others are overutilized
14:46 and need and you start hitting congestion and failures out there similar situation happens on the picture
14:51 on the on the top left as well so we started looking at this problem
14:56 you know way back in 2022 2023 and the first thing that we did was introduced a capability called dynamic load balancing
15:03 what dynamic load balancing does is that it makes a path forwarding decision not
15:09 just based on what available paths that routing tells me but based on the quality of those links in real time is
15:15 making a real-time decision on the quality of those links and making a forwarding decision you'll see in the
15:20 next session that that gave us tremendous improvement in performance over standard Ethernet but again we did
15:26 not want to stop there uh we introduced this feature in 2024 called global load balancing the idea behind global load
15:32 balancing is that it's like a Google maps for the network when I'm driving I see the congestion in front of me but I
15:39 only Google maps can tell me the end to end path quality so I make a decision now to turn left versus right the same
15:46 way global load balancing what it does it gives this leaf device that it needs to make a path decision end toend
15:53 quality information of the entire path all the way to the destination leaf and using that information it can make more
15:59 educated decisions to avoid uh to avoid uh the both the local link
16:05 as well as the remote congested link but did we stop there no and today what we are announcing is something called RDMA
16:13 aware load balancing this is a capability that enables a switch to see RDMA
16:21 subflows be aware of RDMA subflows and map them to specific paths through the
16:27 fabric it is gives us the highest performance as you'll see shortly it
16:32 gives you the lowest variability in performance so if you run that AI job 10 times you're going to get the same
16:38 performance each and every time dlb and GLB still give you some some amount of variability around a mean and it
16:45 requires a least amount of tuning to optimize the performance of your infrastructure
16:51 so today we are announcing AI load balancing uh which is these umbrella term that we are using for all of these
16:57 load balancing capabilities that deliver a 50% or greater than 50% improvement
17:03 over standard Ethernet now at this point if you are thinking that's you know you
17:08 made me curious but did not satisfy my curiosity completely i need to learn more I need to know more uh this is what
17:14 I was designed to do uh Ali Vikram Singh who's joining me or joining the next session is actually going to cover these
17:20 in a lot more detail so stay tuned for uh his session but I want to put a little bit plug over here um and this is
17:26 an important one you will hear our competitors who have proprietary
17:32 networking technologies for AI clusters constantly say things like this proprietary technology is 60% better
17:38 than standard Ethernet 70% better than standard Ethernet what they're comparing against is unoptimized Ethernet that was
17:45 not designed for AI clusters you know with the capabilities and the improvements that we are bringing to the
17:51 table we feel very very confident that customers deploying these clusters will get the performance and like comparable
17:57 to any proprietary technology out there including Infinipad so in this case what does open mean as far as proprietary
What Does Open Mean
18:04 does this mean that people have so anyone has access to the protocols is it
18:10 is it it's based on BGP it's based on Ethernet i mean all and know it can connect to an AMD GPU it can connect to
18:16 Cerebra Samonova it's not it's agnostic to the GPU you know it's not locked in
18:21 yeah industry standard industry standard yeah i have a question about Bitcon um
18:28 uh on the previous slide you said no tuning i would expect that uh that you
18:34 need to do some tuning definitely with RDMA if you're going to go all the way into the stack of the operating system
18:41 the servers as such tuning on day zero like uh you know you don't need to tune
18:46 uh like you it's not like you have to tune constantly like if I'm running a different model model A versus model B I
18:52 don't need to tune the network for that DLB and GB do require some amount of tuning there's some timeouts that you
18:58 have to configure appropriately to get the maximum performance uh But you know this technique will not and I think
19:04 Vikram session will make make that a whole lot more clearer okay thanks so and some of the things that you're
Packet Spray
19:10 talking about are are these part of the ultraethernet consortium work these so
19:15 uh so part some of them are uh some of them will become standardized as part of the UEC uh so for example our switches
19:22 already support packet spraying right what what is UEC standardizing the standardizing the ability of switches to
19:27 packet spray and the ability of the nicks to be able to to handle those out of order packets that's something we already support today okay right because
19:34 I knew that was part of it when you were talking about the the load balancing i just wasn't sure if this was tied into
19:41 to what's actually going to be standardized or not yeah some of these things so honestly from a from a UC
19:46 perspective the standardizing on packet spring when it comes to congestion avoidance right and that's something we
19:52 already support in our platforms
Power
19:58 now uh we can't really have an AI data center discussion without actually talking about power and uh if any of you
20:05 attended Jensen's uh keynote this year in GTC he eloquently stated the problem
20:10 statement especially around optics right he said a 100,000 GPU cluster has 400,000 optics associated with it you
20:18 know if we think that those optics consume 12 watts of power depending on whether what the reach is uh that's
20:25 total of close to 5 megawatt of power that is consumed just by optics alone
20:30 right and what Jensen said that you know he's introducing technologies uh like CPO that will be available in 2026 we
20:37 are working on CPO as well but what I want to say the operative word is that we have technologies today in something
20:44 called linear pluggable optics that take some of the DSP functionality away from the regular transceivers into the switch
20:51 the tomahawk 5 switch itself on the chip itself so that we can actually deliver that reduction in power consumption like
20:58 67% in the case of VR optics again this is available for our customers to take advantage of even today while we are
21:04 working on other technologies like CPO aim for
21:09 2026 now I did mention operational complexity as the one of the bigger
21:15 challenges in AI clusters And uh we have a whole session talking about Abstra and how how it is the
21:22 simple antidote to that complexity but just a little bit of an introduction on what Abstra is abstra is our day zero to
21:28 day end full life cycle management uh solution for fabric management and assures so right from design to deploy
21:35 to day to assurance it can do all of those things for regular data centers now obviously in the last year year and
21:41 a half uh we have been spending time enhancing the capabilities of abstract
21:47 to be ready for AI clusters and we'll see a lot of that today but I just want to point out two capabilities that I've
21:52 you know close to my heart because in talking to our customers one of the things that we realized was congestion
21:58 management was the hardest part of operating an AI cluster so we have delivered some capabilities that provide
22:04 not just switch visibility but switch to nick visibility end toend visibility from all the way from the switches and
22:10 the nicks to give you that view of where is the congestion in my network in my in a in a singular view but again we did
22:17 not stop there seeing congestion hotspot is one part of the problem operators
22:22 could sometimes spend days in remediating those congestion hotspots
22:27 and what we do with Abstra is this intelligent mechanism that is constantly monitoring for congestion and making
22:33 recommendations to operators that here's the hot spot this is a recommendation to tune the network or change the
22:39 congestion handling profiles so that you can al remediate that congestion as you
22:44 switch from one model to the next
Security
22:51 so the third pillar of our solution is around security and you know again Kedar
22:56 will join us later to talk about the security portfolio but like I said security was always important in any
23:02 data center in this new world of AI where your proprietary data is being
23:07 used to train a model you want to make sure that you are protected against those new threat vectors around model
23:13 excfiltration around data excfiltration etc now let me be very clear over here that when your proprietary IP is at risk
23:22 this is not just a technical issue this is you know a hit to your business value if your entire model gets excfiltrated
23:28 out and that's what your and that model was trained on your proprietary IP uh more more of that to come but what I
Cases from 2024
23:35 want to point out over here is that this is not just theoretical risks these things are happening in the industry as
23:41 we speak and I just want to touch upon a couple of cases just from 2024 in an example on the top in early 2024 there
23:48 was a case where hugging face models were infected with malware you know data scientists or users who downloaded that
23:54 malware of that model essentially had that model model running some you know
24:00 some suspicious code that opened a back door to a bad actor in the cloud and from there you know that bad actor can
24:06 now do anything nefarious with your models your data etc the second one actually blows my mind completely is
24:13 because you know this is a case where an AI as a service provider was uh had a vulnerability where a tenant in that in
24:21 that in in that infrastructure could be a bad actor and they run a malicious model once that malicious model is run
24:29 that model now laterally moves across to other tenants in that infrastructure so it's not just you who are you know maybe
24:35 did something wrong uh maybe you know maybe by intention or or not but now
24:41 you're affecting every other customer that was residing in that AI as a service or GPU as a service uh
24:47 infrastructure so I think that was pretty crazy uh so that's why at Juniper we recommend a defense in depth approach
24:55 you protect at the edge with our secure with our SRX portfolio you do not want that threat to enter your infrastructure
25:01 but you know sometimes even with the best efficacy in the industry day zero threats do penetrate and what you want
25:07 to do is that then to provide that east west security within your fabric itself so you assume that you know you have
25:12 some day zero vulnerability some day zero threats you know did enter your infrastructure you want to prevent that
25:19 lateral movement of those threats across your infrastructure with our both our QFX multi-tenency capabilities that
25:24 provide isolation for tenants in the with the QFX as well as our SRX portfolio then you have data center
25:31 interconnect you want to encrypt every communication between your between your
25:36 clouds to prevent from snooping style of attacks so we have max IPSec and uh
25:41 encryption capabilities available on both our SRX as well as our MX series of data center internet portfolio and then
25:48 finally if your applications are distributed and are running on public cloud then the SRX portfolio and more
25:53 specifically the virtualized SRX or the containerized SRX provides the same level of security for your public cloud
26:00 applications as you would see uh for your uh private cloud uh infrastructure and Kedar will spend a
26:06 whole session you know talking a little bit more in depth about this
Open vs Proprietary
26:13 so I said I'll touch upon the topic of open versus proprietary a little bit more and u and what we see is that you
26:21 know in every engagement I we have with our customers they're facing this dilemma right you know Ethernet had
26:26 become the open de facto stat standard for pretty much every place in the network whether it was campus whether it
26:31 was van whether it was data center and now comes this new world of AI and now they have to they're dealing with this
26:38 new dilemma should I go with best of breed technologies But then I have the have the challenge of kind of start
26:43 having to stitch it together and making sure it performs and works well or I go to this single vendor out there that
26:48 provides a GPU also provides a network and I have this allure that going with
26:53 this tightly coupled s you know ecosystem is the only thing that you know gives me that performance so I have
26:58 to make this trade-off between lower cost with a best of breed versus proprietary solutions that are more
27:04 expensive but have that allure of of performance so we really decided to take this challenge head on and the way we
27:12 did this was built an AI lab where we bought in all of these best of breed
27:18 components right we spent a lot of money honestly uh but we brought in all of these best of breed components a GPUs
27:24 from Nvidia and AMD storage from our partners like Vea and Vast nicks from
27:30 Broadcom AMD's Pensando sorry Polar Nick as well as Nvidia's connectex 7 six and
27:36 seven nicks and We brought them together stitched it together into an AI cluster
27:41 rigorously rigorously tested it for the for few months uh against you know real world training and inferencing workloads
27:47 and we're constantly doing that that kind of exercise never really stops as technology keeps evolving and what we
27:53 produce were these validated designs like the Juniper validated designs so if you do a Google search even now for
27:59 Juniper AI validated designs what you'll hit are two assets one is a validated
28:04 design that is anchored on AMD's GPUs another validated design that is anchored on Nvidia GPUs so what we are
28:11 what we what we are hoping to offer our customers is something very simple that they do not have to make a trade-off
28:17 anymore between best of breed flexibility and performance on the other hand they can get the best of both
28:23 worlds so I'm a little confused about Okay so you're doing a validated design based upon this stack here of Kimberly
28:29 Bates with HR group and I'm not a network person so just really not
28:35 um are you're doing a validated design using those that criteria as opposed to
28:42 what as opposed to uh as opposed to Nvidia
28:48 saying that hey if you buy my GPU you have to buy Infiniband or my buy my proprietary networking technology so in
28:54 Apple stack it's like an Apple because some of those things are also in the stacks that is in with so so what you're
29:00 basically saying it's Nvidia's um networking and Nvidia's GPUs which you
29:05 have Nvidia so it's basically you've taken Nvidia's networking out put yours in built that stack out have a validated
29:12 design so I've got confidence in a ship is are you seeing Well that's fine no
29:18 that's enough i'll get exactly that yeah uh and in the case of Nvidia we've
29:23 actually followed their super pod design right the only thing that we've replaced we even use slurm for orchestration
29:30 everything is the same we use we only thing we replaced is infin with Ethernet that that's pretty much the only thing
29:35 that we changed over there so with as validated designs you're saying hey we've tested all these you can mix and
29:41 match do you have reference architectures that say this is exactly how you can connect these and yeah and
29:46 sizing guides and all sort absolutely that that validated design actually is our reference guide so has all the best
29:52 practices down to which knob to configure how to tune your DLB dynamic load balancing everything right is is
29:58 all out there uh and not only that we've actually codified that into blueprints
30:03 in Abstra so if you don't want to you're not a CLI junkie and you want Abstra to deploy that all of those best practices
30:09 that we have learned and deploy them in one click reliably repeatably Abstra can do that as well abstra can deploy that
30:16 entire reference design so what part of Broadcom did you use what what's Broadcom element in that the Broadcom
30:21 obviously the the the switch is powered based on Broadcom's uh switching silicon that's what I figured okay but Broadcom
30:27 also has nicks they have the Thor 2 400 gig nick was that in there as well yes yeah okay mhm
Gartner Magic Quadrant
30:37 okay so um you know here at Juniper we are a engineering company and we do
30:43 enjoy solving some of the most pressing needs for our customers but once in a while it feels nice to get recognized
30:50 and this is something that was uh recently announced by Gartner just three or four weeks ago gartner reintroduced
30:57 the Gartner magic quadrant for data center in 2025 after a 5-year gap and we
31:03 were squarely in that leaders quadrant along with the other giants in that space right so the Gartner magic
31:09 quadrant being in the leaders quadrant I think speaks for itself so I won't linger on this slide uh anymore uh but
31:15 it is powerful what I do enjoy uh and I like even more is that there is a
31:21 companion report to the Gartner magic quadrant called the critical capabilities report and here is where
31:26 Gartner goes into details of a vendor's capabilities to assess their capabilities against a specific use case
31:33 and we were number one in enterprise data center buildouts like I was talking about those 50 gig servers for
31:39 enterprise data center buildouts we were number one uh out there ahead of Arista
31:45 ahead of you know the other you know typical vendors you would expect to be out there and u uh this is a testament
31:52 mostly to our fabric management capabilities with Abstra that you're going to see in a few
31:57 minutes and the topic of today's discussion was AI data centers uh I did say we have a leading market share but
32:04 Gartner recognized that as well uh we are only number two to Nvidia right
32:09 Nvidia is a behemoth out there that forces you know their customers to bundle in the whole solution and look at
32:16 the scoring like we were only 02 behind Nvidia for AI networking right so again
32:23 great testament to a great validation of our technology and the momentum that we
32:28 are seeing with AI data centers out there with our networking and security solutions
32:36 And it's not just analysts who are uh you know raving about us uh we have
32:42 customers as well uh this is just a small fraction of the customers who are actually public references and all of
32:48 these whether you're looking at some GPU as a service providers like digital ocean vulture ion stream xai who's
32:54 building this massive cluster in uh you know called colossus in me in in Memphis or some other uh enterprises out there
33:01 like sambonova cerebras wyoming PayPal uh they all have trusted uh juniper to
33:06 build their AI cluster whether it is for storage networking backend GPU networking or front-end networking so um
33:12 again that was my final slide on uh the traction that we're getting uh with customers and I'll take any few few
33:18 questions before turning it over to
33:26 Vicram going back to your slide where you showed the the uh hybrid AI cloud
33:32 how are you defining that compared to multicloud are you seeing more of multi cloud environments so I think of hybrid
33:38 I think of onrem and on the cloud and then multicloud of course being you've got multiple clouds within that not
33:45 onrem stuff yeah so so we are seeing so I think hybrid cloud maybe have been loosely
33:51 used term it is multicloud as well uh we are definitely seeing a lot of repatriation of workloads happening due
33:57 to the AI wave all right because you know you you need the models are being
34:03 trained on your proprietary data right so whether so we are seeing that applications moving closer to the data
34:08 rather than data kind of moving to the applications in public cloud so we are seeing a lot of private cloud buildouts happening uh with uh the know AI wave uh
34:17 and yes it is actually you could say it's a it's multiloud in a in a sense that some applications are running in private cloud some applications are
34:23 running in in public cloud in that sense yeah so data gravity and latency and data gravity kind of pulling
34:29 applications towards private cloud yeah I would assume security too for particular industries absolutely like privacy data privacy security reasons as
34:36 well thanks another question uh Um until now
34:43 what I heard most about Juniper was Mist how does it fit in this big picture here
34:49 mhm so Mist started out as our um you know portfolio for managing campus and
34:57 branch right data in data center we always so had abstra for managing and
35:02 operating uh data centers right but over the last few years we have uh integrated
35:08 some of these technologies together so Mist is basically a leading AI ops engine like for you know today we're
35:14 talking about networking for AI but that's more of AI for networking right and abstra started out as an on-prem
35:20 instance management instance but now abstra also has a cloud presence and that cloud presence is powered by mist's
35:27 AI ops engine to provide more predictive capabilities uh for data center as well
35:32 so simple answer mist was for campus abstra was for data center but going
35:37 forwards we are seeing more and more of their integration where we're bringing this KI ops capabilities from Mist into
35:43 Abstra in a cloud instance as well okay thanks
35:48 so Jim Sprincy CDC um your customers you know you've laid out your three or four
35:54 pillars is it the security aspect that most people are interested in i mean I'm
35:59 just interested if you kind of did a pie chart yeah so I would say that when it comes to AI clusters I talked about four
36:06 pillars uh you know let's say performance operational complexity security and open versus proprietary if
36:11 you don't have performance the rest of the pillars don't are not nobody's going to consider you right so you need to
36:17 first show that you know I'm neutralizing every other proprietary technology out there with an Ethernet
36:23 lowcost solution lower cost solution that delivers the performance so I would say everybody's in there for the
36:28 performance otherwise you know the remaining pillars do not even matter but yes security becomes a a differentiator
36:33 for us our operational capabilities with abstra and our security do become the differentiator once we have neutralized
36:39 that performance is is good okay