Responsible & Trustworthy AI
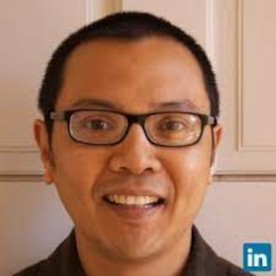
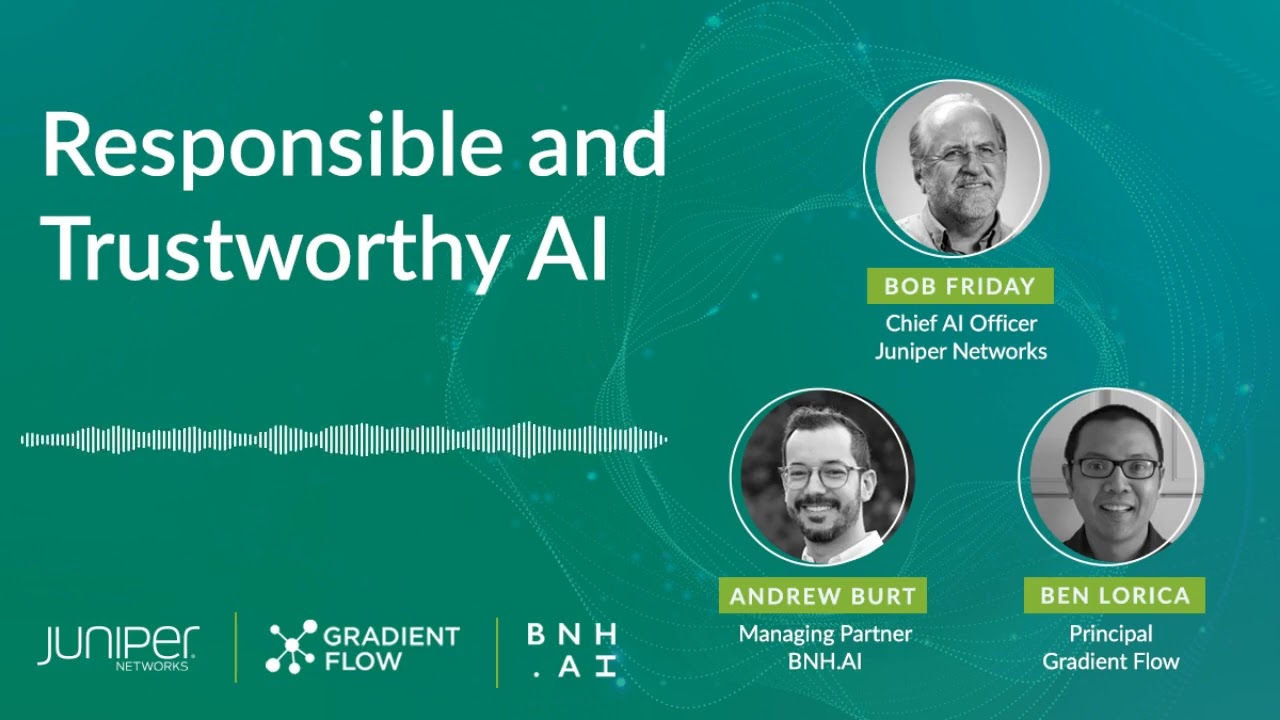
What makes artificial intelligence trustworthy?
Listen as this panel of AI experts explores what it means to build trust into AI, as well as share predictions on the state of AI regulation and governance. Also see Bob Friday’s blog post: Growth vs. Regulation – How Should You Approach AI?
1:58 What makes AI trustworthy? What does it mean to build trust into AI?
6:40 Predictions on AI regulations
9:30 AI risk mitigation techniques and best practices
18:30 Rise of the Chief AI officer
20:45 Introduction of the NIST AI Risk Management Framework
25:25 New job roles and responsibilities as related to responsible AI
You’ll learn
AI risk-mitigation techniques and best practices
Introduction of the NIST AI Risk Management Framework
New job roles and responsibilities as related to responsible AI
Who is this for?
Host
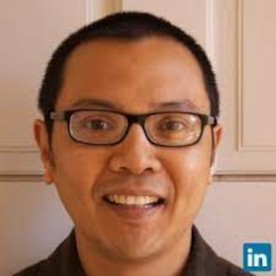
Guest speakers
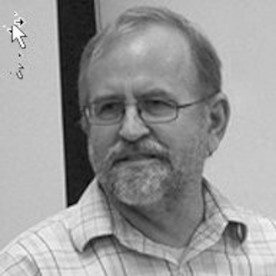
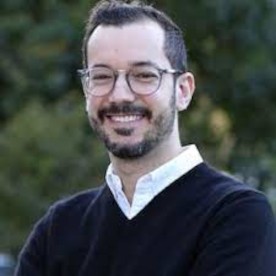
Transcript
0:00 hello i'm ben lorica i am the principal at gradient flow
0:05 and the host of the data exchange podcast ai is the backbone of today's busiest
0:12 products and technologies whether it's self-driving cars
0:17 conversational assistance and even our home appliances like our
0:23 vacuum cleaners as these applications grow in both the consumer and enterprise spaces
0:31 teams of reliability trust and safety in these algorithms are
0:38 rightfully taking front and center that's why i'm excited to share a
0:44 recording of a recent conversation i had on twitter spaces with bob friday
0:50 chief ai officer of juniper networks and andrew burt managing partner of bnh dot ai if you
0:59 have any questions afterwards feel free to reach out directly to myself or any
1:04 of our panelists on twitter or linkedin without further ado here's the conversation sponsored by
1:12 juniper networks today we have two pioneers in the space
1:18 who i've known for a long time uh first we have uh bob friday he is one of
1:25 the first uh chief ai officers uh that i know that
1:30 have actually have that title which tells you how important ai is to
1:36 juniper networks and secondly we have uh andrew burke who is managing partner of
1:43 the first law firm focused specifically on a.i and machine learning risks
1:49 so welcome uh andrew and bob
1:54 thank you ben it's great to be here so let's start with a baseline what is your definition of trust
2:01 specifically uh in your mind what makes a.i trustworthy so
2:06 there are lots of different ways of thinking about trust um and my shorthand definition of
2:12 trust is basically that it's a probability relationship trust is really just the probability of something
2:19 doing what you expect it to do um and so when we talk about ai the question is
2:25 when we talk about ai trust the question is how likely is this ai system to do what it is i expected and i'll just add
2:32 that one of the reasons why trust is so important and critical with ai is because ai is so complicated it's so
2:38 complex that it can be quite difficult for users um or even operators of ai systems
2:47 to understand that probability to to have high confidence in the system doing what it is it's
2:53 supposed to do so andrew uh as a follow-up uh so
2:59 what does it mean for data teams and data scientists today who are trying to
3:05 build trustworthy ai so what are some of the key considerations and and
3:12 steps or even tools and i ask you this because i know you talk to a lot of teams not just data teams but even uh
3:20 chief legal counsels who are uh very concerned with these issues yeah it's a great question i'll say that
3:26 like all all day every day i spend my time at b h helping organizations uh
3:33 who are really struggling with with what it means to make their ai trustworthy um
3:39 and and and responsible with a focus on ai liabilities and so i'll just
3:44 i guess kind of make two points um and stay really really practical which is i'll rephrase your question to say like
3:50 what is it that teams can actually do to make their ai trustworthy um uh and and
3:57 more than that what is the highest impact um uh things that they can do and so
4:02 i'll name two things the first is appeal and override um making sure that
4:08 users of ai systems can flag when things go wrong and operators of ai systems
4:17 can override any decisions that might be creating potential harms or any incidents that's
4:24 the first thing we see a lot of times in practice ai systems being deployed without appeal and override
4:30 functionality um one second um
4:35 uh without appeal and override functionality and so what that really means is that just
4:41 deploying a system with no way of getting feedback from its users um uh is
4:46 basically a big no-no and leads to all sorts of risk um and the second thing teams can do just quite quickly is
4:52 standardized documentation documentation across um data science teams is extremely
4:57 fragmented and that creates a huge huge amount of risk and so something as simple as just standardizing how systems
5:04 are documented um can go a really long way and so uh bob quick review so in
5:10 your ma from your perspective how would you uh define trustworthy ai
5:15 number one and number two uh what are some uh initial steps that you and your team are doing
5:22 yeah thanks ben you know i think from my perspective you know there's kind of three dimensions to this ai trust
5:27 discussion right now one is what i call consumer safety this is kind of the what we're seeing happen in europe around the
5:33 ce mark right trying to make sure these eight new ai products are actually do what they claim to do or safe so there's kind
5:40 of what i call the regulations part of trust coming on there's also kind of what i call the esg environmental
5:46 social governance trust right this is basically where our investors want to trust for doing the right thing with ai
5:52 and then finally there's kind of what i call the user trust right these are people who are actually using our ai products they want to make sure we have
5:59 trust built into the product we want to be able to trust these ai assistants on par with humans
6:04 and into your question you know really what are we doing here at juniper with this you know our first step here is really coming out with ai guidelines so
6:11 you know if you go google juniper ai guidelines principles you'll see that juniper actually has pulls now around
6:17 ai's so uh andrew um and bob brought up the notion of
6:24 regulations and i know this is something uh you have to uh pay close attention to
6:29 since you do talk to the chief legal counsels of many of these uh large companies
6:36 there are so many regulations in this area so for our listeners what regulations
6:42 need to be kept in mind both in the short and long term so that's a great question honestly
6:49 there are so many and frankly there's so many that are overlooked um i'll just go through them so there's municipal level
6:55 state level and federal level just in the u.s and that's ignoring all the big important stuff that's happening in the
7:01 u the eu at the municipal level there's a really interesting law that comes into effect
7:06 in january in new york city and basically any ai system being used in an employment
7:12 context in new york city needs to be um uh
7:18 audited and that's that's frankly quite a big deal what it means for for these systems to be audited who can audit them
7:24 how those audits work and so that's that's the municipal level at the state level there are already laws on the books in virginia colorado
7:32 um even california um that mandate all sorts of different transparency and
7:38 requirement uh transparency and impact assessment requirements on ai systems so those are laws that have already been
7:45 passed and are beginning to be enforced um and then at the federal level um there are a host of different um uh
7:52 proposed regulations the big one is the american data privacy and protection act
7:57 but just to step back basically all of these laws at a high level require different kinds of impact assessments or
8:04 third-party audits of ai systems and so even though there's a lot of variety in
8:09 what the laws mandate um at a high level um they're starting to require basically the same things
8:16 that's a great point so bob uh over to you uh as far as regulations are there
8:22 any specific ones that you're paying attention to i mean i think right now but look what's happening in europe is
8:29 you know it looks like europe is starting to come out with regulations around safety right where they're
8:34 starting to identify specifically ai applications you know self-driving cars
8:39 ai applications that are doing with hr bias you know and they're starting to identify these apps and they're going to
8:45 start running them through you know frameworks like ce right you know if you can't ensure that these ai apps don't do
8:52 harm just as you would with any other product safety so in europe i think i'm seeing much more of a centralized
8:58 regulatory approach you know in the u.s it looks like it's much more distributed across the different frameworks right
9:03 fda each different group inside the u.s is starting to come up with their own ai regulations their own regulations around
9:10 ai so let me broaden this a little bit and for
9:15 this question you don't have to answer specifically about trust it could be on
9:20 any uh specific ai risk that you are paying attention to or that you see
9:26 other teams paying attention to so are there any uh ai risk mitigation techniques
9:34 that you want to highlight for our audience that uh you think are especially best practices so andrew you
9:40 had mentioned documentation so anything else that comes to mind i mean it's it's funny because the big
9:47 thing what i find myself and other folks at b h
9:52 um telling our clients over and over again is just documentation and appeal and override those two things can go so
10:00 far um but because i already said both of those i'll just also add model monitoring we
10:06 see very very frequently data science teams um uh basically
10:13 emphasizing two things time to deployment and accuracy metrics um and
10:19 so they feel like if they can get a high accuracy model and they can get that model deployed quickly like they're good
10:24 and they've done their job and very frequently model monitoring kind of falls out of the picture and so
10:31 ensuring that model monitoring efforts um uh are are um
10:36 are stood up can be a really really effective risk mitigation and how about you bob
10:42 are there any uh specific tools or sets of processes
10:47 or checklists that uh either you're implementing today or plan to implement
10:53 or are inspired by yeah i mean i think on the engineering side right you know for the actual engineers who are
10:59 building and deploying these models you know if you dig into that you'll find out they have tools like ml flow and
11:05 these are basically tools that when you train a model you have to look for data drift right you know once the model's
11:11 trained you want to make sure the data that you trained it on and the data that's coming in real time there's not a big delta you know so there's tools like
11:17 that to make sure your models are kept up to date you know and trained on the latest data that represents the data
11:23 coming in i think insider juniper inside of companies you know beyond just building product there's kind of the new
11:29 risk we have this in the company right you know whether it's an hr department buying ai tools or a supply chain you
11:36 know and i think right now for almost all large companies right we all have training modules we go through ethics
11:42 training awareness training you know what's right wrong governance so i think we're going to start to see
11:47 that being baked into our company training programs right where we go through ethics training we'll start to
11:53 see aib making people more aware of what they're doing and when they're buying ai
11:58 tools what they should be aware of and can i just add to that sorry ben tell me if i'm i'm messing with the flow
12:05 but one thing i'll also add which i neglected is just having clear policies in place what bob said is i think
12:11 absolutely critical just like monitoring for data drift but one thing we see all the time um is that um the regularity or
12:18 the formality of when things are monitored and checked can be a highly highly variable so just having
12:25 some clear policy in place and whether it's ongoing active monitoring for drift
12:31 or just some type of periodic um testing to see what might have changed between
12:36 training and deployment so just having clear policies written down having everyone understand what those are so
12:43 they don't deviate too much across teams and time that can also be a really highly effective risk mitigate
12:50 so andrew what is the nature of your conversations around these topics these days i know
12:55 that in the past when we've spoken you you've expressed uh
13:01 the opinion that you felt that data teams were still a little resistant
13:06 to having some of these checklists or or extra
13:13 tools or extra requirements yeah i mean to some extent um uh we're
13:19 kind of crashing the ai party um and i'll say that that you know one one thing um that i see all the time and is just
13:26 true is just there is so much hype around ai and frankly there's so many organizations that talk about ai that
13:32 aren't actually seriously deploying it um and so kind of cutting through the hype and getting to the actual
13:39 kind of use cases um because we're a law firm people don't tend to call lawyers
13:44 unless they're worried about getting in trouble and so the conversations that that i have um and that folks you know
13:51 other folks at the law firm have are focused on different types of liabilities how might this existing law
13:56 impact an organization how might a future law impact an organization um there are a number of different um uh ai
14:04 incidents you can go the um there's actually stood up i think last year the ai incident database which just
14:10 centralizes all the bad you know um uh things that ai systems can do um and so typically that's where
14:17 our conversations go um uh and i'll say that that um
14:22 that's quite different i think from a lot of the broader discussions about ethical ai
14:28 and trustworthy ai which frankly can be had without real fear of consequences
14:34 and so for that reason there's a wonderful paper that a non-profit algorithm watch published
14:40 just focused on the number of organizations releasing ai ethics guidelines um and so many different organizations
14:47 have um but then one level deeper you really want to get into basically
14:52 consequences when things go wrong and that's where you know as a law firm that that's where uh our our conversations
14:59 tend to focus so bob uh in your conversations with your fellow cxos
15:06 what's your sense of uh of uh what people are doing out there yeah i think
15:12 in my in my conversations right now i think people are trying to figure out the difference between you know we've been building these complex systems for
15:18 years right you know so what is the real difference between these ai systems we're building and what we built in the
15:24 past you know so the conversation is really around where do you draw the line between ai and automation you know
15:31 optical character recognition is that really ia or is that just an automated tool we use for translating text into
15:38 you know letters into text and i think this really gets down to what is the definition of ai
15:45 you know for me right now you know when i think the word ai you know and why i'm a chief ai officer here you know
15:51 is when you're doing ai it's ultimately doing automation but you're basically trying to automate something that would
15:56 typically take some human cognitive reasoning right and so i think that's a
16:02 subtle difference between what we've done in the past around automation you know and what we're doing right now
16:07 around ai right you know when we're driving cars that's doing something on par with a human and the behavior
16:12 changes right so i think that's the other definition around ai is when behaviors change that's hard like when you buy a
16:19 tool and it doesn't change you know how it's going to behave with real ai the behavior is changing over time
16:25 so let me uh actually make make it a little more concrete for you bob so one class of models for example these
16:32 large language models right so that many people use i mean i use it all the
16:37 time these days uh as part of some uh larger nlp pipeline so typically
16:44 people will take some pre-trained model maybe fine-tune it uh and if if the fine tuning works out
16:53 then they can deploy right so what what do these large models that maybe
16:58 someone else pre-framed what are the implications of that for you and your team well i think as you mentioned right
17:04 now so you take a large you know hugging face or something that google or facebook trained you know the companies or the startups
17:11 i'm talking with right now that basically they're putting layers of making sure you know you don't get inappropriate
17:17 hate messages or hate language coming out of these brief trained models right you know and this gets back to where you
17:22 have to constantly watch the behavior of these ai systems right because they're changing over time you know where these models these big
17:29 models get retrained or you update them right so i think your your analogy here is correct right you know these big
17:35 models we're all using we're building on top of more complexity right
17:40 we're all building on top of big complex models that we don't fully control sometimes you know honestly sometimes i
17:47 think about the pythons ecosystem right so
17:52 can you every time you install a python library it just installs so many dependencies
17:58 that yeah you know we we depend on the ecosystem that has so many dependencies the dependency
18:05 graph of these libraries can be scary at times and i think that's what you think about
18:10 open source that's a whole different topic of complexity right you know if you look at where the whole you know
18:15 industry is headed right now we're all using you know 20 30 40 different open source packages to build something you know and
18:22 you're right the trail dependency goes down a pretty deep rabbit hole and that's a great point by the way bob
18:29 i'm intrigued by your title chief ai officer so what prompted juniper
18:35 to stand up uh chief ai role yeah you know if you look at the specific case here right you know
18:41 juniper acquired my last company missed in 19. you know and our first mission here was really to
18:46 extend cloud ai across the enterprise portfolio that was aps wireless switches and
18:52 really we're trying to extend that across the broader company so there's really two missions one is on the product side you know how do we extend
18:59 cloud ai across the juniper portfolio and then there's this other dimension around governance you know how do you
19:06 govern ai going forward in the future right and this is our discussions around esg you know what does it mean to have
19:11 investors and customers start to ask us what is your policy on ai you know what are your principles and guidance
19:17 so i think there's two dimensions one is on the product side and one's on the governance side across the company
19:23 previously when we when we spoke uh one of the areas you folks were quite
19:29 engagement were in nlp and chatbots for example right so so they take chatbots or conversational ai
19:36 are there any specific uh things you are doing now uh to make that
19:42 to make those systems uh more responsible and trustworthy yeah and i would say the conversational interface
19:48 you know here you know we're working on specifically really to build something that can troubleshoot and manage and
19:54 operate networks on par with you know it don't you know humanity domain experts you know and really that conversational
20:00 interface is the key to trust right you know and we talked about you know the user trust right how do you get
20:06 a user to really trust an ai assistant you know and my analogy is always it's on par with hiring a new person right if
20:12 you have someone join your team you know that person has to earn your trust you have to be able to interact with this person you have to be able to
20:19 interact with this is ai assistant and that's where the conversational interface comes in right the days of
20:25 dashboards i think are giving way to the days of natural language you want to interact with your ai assistants like
20:30 you would with any other new member on your team and you want that ai assistance to really earn your
20:36 trust and you want you want that ai assistant to actually get better with time the more time you spend with it you
20:41 know you want to trust that that ai assistant's actually learning lenny bring andrew burke back into
20:48 conversation i know that you and your firm were instrumental in this new
20:53 uh risk ai risk framework from the national institute of standards and
21:00 technology nist which is in uh standards body here in the us
21:08 and uh quite influential if you look at for example uh the cyber security space
21:14 a lot of people look to nest guidelines in that space so recently
21:19 you were part of a team that helped put together the nest framework for ai risk
21:24 so at a high level what is it and what has been the feedback
21:31 yes so so just for for background so it's actually quite exciting we're the first law firm in the history of nist um
21:36 to be given a research grant and and what we have been doing is um conducting research um and providing counsel in
21:42 support of this big effort it's called the air mf the risk management framework
21:48 that effort is a congressionally mandated effort and basically a task nist with setting some standards for how
21:55 to manage risk um for ai very similar to what nist did about a decade or so ago on setting
22:01 standards for how to manage cyber security risk and so um basically where things stand is
22:07 um there was the first draft of the rmf that was published a few months ago the final um draft is going to be um uh uh
22:16 ready by january and so we're actively you know supporting this effort um and for folks who are not familiar with
22:23 what's kind of actually inside there are really four components um uh to the rmf
22:28 um there are three m's and a g so map measure manage and then govern and so basically govern means having policies
22:36 and procedures across the whole organization and then map measuring and manage um are three different functions
22:43 that are applied to specific ai systems um and so i will leave it at that i'm happy to to go deeper ben if you want me
22:50 to go into the weeds but otherwise that's the high level so so what has been the feedback since the release
22:56 um that's a good question you know i um uh there there are people better than me who who know the the the in-depth
23:02 feedback i think the feedback has been positive um generally i think one of the
23:08 things the reasons why i'm so excited about the project um uh um but is is its
23:14 potential for impact and frankly i think the wild west nature of right now you know ai risk management efforts i think
23:21 every organization approaches it pretty differently and again it's it's a little bit like the
23:26 wild west and so um trying to put some cohesive strategy on top of that and cohesive
23:33 framework i do think there's a little bit of friction and so i think some organizations have have been basically
23:40 saying you know this is not enough this is not stringent enough and i think other organizations have been saying you
23:45 know this is way too detailed and granular and so i think my impression of the feedback um is that
23:53 um it basically suggests that right now the rmf is kind of the happy medium
23:58 but for folks who are interested um nist is actively seeking feedback and you can actually give them feedback directly
24:06 and you can look i think if you just google ai rmf you'll see all the existing documents on the
24:11 website and there are different methods for getting in touch with the official nist team
24:16 by the way a few validation points i have seen the nest framework cited in papers even uh by uh leading ai teams
24:24 so that's uh that that's a good sign right yeah i mean i think again one of the
24:30 reasons i mean honestly there are a bunch of reasons why we're so excited and frankly like you know honored to be
24:36 uh um actively supporting it um but i think it really of all the different efforts at setting like a unified
24:42 standard i think it's the one that's most likely to succeed um and again just
24:48 like looking at what happened with cyber security i think to some extent ai is today where cyber security was you know
24:54 10 years ago or 15 years ago everyone knew that risk was an issue a lot of incidents were
25:00 happening kind of behind the curtains they weren't being widely reported and it took a bunch of
25:07 different things to make infosec more standardized and so having a
25:12 national level standard it's not mandatory it's not regulation but having a national standard that organizations
25:19 can compare them to um themselves too i think is going to go a long way so it's awesome to see you
25:24 know research folks already citing it in preparation for this twitter spaces uh i i dug around and mined some data and i
25:31 looked at uh for example job postings and uh around this around the topics
25:38 that we're talking uh about today discussing today responsible ai and uh it's clear from uh
25:45 just uh looking at job postings that uh top of mind for companies in this area
25:51 andrew are security and privacy so i i mean responsible ai in general
25:56 and then fairness and then trust so really uh it's that basic can we make
26:03 the ai system secure and make sure it doesn't violate privacy that's still at
26:08 the top top line yeah i mean we so we so so we have a
26:13 whole list of different types of liabilities that ai can create um but at a high level we really just talk about
26:20 four and just like in the order um of what clients seem to be you know concerned about its fairness and privacy
26:27 are far far and away the top concerns um and far far and away the top incidents where
26:34 we get called in there's some type of fairness incident there's some type of privacy incident um the other two are security and
26:40 transparency um and i think over time security and transparency of ai systems
26:46 are gonna like their profile is gonna increase as a concern um but in our experience you
26:53 know across our client base that's that's typically what we tend to see yeah yeah yeah it seems like uh i joke
26:59 with people in this space it seems like a lot of the concerns right now tend to be theoretical because they can't
27:06 they can't even name like a actual uh uh concrete incidence of model inversion or
27:15 model extraction it's all kind of uh papers and research projects right so yeah yeah i would say been an
27:22 interesting example where you know hey you know it's all about fairness whether it's ai or anything you know
27:27 there was a case where a city basically created an app for reporting potholes um
27:32 and what they found out was it turned out that you know rich people tend to have cell phones more than you know poor
27:37 people so what happened there turned to be unfairness right it turns out all the potholes that got reported were in the
27:43 good side of town you know so that's an example no matter what you do with fairness whether it's ai or anything you
27:49 always have to be on the lookout right you always have to make sure you haven't built some unfair component into the system now with ai it's a little bit
27:56 trickier because sometimes you're not aware of it it happens in the training session that you're not maybe fully aware of
28:02 so let's close by uh just speculating a little bit about how uh
28:08 uh this space will evolve so andrew uh since you talk with a lot of
28:13 uh data data and ai teams and chief legal counsels so are you seeing
28:20 kind of uh more focused roles so hiring more focused talent
28:26 around these topics so or is it still kind of something that
28:32 data teams and ml engineers have to do as part of their uh broader portfolio of
28:38 uh of tasks i'll give you i'll give you a real lawyer's answer which is it depends
28:44 um and in fact some of the data scientists i used to work work for uh worked with a long time ago got me a
28:50 sticker for my computer that just said it depends because that's you know typically what what lawyers say um and
28:55 so the reason why i'm saying that is because um uh a lot of what matters is
29:00 like the scale and size of the organization and then also how serious the organization is about ai if in
29:08 reality ai to that organization is mostly about marketing um then uh chances are less
29:14 that they're gonna have you devoted dedicated resources and teams focused on ai risk um but i think what we see you
29:22 know across our client base at b h um is we see increasing concern from legal
29:28 departments and also data science teams who want to do the right thing and also don't want to get in trouble
29:34 but increasing concern as their new and new laws on the books related to ai and so um i think it it kind of started in
29:42 the privacy offices which were which tend to house the the most technically sophisticated lawyers um
29:49 and what we are seeing i'd say over the last year or so is instead of you know the chief privacy
29:54 officer or someone in that office being responsible for managing ai risk we are
29:59 starting to see specific um uh roles um uh uh reporting
30:05 directly general counsel um that are focused you know specifically on ai so
30:10 something like associate or senior counsel for ai is a is a title that
30:16 we're seeing more and more and their role is to interact with um and oversee the risk side um of of a
30:24 lot of what the data scientists are building and so how about you bob how are you approaching uh staffing for these topics
30:31 you know i would kind of echoing and andrew here is like we are also starting to see more privacy you know start with
30:37 gdpr right you know it started coming with a cloud privacy data
30:42 discussion you know so you also inside juniper and other large companies you're starting to see more people here
30:49 responsible for making sure data privacy is happening so that i agree with you know chief ai
30:55 officer right even in my own role here right now we're starting to see more and more companies put data privacy chief ai
31:01 officers in place to really help kind of look at the governance aspect of this uh with that uh thank you andrew and bob
31:10 for a spirited discussion very practical uh takeaways and ideas uh that you
31:16 shared with us today