Bob Friday Talks: Arijit Raychowdery (Georgia Tech) on AI in Higher Education
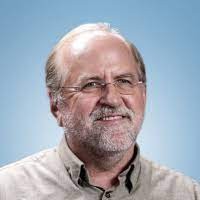
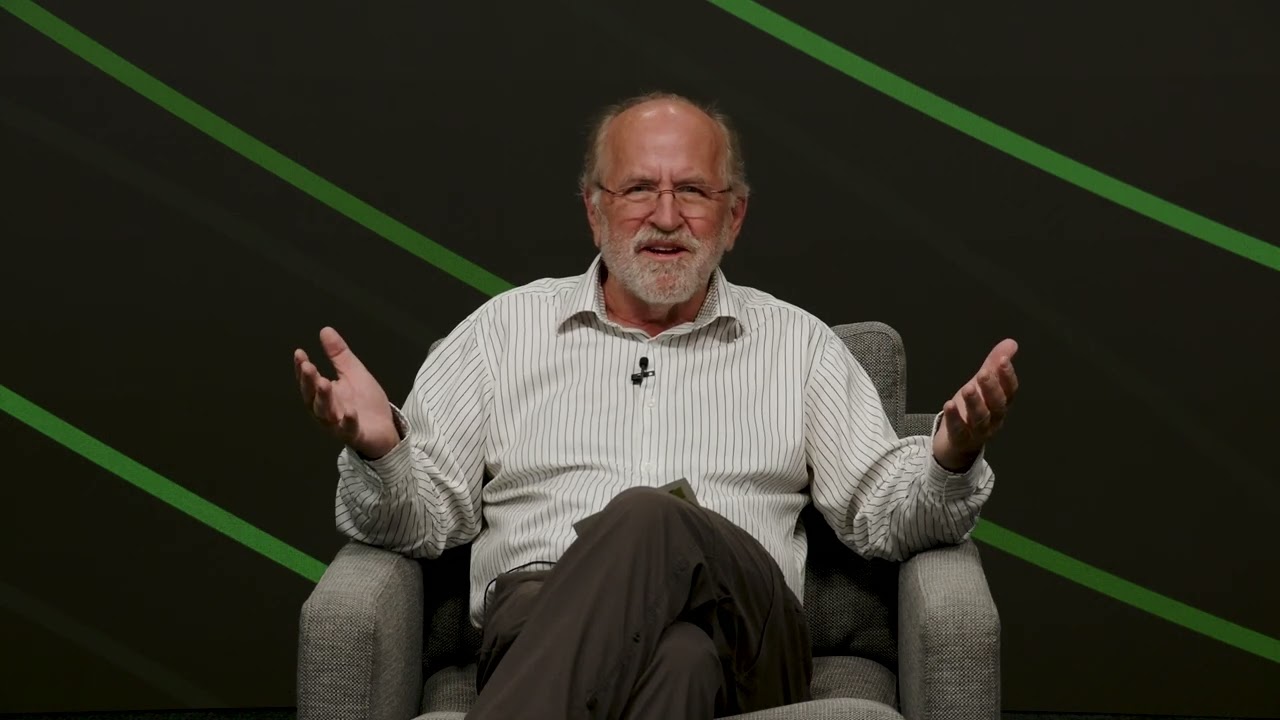
AI Integration in Higher Education at Georgia Tech
Arijit Raychowdery from Georgia Tech discusses how AI is being integrated into higher education curriculum and operational practices. Learn about the impact of AI on various professions and how students can leverage AI tools in their projects and studies.
You’ll learn
Insights on AI integration in higher education.
The impact of AI on various professions.
How students can use AI tools in their education.
Who is this for?
Host
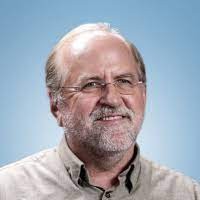
Guest speakers
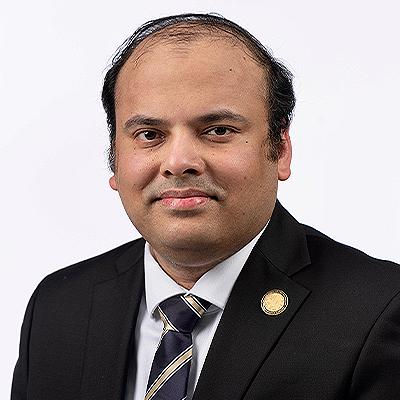
Experience More
Transcript
00;00;00;00 - 00;00;29;20
Unknown
Welcome to another episode of Bob Friday Talks. I am joined by Ray Chowdhary, the Steve Chaddick School Chair at Georgia Institute of Technology. Today we're going to discuss AI in higher ed, the integration of element, and how students practically use it in their day to day use today. Urjit. Thanks for joining us. Maybe we can start out a little bit by giving you a little bit of your background, you know, and what the research of AI looks like at Georgia Tech today.
00;00;29;22 - 00;00;52;11
Unknown
Sure. Thanks a lot for inviting me, Bob. It's great to be here. As you have mentioned, I am this currently serving as the Steve Chaddick Chair in, electrical and computer engineering here at Georgia Tech. I am a professor here, and I've been here for the last 11 years. This is my blog there. Having moved here from the industry, I used to work at Intel before that and before that, you know, for Texas Instruments for a couple of years.
00;00;52;13 - 00;01;21;13
Unknown
So I, I am an industry transplant, if you may. My research interests have always revolved around circuit and circuit design, and I've been, you know, in the industry and now in academia. I have mostly been working on digital circuits. And of course, a large part of my work has also been impacted by AI. So we are using AI as, as you know, in EDA tools, for example, for circuit design, as well as use doing circuit design and architectural exploration for for for AI workloads.
00;01;21;15 - 00;01;36;12
Unknown
So you asked me about, you know, the AI research in Georgia Tech. So Georgia Tech is, you know, we have the, the at the moment, we are the largest college of engineering in the country. So you can imagine that, there is a lot of work going on in Georgia Tech in the, in the, in the AI area.
00;01;36;14 - 00;02;00;05
Unknown
Starting all the way from you know, if you look at just electrical and computer engineering, we have a lot of people working on, new technologies for accelerating AI workloads. These are both on the server side as well as, you know, on the on the edge as well as somewhere in the middle. We have people working on, new transistor topologies, new transitional, integration methods, new packaging technologies, new memory technologies, new interconnects.
00;02;00;07 - 00;02;21;28
Unknown
And these involves, you know, material discovery, integration and so on, like, all throughout the spectrum. And then we have people working on circuits and architecture to accelerate many of these AI workloads, including language models and and vision models. And then we have people who are working purely on the theory of AI models and looking at what's there beyond deep learning, what's there beyond transformer models?
00;02;22;01 - 00;02;41;29
Unknown
How do you bring more explainability to AI models? So there is a large amount of work going on in the algorithm space in that, in that area. And that's where, you know, engineering intersects with, computer science, for example. And then on the applied side, just, you know, there is a lot of work going on on using AI for sustainability, for energy, for maintaining our grid.
00;02;42;01 - 00;03;01;14
Unknown
There is work going on in using AI for medical sciences, where we partner very closely with our, partner institute, Emory University, with each other. You know, they have a medical school. There is a huge focus in robotics and particularly for manufacturing and automation. So on the applied science, you know, of AI, you know, you can think about all possible disciplines that that's out there.
00;03;01;17 - 00;03;20;21
Unknown
Georgia Tech has some presence in any of them. So, overall I feel like, you know, we are we are very blessed to be in a place where there is such a vibrant research culture. And we work very closely with companies, with the government, with other entities. As we look at, you know, the entire spectrum of AI from all the way from devices to algorithms and applications.
00;03;20;23 - 00;03;41;11
Unknown
Yeah. So maybe, I mean, you're at the forefront of research on AI right now. We know when you look back, you know, what happened with the internet and computers and Moore's Law where we, you know, doubled the size of these transistor densities every 18 months. You know, when it comes to AI. Any thoughts on how fast this AI is growing?
00;03;41;12 - 00;04;05;21
Unknown
Or if we look at the size of these models, right. We're talking billions, if not trillions of weights. These models have gotten so large, you know, they're on par with your brain in terms of neurons. You know, any sense of how fast this is moving right now, you know, towards that singularity. So yeah. So I think the the let me let me break it down into two parts is how fast is it growing.
00;04;05;22 - 00;04;21;08
Unknown
Yeah. I mean, if you look at the model sizes, it has been you know, an exponential growth path in the last few years. And there is no I don't see any, any limit to, you know, this at the moment. You know, it seems like it's, it's, it's just an exponent at the moment. And you can see why, why that's the case.
00;04;21;08 - 00;04;38;07
Unknown
Right? As the models and the training data gets larger, the model sizes get larger. You are getting more and more out of this. AI models, you know, thinking about language models and how, how better they are. They are getting, you know, in actually interpreting and working with humans. So I that I that path is going to keep on continuing.
00;04;38;07 - 00;05;00;16
Unknown
But again, I think there is a there's a, there's a realization both in the industry and academia that again, that's an exponent. And all exponents eventually must die. Right. Like that's the prophecy of Moore's Law as well. So, so I think this exponential going to, you know, not be sustainable beyond the point there are energy crisis, you know, you will not be able to provide enough power to run these data centers or train these models.
00;05;00;18 - 00;05;19;12
Unknown
So there has to be this this exponent will slow down. But you know what's going to come next? I think there's a large part of research that's trying to address that. You know, what's going to happen beyond deep learning or how do we augment deep learning with other kinds of models so that you have more, you know, more, I would say more efficient scaling patterns for AI.
00;05;19;14 - 00;05;38;06
Unknown
Then I think the whole question of singularity, I think that's I think that's more of a philosophical question than a scientific question. And, I mean, we don't know, I think is it, you know, is AGI you know, really possible? I think maybe it is, maybe it is not. But at least at the moment I feel like, you know, we, we are trusting AI to do a lot more than it actually can.
00;05;38;09 - 00;05;54;18
Unknown
I think that's my worry. As opposed to, you know, we are, you know, the AI is going to take over and kill us all. I don't think we are. There will ever be there. But I'm I'm I'm, you know, from my perspective, I think we need to make AI systems more interoperable. They should be more, explainable.
00;05;54;18 - 00;06;12;29
Unknown
There should be more transparent. And that's how I think we will be able to, you know, broad trust what the situation with the AI, I mean, the humans and I need to work together in a trustworthy and and in a, in a and I secure kind of an environment. I think that's I feel like, you know, we are getting there with some of the recent advanced algorithmic advances in AI.
00;06;13;01 - 00;06;35;22
Unknown
You know, maybe, you know, from a research perspective, you know, if you look at, you know, what's holding back, I you think it's more about learning to build bigger, faster silicon and hardware to train these models. Or you think it's more about the research to be more focused on building more efficient transformer models, building newer models that are more efficiently can be trained.
00;06;35;24 - 00;06;57;12
Unknown
That's a great question. I think there are there is value in both, and I am not very sure the industry is the best place for, doing work on, on, let's say larger models, more data, more training. I don't think that's something that, that, that, you know, that, that academia is very good at. I think, if you look at know any of the large us and Hyperscaler companies, super scalers.
00;06;57;12 - 00;07;12;22
Unknown
Right. If you look at them, they have more and more data with more compute power and more and more resources to be able to do that kind of research. So I feel like the industry moving in that direction and in bringing us more complex models from, you know, GPT 4 to 5 to six and whatnot in one direction.
00;07;12;26 - 00;07;29;07
Unknown
There are different versions of lab and all that. So that I think is going to continue. And that's what the industry strengths are. And the more data more, more, you know, more I'd say the larger models and so on. On the academic side, I feel like more of the research needs to happen. On understanding what's going on at the under the hood.
00;07;29;07 - 00;07;45;08
Unknown
Right. You know, why does this in these models work? If we have some understanding of why the models work, we would also be able to understand when the models don't work. And that's I think, the the important part of this conversation. We also need to be a combination of things like bias. And we need to understand, you know, how there's training bias.
00;07;45;08 - 00;08;09;22
Unknown
There is data bias. How do we tackle some of those issues? We will also I think academia being the thought leader in generally in in a social construct, I'd say you'd also play an important role in understanding how, policies and regulations need to, you know, influence. Yeah, if at all. And then there is, you know, from a purely scientific discovery perspective, I think, you know, this whole idea of just doing deep learning is not enough.
00;08;09;22 - 00;08;36;11
Unknown
We need to augment that with, you know, with probabilistic models, with symbolic models, so we can create more holistic AI models. And, you know, there is a lot of existing data or existing understanding of how the physical world works. And, you know, our 2500 years of, you know, physics understanding as human society and understanding of the of the, of the of the workings of the world, that all need not be just data driven.
00;08;36;11 - 00;08;55;18
Unknown
We also need to do like, you know, symbolic reasoning. So I feel like the last 40 years of AI development, not just what happened in the last ten years, we should look at it more holistically. And that should be our driving force as well as academia has been said. Yeah, well, I always wonder now that these models are gains, you know, they're almost starting to gain the complexity of a brain.
00;08;55;21 - 00;09;21;06
Unknown
You know, is there any signs that the neuroscientists are going to start applying their their efforts to understanding how these models work? Do you see the, you know, the the comparison between the brain and these big, complex models starting to become a neuroscience type of problem? Absolutely. I you know that this is a great question. I actually I work with, with a group in MIT, I think they're essentially working on very exactly what you're saying.
00;09;21;09 - 00;09;40;15
Unknown
So they have neuroscientists who are working on primate brains and they're trying to understand, you know, let's say if, if a rhesus monkey is looking at a bunch of different images, what parts of the brain light up and they are trying to build deep neural network models to capture that information, and then using that deep neural network model back again in triggering some of the processes in the monkeys brain.
00;09;40;17 - 00;10;06;04
Unknown
So there is information that's going on in computational sciences that we are trying to bring back into neuroscience. And then, of course, neuroscience informs a lot of the, computational models that we are developing. Having said that, I think the abstraction levels of neuroscience that we are using for computation are at a very high level. I mean, of course, the, the, the the signaling at the neuroscience level is all chemical and that there are lots of processes that go on at a very low level that we really don't understand yet.
00;10;06;07 - 00;10;27;08
Unknown
But very recently I would say that, you know, one of my colleagues in Georgia Tech, he has been applying, machine learning models to understand, depression in humans. And by putting something like a pacemaker, like a sensor within the brain, they were able to actually understand, how to treat depression that is not typically treated by chemicals, by drugs.
00;10;27;10 - 00;10;56;25
Unknown
And they have had very good success in treating patients who have this kind of drug resistant depression by triggering right parts of the brain with electrical pulses. And the only reason that they were successful is because they have created, an obstructed view of the brain, of, of the, the dealing with using computational models. So I think there is a lot of great synergy between both directions, like neuroscience informing computational sciences and other way around as well, which I think is great for healthcare.
00;10;56;28 - 00;11;13;27
Unknown
Yeah. No, it's interesting how it all started, you know, trying to model the neural networks or model out the brain that were back, using them to help model the brain. But these neural sciences. So so maybe I'd like to change the topic a little bit. You know, I'm a Georgia Tech alumni. You know, I went it's been early 80s since I went to school there.
00;11;14;00 - 00;11;33;11
Unknown
Yeah, maybe give the audience a little bit of feel for how is I really changing the student experience at Georgia Tech, at higher ed universities, you know, are we embracing AI as friend foe? It kind of reminds me of the calculator in high school, you know, or professors embracing it as a tool to help them. Or is it basically, no.
00;11;33;11 - 00;11;49;15
Unknown
AI in the classroom. Yeah, yeah. Great question. And I think that's a great analogy as well. And the reason I'm saying this is a great analogy is because if you look, if your document is to look at any student today, no matter what campus, they are using calculators, right. So I think what has happened is, have the forgotten how to do additions and subtractions?
00;11;49;15 - 00;12;05;07
Unknown
Maybe not. I hope that they have not that still can do in addition and subtraction and multiplications and so on. But what they're also doing is they're using calculators as tools. I think that's well, and that's how I look at it. And that's why most of higher ed is looking at I have, least in Georgia Tech, it's another tool that we have.
00;12;05;07 - 00;12;24;25
Unknown
But so you need to be able to use it properly. So Georgia Tech has completely embraced AI in all possible ways. Starting at the very beginning, when students apply for, for, you know, to Georgia Tech, they are now you know, we not only tell them, but they can use AI for right, writing their college courses, but we actually encourage them to write their colleges, as with AI.
00;12;24;25 - 00;12;44;27
Unknown
So I think the idea is, you know, you use AI as a tool, understand that it has its limitations. But if you understand its limitations and you can, you know, provide the right prompts, for example, to a language model, you would be able to generate good text and it will be personalized, it'll be able to your liking. So some generic copy and paste from an AI chat box is not what we are looking for.
00;12;44;27 - 00;13;02;16
Unknown
What we are looking for is how do you use this better as a tool that will help you in your, you know, literally let's say you are writing some literary, you know, passage. You can help you if you are trying to understand some, mathematical or scientific principle. And, you know, there is information in a, in a trained model that can help you.
00;13;02;16 - 00;13;22;17
Unknown
I think we will do that. And the students are also, there are a couple of courses, for example, where professors are using, language models to create, study material for the students. So it becomes more personalized in the student is like as opposed to having a tutor, a human tutor, you now have an AI tutor. You ask a question to the city attorney, replies back, and you ask another question, and so on.
00;13;22;20 - 00;13;38;16
Unknown
So so I think it yeah, you know, overall, I think the intersection of AI and our curriculum and Georgia Tech have been, you know, from the very beginning, very close. And we have, you know, we have we continuously are using AI tools, to evolve our code and our curriculum and course materials. So it it's an ongoing process.
00;13;38;16 - 00;14;02;27
Unknown
But, you know, but we are all for it. And we are kind of completely, completely committed to making sure that our students use AI in their whatever, you know, career pursuits that they have. Yeah. So you if if you had a high school, you know, senior trying to get into college and their interest in AI, you know, what would be the advice nowadays for someone coming into university is the curriculum changing is basically computer science.
00;14;02;27 - 00;14;28;01
Unknown
The path to AI or the basics are to optimize the curriculum inside the higher ed, you know, to help to help a career in AI. Yeah, yeah. I think first of all, I think, you know your point about high school students. So just to give you some context that we feel like, you know, we need to engage with students when they're in high school and even in middle school so that they can they can be, well aware of what's going on in the in the world of engineering and sciences.
00;14;28;01 - 00;14;44;27
Unknown
And I, that will give that will give them enough, confidence that they can navigate this path, because I feel like there are a lot of students in high school who feel like they are. They have been, you know, they have been misinformed that the AI is going to come and take all their jobs and they don't have a, you know, career path anymore and so on.
00;14;44;27 - 00;15;03;15
Unknown
So these are all, you know, as we know, these are this is not the right information. Right. So we want to make sure that students early on, even in high schools, are aware of what the capabilities are of AI and what I cannot do and where, you know, human beings are going to be important and that the jobs are probably going to evolve and change, but it's not going to be, you know, eliminated by AI.
00;15;03;18 - 00;15;23;00
Unknown
So to that effect, we have programs. For example, we want, you know, where we engage with local high school students, and then we bring them on to campus over the summer for a couple of weeks, and we teach them AI. They are using, you know, Google Tools, Colab and whatnot. And they they essentially get exposed to, you know, AI tools and they go back to their schools and teach their fellow students and so on.
00;15;23;02 - 00;15;48;13
Unknown
So there is a lot of outreach and engagement with high school and middle schools in and around the city and the state of Georgia. And then I think from a the second part of the question, which was, you know, what should we tell our high school students? I think a large part of this would be computer and computer science and algorithms is one part of AI, which is, you know, if you really want to understand how AI works and you are the one to become the inventor of the next model, you have to look under the hood and understand how AI works.
00;15;48;13 - 00;16;08;17
Unknown
You can kind of, you know, do the math and all of that. On the other hand, you know, AI is going to impact a whole bunch of other jobs and professions as well. And for students who are not interested in that, who want to use the AI as a tool for their design project, or they want to become architects, or they want to become electrical engineers and want to use the AI for, you know, for essentially, from a data science perspective.
00;16;08;23 - 00;16;24;25
Unknown
So we need to be able to provide them all those opportunities and those infrastructure as well, so that they can use AI for whatever educational mission that they have. So, from from a school's perspective, from Georgia Tech perspective, we are trying to do both. We are trying to provide opportunities for students who want to innovate the next AI model.
00;16;24;27 - 00;16;40;14
Unknown
And, you know, they are more the computer science, the electrical engineer and kind of students. And then we also have students a large number of students who would want to use the AI in their in their individual pursuits. And we want to create a low barrier to entry for them as well. So that's that's going on as well.
00;16;40;16 - 00;17;01;09
Unknown
I think, RJ, I want to maybe go a little bit deeper on this, your research right now, because, you know, and listening to you sounds like you're really working on trying to make the infrastructure more efficient in training these models, which are really about linear algebra and matrix math, you know, maybe give the audience a little bit, Phil, how fast is that changing?
00;17;01;11 - 00;17;34;05
Unknown
How fast are we improving the compute infrastructure to actually train these models? Sure. Of course, you know, hardware infrastructure always is, much slower than than software and algorithms like the model sizes are increasing at alarming pace and the hardware infrastructure is not able to keep up with it. You know, the amount of memory that we need, the amount of, you know, compute that we need keeps on, you know, growing, having said that, I think a large part of my research is understanding how you can efficiently map some of these algorithms, particularly the linear algebra that you need for these algorithms on efficient hardware.
00;17;34;08 - 00;17;57;14
Unknown
A large part of the design of silicon, and compute is essentially in, is invested in memory, like, you know, if you have large models that need to be stored in memory and, and the question is, how do you move data from one part of the memory to the compute and back. And then the models are so large you cannot fit it in one compute unit, like not one GPU, not one CPU or one, you know, specialized accelerator that you may have.
00;17;57;16 - 00;18;21;16
Unknown
It needs to be distributed across many, many, many computers. So the question now is how do you move data? And is it, copper, is it, you know, is it going to be optical? What kind of interconnect do you need? So there there are various questions that you want to answer. And I think my group is primarily interested in understanding, how to use these new kinds of technologies to accelerate, I workloads of the future.
00;18;21;18 - 00;18;52;09
Unknown
And that means, you know, a lot of, you know, system level modeling and simulations and understanding that impact of technology on these kinds of workloads. And we also build a lot of prototypes. You know, we build the FPGAs, we build the, you know, GPU prototypes, as well as silicon prototypes to test out some of the ideas that we have and kind of innovate on what's the next big compute unit beyond, let's say, a GPU that you may have or a TPU that you may have, so that you can accelerate this work, this huge, you know, workload exponential that you are seeing.
00;18;52;09 - 00;19;11;03
Unknown
So so that's kind of in a nutshell, where my research group is kind of focused at the moment is, you know, here at Juniper, we call that networking for AI. You know, where these clusters, the GPU clusters are getting so large. As you said, it's important moving data between memory cycles, between devices and clusters and such. How important is it networking?
00;19;11;09 - 00;19;30;29
Unknown
I know juniper here. We're at, you know, 800 gigabits per second, right. You know, getting data from A to B is becoming a critical part of this. You know, do you see that going up to terabytes. How fast is is the networking limiting how fast we can train these things. Absolutely. Network is limiting I think. And if you look at some of this, memory extended in know, you know, hardware.
00;19;30;29 - 00;19;45;04
Unknown
And if you look at some of these new kinds of, protocols that we are using to send data over these links, as well as new physical layer links, right? So you're not, you know, going beyond copper now looking at optical. And the question always is, you know, with optical look the question is, you know, what's the cost?
00;19;45;12 - 00;20;00;24
Unknown
What what you know, what should be the length before you start going from copper to optical. There is work going on in it. Even introducing optical in the package, like, you know, taking it all the way close to the chip. So so I think, you know, there are different kinds of protocols. There are different kinds of physical layer mechanisms.
00;20;00;24 - 00;20;29;29
Unknown
And there is there are different kinds of ways that you can move and route data. So that is the big limiter at the moment. Data movement is the is the big limiter. Because if you look at, you know, some of these AI models, the number of compute cycles per byte, that's really low, which means that you cannot reuse a lot of the data even if you do batching and all that, and particularly with some of these language models, you need a lot more, memory per compute or lot less compute power per byte, which means you need to move data from the memory to the logic and from one, you know, one cluster to the
00;20;29;29 - 00;20;57;05
Unknown
other, from one rack to the other. So networking will and it still is and will continue to be a big, big player in how we charted our next, you know, ten years of AI workloads in hardware. So yeah. So I'm aware of what, you know, what you guys are working in, in juniper. But I think the entire community that's in the that's working on the networking infrastructure, that's as vital as, you know, as the companies that are building the Asics on the hardware or for the AI workloads.
00;20;57;05 - 00;21;12;18
Unknown
Yeah, it sounds like what you're thinking on the networking side that, you know, you think the photonics, the optics are actually going to get integrated into the chips, you know, is that the next big step in the networking effort to get this faster, get faster? Or where do you think the how how fast are we going to be able to move data here?
00;21;12;25 - 00;21;34;22
Unknown
We're at 800 gigabits per second. We're going to double that next year. Yeah, I think we will. I think we'll leave. It will eventually have to go beyond one terabyte per second. I think that's that's definitely the place where we want to wait. I think the other important piece is what's the pixel perfect. Right. So think that's that's another important factor I think with optics in the conversion as you know from electronic optics and back from, you know optics electronics.
00;21;34;22 - 00;21;53;28
Unknown
That's expensive. So we want to make sure that we use this conversion only when needed. So there is a lot of work going on in the package level where you want to kind of integrate optics directly on the back package. But that comes with an energy cost. And a large part of the research in academia is, geared towards how do you increase throughput but also decrease, you know, energy per bit.
00;21;54;01 - 00;22;11;18
Unknown
And that involves, you know, new kinds of material, a lot of compound semiconductor work is going on in that space. And also, how do you do these conversions? You know, this, this, you know, electro optic and optoelectronic converters. And how do you build better converter so that you can integrate them very close to the logic, but at the same time make them very efficient.
00;22;11;18 - 00;22;31;28
Unknown
So, yeah, this is kind of evolving. And I think there is, the jury is still out, you know, at what length, scales should we produce? Optics. And I think that that will will keep on working on this for the next few years for sure. Yeah, yeah. The other topic while we have you here, I was kind of interested in was around the how Georgia Tech and hybrids, because there's a lot of talk right now.
00;22;31;29 - 00;22;54;04
Unknown
Almost all the universities I work with right now are trying to come up with some AI strategy for internal operations. Yeah, maybe. Any words of wisdom for the other universities out there? You know, at Georgia Tech, you know, are you finding ways of using AI to make the university more operational efficient? Yeah. I mean, I don't know if these are what what is the wisdom, but, you know, I can share some of the things that we are thinking of.
00;22;54;04 - 00;23;16;16
Unknown
We are definitely interested in using AI and, and, you know, AI based tools as a means of, of, you know, forecasting data, using it more as a data science tool, like, you know, AI has helped us in creating dashboards, for example. But I can do very clear and easy visualization of operations of, you know, of, things like budget resources and so on and so forth.
00;23;16;16 - 00;23;41;01
Unknown
So that's one place where I've seen, you know, AI being useful. As you can imagine, Georgia Tech being such a large organization, many of the software infrastructure that we use are not, you know, not developed internally within Georgia Tech. We essentially, you know, license many of the software tools and, when we license tools, I mean, many of them are already getting I, you know, I use some of the intuition of AI, and we are adopting many of these tools.
00;23;41;04 - 00;24;10;11
Unknown
Internally, I think we are also thinking about, you know, using AI for, for things like, you know, making software processes more and better, or even for the training, like, you know, we have so many staff members that need to be trained on you know, on budget processes and procurement processes and so on. So there is some internal, internal, you know, discussions and initial work going on on whether we can train language models to, for example, to be very Georgia Tech specific and train people, in operations.
00;24;10;13 - 00;24;26;25
Unknown
So I think that's also going on in parallel. So all of these are in very nascent stage. And and again, you know, I, I expect that in the next few years we will start using this in our operations. You know, maybe as we wrap it up here for the audience, any last words of wisdom where you think this?
00;24;26;25 - 00;24;52;21
Unknown
I know this AI train is headed from a research perspective, you know, what do you think the next major step is going to be in the journey to AI? Yes, I think there has to be some sort of, I think the energy cost of AI, particularly this language models and all, are becoming, untenable. So I think that's where a lot of the investment needs to happen in making, and making an AI systems more efficient, more energy efficient.
00;24;52;24 - 00;25;11;20
Unknown
So, a part of this would be in the hardware, in designing data centers and making sure that, you know, we have the right kind of materials. Right. Kind of, you know, circuit architectures, right, kind of software stack to be able to make things more efficient energetically. And then I think, as a society, we also need to be cognizant of the energy cost of AI.
00;25;11;20 - 00;25;34;14
Unknown
I think we not we, you know, we we, we enjoy making tech smarter, but it comes with an energy cost. It comes with the sustainability cost. I think as a society we need to understand that as well. So I feel like, you know, if we really want to use the AI and which we do. And as a true companion, I think understanding the limitations of AI and where it is kind of, you know, consuming a lot of resources, we need to be cognizant of that.
00;25;34;14 - 00;25;56;26
Unknown
And a large part of the research, which I feel like would be driven in those directions, things making things more efficient and more, you know, sustainable as opposed to the current trend of exponential growth, which is great for for the very beginning, but it's not good for our long term sustainable. Okay. Well, I want to thank you for joining us today, and I want to thank the audience and look forward to seeing you on the next version of Black Friday Talks.
00;25;56;28 - 00;26;06;09
Unknown
Thank you so much, Bob. It was it was great talking to you. Thank you.