Bob Friday Talks: Ram Velega on AI Networking and Infrastructure
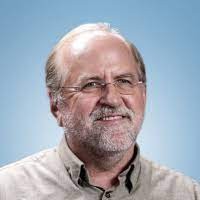
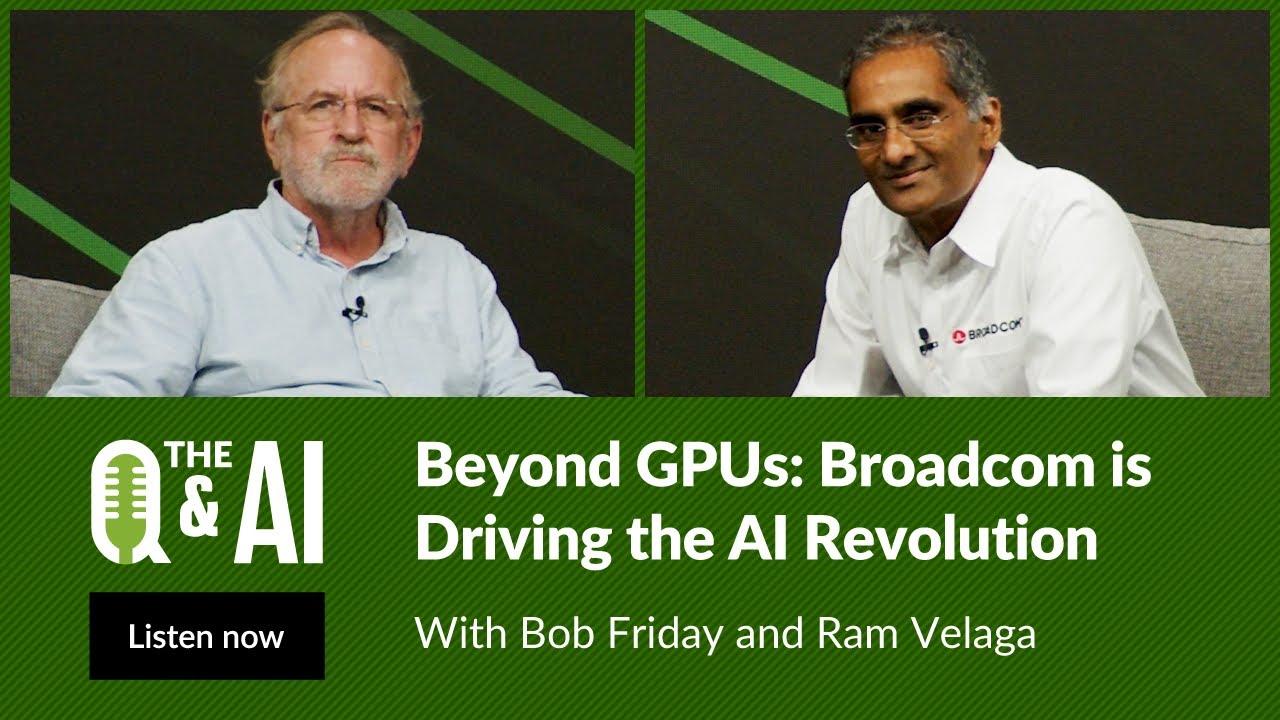
Bob Friday Talks: Ram Velega on AI Networking and Infrastructure
In this episode of Bob Friday Talks, Bob interviews Ram Velega, Senior Vice President and General Manager of the Core Switching Group at Broadcom. They discuss the network infrastructure provider’s role in the AI ecosystem, including how different elements of the infrastructure ecosystem support large AI deployments by offering high-speed connectivity solutions essential for AI clusters. They also touch on the impact of AI on various sectors, the importance of networking in AI, and the potential future of AI.
You’ll learn
General AI trends and their implications
Networking components crucial for AI
AI's influence on economic and sustainability factors
Who is this for?
Host
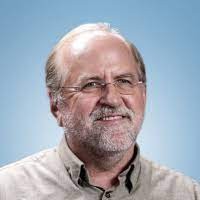
Guest speakers
Experience More
Transcript
1. Introduction and Guest Introduction
0:00 hello and welcome to another episode of
0:02 Bob Friday talks today I am joined by a
0:05 very special guest here for another
0:06 round after the aidc sees the moment
0:09 event Ron Vela senior vice president and
0:12 general manager of core switching group
0:14 at broadcom we're going to touch upon a
0:16 wide range of topics today such as
0:18 general AI Trends to networking for AI
0:21 and from there we're going to dive into
0:22 a conversation around ai's impact on
0:25 various sectors of the networking
0:26 industry such as economics and
0:28 stainability Ron welcome today you know
0:32 to start with maybe I was reading an
2. Broadcom’s Role in the AI Ecosystem
0:34 article about broadcom is actually a big
0:36 Ai and player for investors you know I
0:39 you think of Nvidia and gpus but you
0:41 really don't think of broadcom being a
0:42 big AI player so so maybe you can give
0:45 us a little background on where does bre
0:47 Brom fit into the AI
0:49 ecosystem hey good morning thanks for uh
0:52 having me here um uh you're right is
0:55 which is generally people don't think of
0:58 us as in you know uh AI player because
1:01 we don't have a GPU um broadcom doesn't
1:04 make a GPU broadcom doesn't necessarily
1:07 make you know storage so to say uh but
3. AI Trends and Networking for AI
1:10 however what broadcom does too is if you
1:14 look at the general AI
1:16 market today about maybe close to 70% of
1:20 the GPU consumption is the large clouds
1:24 the clouds I'm referring to Google meta
1:28 Amazon you know Microsoft Alibaba bite
1:32 dance tensent and so on so forth and
1:35 when these large Cloud customers are
1:38 deploying their you know
1:41 gpus there is a tendency for them to
1:43 also develop their own inhouse custom
1:47 you know
1:48 accelerators uh if you look at Google um
1:51 you've heard about their TPU yeah is one
1:53 of their in-house accelerators and
1:55 similarly meta has their in-house
1:57 accelerators and you probably heard
1:59 about other companies is building their
2:00 own in-house accelerators that's where
2:02 broadcom comes in is when the customers
2:05 have their own IP and they want to build
2:06 their accelerators bcom provides a whole
2:09 Suite of Ip you know whether it is CIS
2:11 or being able to actually provide a
2:13 platform where we can integrate their
2:15 highspeed hpms and so on so forth and
2:18 take their design and take it to
2:20 production so that's one way we play in
2:23 the uh accelerator Market but more
2:26 importantly if you think about it the AI
2:30 is all about very large scale
2:33 distributed computing and when you're
4. AI’s Impact on Various Sectors
2:35 doing a large scale distributed
2:36 computing you have to be able to connect
2:38 all these accelerators together so
2:41 Network becomes a very important element
2:44 in this AI play and broadcom does a very
2:49 you know comprehensive job in networking
2:51 our switching and routing chips our
2:54 Optics capabilities our Nick
2:56 capabilities all of our PCI switching
2:59 capabilities all all of these kind of go
3:00 into you know helping build these large
5. Use Cases for AI Accelerators
3:03 AI clusters okay so it's not all about
3:05 gpus so you're seeing a lot of
3:07 Enterprise and businesses building
3:09 special accelerators for different use
3:11 cases out there you know be kind of
3:13 interesting what what use cases are you
3:15 seeing that they're requiring
3:17 accelerators being out in the market
3:19 right now yeah so so today when you look
3:22 at these large you know uh 20 30 40 plus
3:27 billion dollars of cap exes by these
3:29 companies that are happening you know
3:31 building these very very large you know
3:34 AI systems it's because they're all
3:36 trying to race for what you call you
3:39 know artificial general intelligence
3:41 right you have seen chat gp4 and you've
3:43 seen versions of it called you know
3:45 Gemini from uh Google you've seen you
3:49 know llama versions from um meta mistol
3:53 and so and so forth so you have multiple
3:55 different large language models all
3:57 trying to get towards what's called a AI
4:00 or what you perceive AGI to build
4:03 these the general feeling is that you
4:06 may have to build data centers which
4:08 have many hundreds of thousands of
4:10 accelerators in a data center or up to a
4:13 million accelerators in a collection of
4:15 you know data centers so today almost
4:18 all the investment is going into these
4:20 foundational large language models okay
4:23 so okay so they really this is all about
4:25 geni and llm and I think even here at
4:28 Juniper right I mean you almost see
4:29 every business now you know they either
4:31 have a customer facing initiative but
4:33 they also have internal initiatives to
4:35 be used gen LMS to increase the
4:39 efficiency of their business you know
6. Operational Efficiency with AI at Broadcom
4:41 curious to see at broadcom you know
4:43 inside of broadcom what big have you
4:46 found operational efficiency where
4:48 you're actually leveraging gen Ai and AI
4:51 inside of broadcom yeah I you know look
4:53 I I would say it's probably too early to
4:56 show that there is a significant
4:58 operational efficiency by by using you
5:01 know the Gen AI capabilities inside the
5:04 Enterprise but that said this is
5:07 definitely the time to start actually
5:09 investigating the art of what might be
5:12 possible you know one of the places that
5:14 for example within my team we're
5:15 starting to look at is uh we have a
5:18 customer support team and often times
5:22 the similar questions come into our
5:24 customer support you know team and then
5:26 they have to go look up some you know
5:29 documents that we have on how our chips
5:30 work and how they interact with the
5:32 customer systems and try to come back
5:34 with them with responses and what we're
5:36 trying what we're doing now is we've
5:38 taken you know one of these large
5:40 language models which are available via
5:43 cloud with one of our you know partners
5:46 and we've taken our internal data and
5:48 started to fine tune or train with our
5:51 internal data on these large language
5:54 models so that when the customers
5:56 questions come in through our chat bot
5:58 we're allowing the chatbot to answer the
6:01 questions now we haven't actually
6:03 enabled it with the customers yet but we
6:05 are actually having our customer service
6:07 team or our technical assistant team use
6:10 the chat bot to see the kinds of answers
6:12 that we're getting and what we're
6:13 finding is 60 to 70% of the time it
6:16 actually is giving a pretty good answer
6:18 but however this you know 20 to 30 plus
6:20 per of the time where the answer is not
6:22 what we would give so we are kind of
6:25 going through this process because until
6:27 we get to a point where we are comfort
6:29 able with almost all the answers that
6:31 the chat part is giving we obviously
6:32 won't fully enable it but we can see
6:35 some improvements in internal
6:36 productivity where there is another
6:38 filter being applied which is our humans
6:40 are applying a filter to the answer
6:42 these machines are giving so that's just
6:43 one example um there's other examples
6:46 too I mean when we think about broadcom
6:48 it is as much a software company in
6:49 terms of number of software Engineers we
6:51 have as much as we have silicon
6:53 engineers and we're using the tools
6:55 available you know you could refer to
6:57 them as co-pilot or other you know equal
6:59 tools we are Vex Ai and so on so forth
7:02 from Google and we're using those tools
7:04 to see can we make our software
7:06 Engineers more productive and lastly but
7:09 definitely not the least is building
7:11 silicon increasingly is a very very hard
7:14 job and getting silicon right the first
7:16 time is imperative if you're not going
7:18 to spend another 20 $30 million in doing
7:20 spins we're starting to look at can you
7:23 use AI to check our RTL code can you use
7:26 a AI to improve our you you know what we
7:29 call Design verification process before
7:32 we do tape out so there's multiple
7:34 different fronts none of them is a slam
7:36 dunk yet but you know it's worth kind of
7:38 probing to see where this leads and
7:40 that's what we're doing yeah yeah now
7. Build vs. Buy for AI Solutions
7:41 the customer support use case is one
7:43 dear to my heart that's one we're
7:44 working on also know maybe you want to
7:47 share a little bit because I've just
7:48 went through this exercise there's kind
7:50 of this build versus buy are you headed
7:53 down the you know buying the solution
7:56 off the off the shelf or is this more of
7:58 internal see if we can't build it
8:00 ourself you know using rag or some other
8:03 techniques to search through your
8:05 documents yeah so what I would say is um
8:10 you if you kind of think about the AI
8:12 and how the infrastructure is built for
8:13 AI there is an you know first a large
8:15 language model on top of that you're
8:17 kind of doing fine-tuning of that model
8:20 with your you know data specific to
8:22 yours and also often times remember this
8:24 data is proprietary to you and you don't
8:26 want to necessarily put this data out
8:28 there in the public domain right
8:30 and after you're fine-tuning the data
8:31 and then you're kind of trying to figure
8:33 out how you you know start making it
8:35 available for this engagement so clearly
8:37 we're not going to be out there building
8:39 our own large language model it's an
8:40 expensive Affair so we're going to take
8:42 an available large language model likely
8:45 from you know uh one of these Cloud
8:47 providers or somebody who's developing
8:48 this large language model and decide
8:50 whether we're going to do it you know
8:52 inside our private you know data centers
8:54 or we do it inside the cloud but either
8:57 way what is important is the data that
8:59 we're going to fine-tune it with and
9:01 train it with that is very proprietary
9:03 data to us and we want to make sure we
9:05 do it in a way that we don't leak that
9:07 data out and you know that is a decision
9:10 that each company will independently
9:12 make you know uh on what makes the most
9:14 sense for them so there's very efficient
9:16 ways of doing it rather than trying to
9:18 build your own large language models
9:20 which I don't think most Enterprises
9:21 will do yeah yeah I I think we're all in
9:23 this journey right now I think you
9:25 Juniper broadcom I think we're all in
9:27 the same Journey yes yeah so you think
9:29 about where we are in this AI Journey
8. Future of AI and AI Singularity
9:31 you know and if I put AI Singularity
9:34 Terminator you know AI passing the
9:37 Turing test you know what do you think
9:40 is this a 50-year journey 20e journey
9:43 where are we in this journey to AI
9:46 actually doing task on par as humans
9:49 well I wish I knew I'd be making a lot
9:51 more money uh but um you know if uh if
9:55 the book The the gentleman who coined
9:57 the term Singularity and in the book in
9:59 singular what he does talk about is
10:02 these are asymptotically increasing
10:04 capabilities that they achieve escape
10:07 velocity before you know it so any
10:09 projections that this might be much
10:11 further out than you know than it might
10:14 be based on historical development is
10:17 usually under underestimating how
10:19 quickly these things develop right and I
10:21 think that's why when you actually you
10:23 know hear the CEOs of these very large
10:25 megascale companies uh talking about
10:28 their massive CICS they say the risk of
10:31 not investing is much higher than you
10:35 know um than actually you know uh the
10:38 capital cost of investing and being in
10:40 the game and generally when you talk
10:43 when I uh speak with uh you know people
10:46 who are involved in these uh Investments
10:49 right now they say we probably at least
10:51 two generational models away okay from
10:56 what they believe might be the end State
10:58 and each generational model is 5 to 10x
11:01 more compute capacity than the previous
11:03 one so if I assume that the next
11:05 generational investment for the next
11:07 generational model is going to start you
11:09 know sometime summer of next year you've
11:11 got another 18 months for the F first
11:14 phase of first generation of that model
11:16 you add another you know 18 months to
11:18 the next generation of the model so
11:19 you're talking about 3 years from Summer
11:21 of next year so we probably are in a
11:23 4-year Journey at least you know of this
11:27 investment cycle happening before we
11:29 know know whether we are on the right
11:30 path yeah so so I don't know if you play
11:32 around with these these new llm agent
11:34 Frameworks now I mean because it does
9. New Software Programming Paradigms
11:36 seem like we're Ting a new software
11:39 programming Paradigm right we're getting
11:41 to the point now where we're using these
11:43 llms to actually solve tasks where I'll
11:47 give it access to a bank account ask it
11:49 to optimize a website so you can kind of
11:52 see we're getting to that point where we
11:54 couldn't build Solutions now that can
11:56 all sort of start you know once you give
11:57 an AI access to your bank account it's
12:00 kind of amazing what these things can
12:01 start doing but you know back to your
12:03 distributed programming technique you
12:05 know do you see a new software
12:07 programming Paradigm coming down you
12:09 know AI programming versus Cloud
12:11 programming how do you see our software
12:13 Paradigm programming Paradigm changing
12:15 here um well one first I will never give
10. Networking Infrastructure for AI
12:18 AI access to my bank account two um I I
12:22 don't know if I can speak enough about
12:25 you know changes to the uh software you
12:28 know model and you know paradigms and so
12:30 on and so forth um but however what I do
12:33 have very strong opinions on is how the
12:36 infrastructure for AI needs to be you
12:38 know built right and often times people
12:41 will compare cloud computing to you know
12:44 AI you know uh machines and what I'd
12:47 like to point out is when you look at
12:49 cloud computing cloud computing is a
12:52 very different you know U you know
12:54 Paradigm than uh AI because in cloud
12:57 computing it's about virtualization
12:59 because if you think about cloud
13:00 computing how did cloud computing come
13:01 about you had a CPU that had far more
13:04 horsepower than any particular
13:06 application could use and then you said
13:08 Hey how do I make more use of this you
13:11 know CPU so I'm going to virtualize the
13:13 CPU so I can run multiple different
13:14 applications on it right and then you
13:16 start to worry about how do I prevent
13:19 isolation so that one virtual machine is
13:21 not talking to Virtual Machine there's
13:22 no leakage of information from one to
13:24 the other how do I increase utilization
13:25 so on so forth and so in cloud computing
13:28 because was about increasing the
13:30 efficiency of the CPU generally the
13:32 networks were not that stressed yeah you
13:34 built large megascale data centers and
13:36 there was a lot of eastwest traffic but
13:38 the amount of bandwidth on the network
13:41 was only the amount of bandwidth that
13:42 you had per CPU which was probably 25
13:44 gigs at some point and 50 gig barely
13:46 pushing 100 Gig but if you look at
13:49 machine learning it's the completely
13:51 different issue no one applica actually
13:55 no one GPU can run a machine learning
13:58 application
13:59 you know especially if you think about
14:00 these large language models you need
14:02 many thousands of gpus many hundreds of
14:04 thousands of gpus to be attached
14:06 together with the network to look like
14:08 they're one large machine right and now
14:11 the other thing that you find is on each
14:13 of these machines these accelerators the
14:16 amount of bandwidth coming out of it is
14:17 no longer 50 gigs or 100 gigs it's 400
14:20 gigs 800 gigs and some of these road
14:22 maps that you see they have up to 10
14:24 terabits of IO coming out of each of
14:27 these accelerators
14:29 so networking as we have seen before is
14:31 going to go through a paradigm shift
14:33 with regards to how large these networks
14:35 are going to get and network is going to
14:37 become the fundamental for how these
14:39 accelerators are going to build be built
14:41 and that's why I think Juniper is in an
14:43 awesome you know place to be at the
14:45 center of what I call the network is the
14:48 computer right and you know eventually
14:51 obviously it might change how the
14:53 software paradigms change in terms of
14:55 how the software programmer interacts
14:58 with the machine what value does a
14:59 software programmer add versus what does
15:01 a large language model already abstract
15:03 a as value it can provide uh but I'm
15:06 definitely not the guy to speak about it
15:07 but I see a paradigm shift coming in how
15:09 networks are going to be utilized and
15:11 needed well I mean I think it's clear
15:13 what like you said the what Juniper
15:15 calls networking for AI right yeah you
15:17 know we definitely have the x86 front of
15:18 the house and now we're going to have
15:20 this GPU back of the house yeah and that
15:22 networking infrastructure is definitely
15:24 going through a paradigm shift you know
15:26 800 very high speed connections in
15:29 between all these epu clusters to move
15:31 data around yep now the other Paradigm
15:33 Shift we talked a little bit before the
15:34 show here was around you know what AI
15:37 use cases you know is that going to
15:38 extend outside the data center you know
11. AI Use Cases Beyond Data Centers
15:41 are we are you seeing the need for
15:42 bigger larger faster networks to handle
15:46 these AI use cases going outside that
15:48 are actually running in these data
15:50 centers no look that's you know what
15:52 people today call the $600 billion
15:55 question okay to give you a rough idea
15:57 of how this number $600 billion comes
15:59 about is you know Nvidia they say you
16:02 know roughly to 100 to $150 billion is
16:04 their annualized run rate and let's
16:07 assume you're spending $150 billion on
16:09 gpus you're probably spending at least
16:12 half that much on building the data
16:13 center infrastructure and the softare
16:15 and everything that goes around it so
16:16 now you're talking about $300 billion a
16:18 year of spend now if you assume the
16:21 people who are building these data
16:22 centers at $300 billion of spent are
16:24 hoping to get at least a 50% margins on
16:27 their business they got to generate
16:29 about $600 billion a year in Revenue
16:32 right so there better be a lot of
16:34 applications coming in into the 600
16:37 billion to sustain the $600 billion of
16:40 Revenue at the user level right and
16:43 clearly for these users to be able to
16:46 extract value eventually for these maths
16:48 to add up these are users who are
16:50 sitting in the home or these are users
16:52 sitting in the Enterprise who are trying
16:54 to access this intelligence you know
16:56 that is probably being fine-tuned into
16:59 the at the data centers but eventually
17:01 being delivered to them either at their
17:03 home or at their place of work or let's
17:05 say you know somebody is on the Fe is at
17:08 the field you know looking at a wi
17:09 turbine or looking at an hbac system
17:12 trying to figure out how to repair it
17:13 all of this information has to be
17:14 delivered to the user and so the
17:16 networks are what connects them from
17:18 within the data centers over the service
17:20 provider eventually to The Last Mile
17:23 towards the edge and I would say you
17:26 will find use cases you know will um we
17:29 will figure it out in the next year or
17:31 two but at the end of it it's going to
17:33 push networks well I I I don't know if
17:35 you tried this apple Pro Vision or the
17:39 face uh meta I mean I think the use case
17:41 that's going to drive these bigger pipes
17:43 is going to be around augmented or
17:44 virtual reality yes you know what I saw
17:46 coming down the pipe with the augmented
17:48 reality is kind of that remote worker
17:50 use case and that definitely is going to
17:52 require bigger pipes to handle those use
17:54 cases where you're doing a remote
17:56 augmented reality use case out in the
17:58 field bu somewhere yeah no I agree look
12. AI at the Edge
18:00 I think sometimes the word remote has a
18:03 bad annotation now everybody's like oh
18:04 is that working from home working
18:05 remotely and I would actually probably
18:07 change it to the field worker use case
18:10 right people are in the field you know
18:13 you cannot have a robot today who is as
18:16 you know capable and agile as a human
18:18 but you may have humans on the field
18:21 solving problems that you could actually
18:23 feed them information that makes them
18:25 far more productive right we will see a
18:27 lot of those applications
18:29 now now broadcom has been a big part of
18:31 my career you know I'm in the networking
18:32 business you know i' we've got broadcom
18:35 inside of our access points switches
18:37 routers everywhere um you we talked
18:40 about accelerators going out to the edge
18:43 yeah you know so we know we have these
18:45 large data centers being built with big
18:46 GPU clusters to train and run big gen
18:50 models but we also have kind of this
18:52 thing moving out to the edge yes where
18:54 broadcom has a big play you know what
18:56 use cases do you see happening at the
18:58 Edge that's going to be driving you know
19:00 am I going to be bringing all my video
19:01 back to some data center or am I going
19:04 to be doing more and more of this AI out
19:06 towards the edge of the network
19:07 somewhere yeah if you look at
19:09 specifically in the uh equipment that we
19:12 build and kind of deploy at the edges
19:14 you know one simple you know use case
19:16 for it is uh set toop boxes broadcom is
19:18 in the business of set toop box you know
19:20 businesses and business and we build the
19:23 setop Box chips we are embedding you
19:25 know neural engines inside our setop box
19:28 you know chips
19:29 where you are able to do things along
19:31 the lines of security troubleshooting
19:33 because otherwise for the same thing
19:36 where a cable provider might have
19:37 otherwise had to move you know send a
19:39 truck in to replace and troubleshoot and
19:41 stuff you're able to actually improve
19:43 the performance of the end user those
19:46 things that we could do on the set up
19:48 box you know whether that is security as
19:49 I was saying troubleshooting it and
19:51 being able to actually look at your you
19:52 know link you know health and so on and
19:54 so forth so and and there's you know I'm
19:57 I'm uh pretty
19:59 um you know confident that even in the
20:01 Wi-Fi space even a lot of it is about
20:03 getting your Wi-Fi connections right
20:05 your signals correction correction
20:07 happening you'll also start to see some
20:09 of these AI neural you know engines
20:12 going right into the chips to improve
20:15 the user experience and make it more
20:16 secure and more available yeah well I
20:18 can tell you you know working with my
20:19 Healthcare customers there there's
20:21 definitely visions of doing Wi-Fi radar
20:24 at the edge for fall prevention you know
20:26 I've seen a lot of video at the edge now
20:28 where they basically want to use a lot
20:30 more computer vision so I can definitely
20:31 see where computer vision in that
20:33 process is going to be moving towards
20:35 the edge cuz I'm not sure I want to
20:36 bring all that video traffic back to the
20:38 data center so I think that's probably a
20:40 good example where we're going to start
20:41 seeing more broadcom at the edge
20:42 becoming more and more relevant to this
20:44 AI Venture true I think look you know uh
20:49 more likely than not some of the video
20:50 might still be delivered from the data
20:51 center but the latency with which you
20:53 deliver you know that video and then
20:55 being able to actually do any
20:57 localization or as as you were saying
20:59 argumentation of that in the you
21:01 specific to that particular location or
21:04 that user is definitely where the
21:05 silicons come into play well R I want to
13. Closing Remarks and Future Outlook
21:07 thank you for joining this episode of B
21:09 FR talks you know and maybe for our
21:11 audience any last words of vision where
21:13 you see this headed you know 5 years
21:15 from
21:16 now oh 5 years from now I I hope we will
21:20 look back and say was in in outstanding
21:23 run and I I think we are a generational
21:26 you know opportunity especially for
21:27 those of us who are in the network
21:28 business right you know a few years ago
21:30 if we built a 50 terab switch or 100
21:32 terabit switch we would be looking at it
21:33 and saying who's the customer now we
21:36 have customers you know knocking on our
21:38 door saying hey when is your next switch
21:40 when is your next switch so I see this
21:42 as a tremendous opportunity for you know
21:44 Juniper for broadcom and everybody who
21:47 in the networking business because the
21:48 network matters Y and we are at the
21:51 heart of distributed computing and we
21:53 are at the beginning of a long cycle for
21:55 distributed computing well I I think
21:57 what I tell people you know internet
21:58 networking on part of power and
22:00 electricity you have to choose what you
22:01 want but anyway R I want to thank you
22:03 for coming today it's been great having
22:04 you and I want to thank everyone here
22:06 for joining B Friday talks and look
22:08 forward to seeing you on the next
22:09 episode
22:11 [Music]