AI Skeptics: Get Started On Your AI Journey
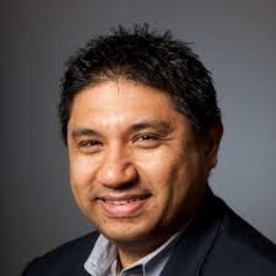
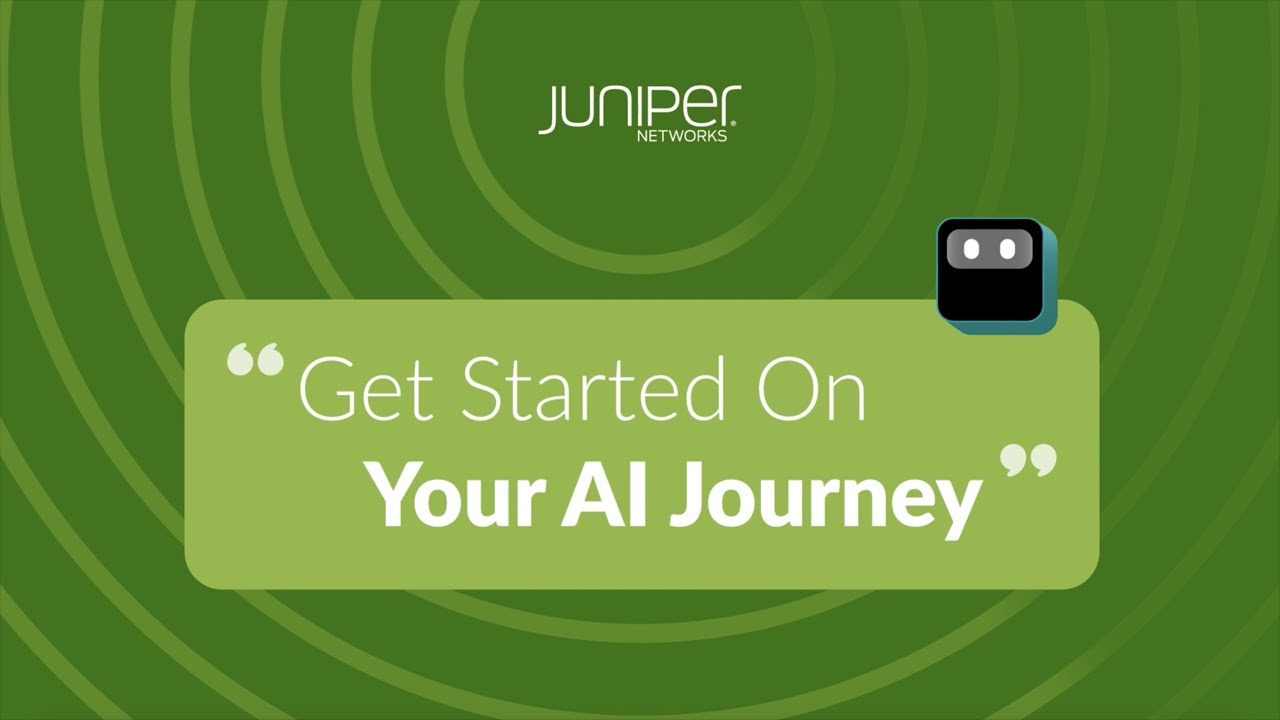
You've an AI solution and are ready to get started on your AI journey. Where do you begin?
AI isn't a magic wand you can wave to fix all your company's problems. It takes time and effort to onboard AI and set it up for success, just as it would a new hire on your team. In this video, you can hear about some ways to integrate AI into your processes and work with it to continually optimize results. Hear real stories from IT professionals who've lived it. You’ll learn what it took for IT professionals like you to integrate AI into their day-to-day operations and the business outcomes they were able to achieve.
You’ll learn
How to set up AI for success
What it takes to integrate AI into your day-to-day operations
Who is this for?
Host
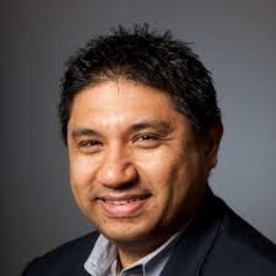
Guest speakers
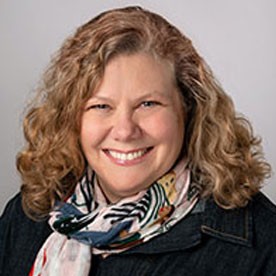
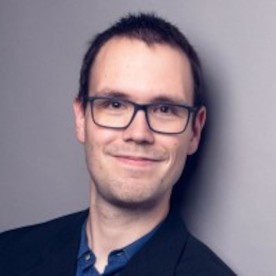
Transcript
0:00 [Music]
0:06 hello everybody i'm zeus caraval
0:07 principal analyst with zk research and
0:09 i'm hosting uh this episode of ai
0:12 skeptics
0:13 in our previous conversations of the ai
0:15 skeptific series we've heard about
0:17 perspectives around how
0:19 ai or artificial intelligence will help
0:21 it practitioners
0:23 and technology channel partners
0:25 accomplish more
0:26 in today's conversation i'll be joined
0:28 by uh sharon mandel ceo of juniper
0:31 networks uh jerome say hi
0:34 hi uh thank you for having me i'm
0:37 excited to be here today
0:39 and we're also joined by juniper
0:40 network's ambassador christian scholes
0:42 of axion networks and solutions
0:44 uh and so what you say as well christian
0:48 yeah hi everybody um yeah thank you for
0:50 having me um great to be here
0:52 and it's a it's a it's a great duo to
0:54 have because they both share some
0:55 takeaways on what it's like to actually
0:57 implement the ai and work alongside it
1:00 and uh you know sharon i'll start with
1:02 you um
1:03 i you know you and i have been talking
1:05 about ai for quite some time and it's
1:07 kind of a funny topic because
1:09 i think everybody kind of conceptually
1:11 understands the value although there's a
1:13 lot of skepticism and
1:15 uh trepidation around it so
1:17 just talk a little bit about the process
1:20 you went through rolling out mist inside
1:22 juniper well sure well rolling out mist
1:25 is is
1:26 fairly straightforward um because a lot
1:29 of it comes out of the box so
1:31 um i think for a lot of people
1:33 they hear ai they think oh my god i have
1:36 to hire
1:37 you know an army of data scientists i
1:40 have to do
1:41 all of this work and
1:44 in this case the ai is built in and so
1:49 it
1:50 it it starts with
1:52 models that are trained and then it gets
1:54 to understand your network and
1:57 so it's it's not a lot
1:59 different than um
2:02 installing any other wi-fi network
2:04 except that
2:05 it's easier because we we build in the
2:08 pre-configuration and and make that that
2:11 simpler i think the other challenge is
2:13 you know people start to
2:15 wonder oh my god you know are my is my
2:18 job gonna go away as a network
2:20 administrator
2:21 and i think that um very quickly people
2:24 understand that that's not true right
2:27 because
2:28 um
2:30 the the situation was they always had
2:32 more work to do than there were people
2:35 to do it um they were solving problems
2:39 um that you know were like finding a
2:41 needle in the haystack because the scale
2:43 of the data that had to be analyzed to
2:45 solve them was so large and so really
2:48 what what introducing ai into that mix
2:52 did was it took away
2:54 the mundane problems it made some of the
2:56 very complex ones much easier
2:59 to solve and then they got to go back to
3:01 the work that they really like doing
3:03 which is architecting and designing
3:05 networks not
3:07 um troubleshooting them
3:09 yeah it's it if the analogy you used
3:11 about it sort of being in bed it's
3:12 interesting because it's not unlike an
3:14 automobile today right there's ai in
3:16 your car that helps with lane change
3:18 alert parallel park assist you know
3:20 adaptive cruise control and it's not
3:22 like we need to be data scientists to
3:23 drive today we just need to go in and it
3:26 makes us smarter better drivers
3:27 similarly it sounds like your
3:28 implementation of ai made your engineers
3:30 smarter better engineers
3:32 so uh christian uh now you've been using
3:35 this a couple of years now so why don't
3:37 you talk about your journey
3:38 yeah um i started with uh
3:40 i was around 2020 when i started with
3:43 mist
3:44 i started with adopting it in my home
3:47 so i built this new house for me and my
3:50 wife
3:50 and obviously it
3:52 always was my dream to build a smart
3:54 home a true smart home so i started with
3:56 roughly 200 sensors i'm currently at
3:59 roughly 300 to 350 sensors
4:02 and yeah as you can imagine with that
4:04 amount of sensors
4:06 you don't want to administer all of them
4:08 by hand so you basically want to to
4:11 architect what you want to achieve like
4:13 i want a certain temperature or i want a
4:15 certain air quality or
4:17 yeah whatever you feel comfortable with
4:19 and then you want the sensors to
4:21 interact with each other and the
4:23 orchestrator or the ai part to basically
4:25 control all of that
4:27 to yeah
4:28 fulfill exactly what you asked it to do
4:31 that's interesting so uh i can't wait to
4:33 see that's the next analyst summit we
4:35 should do at your house um
4:41 and uh another question for both you and
4:43 sharon i'll start with you again um how
4:45 long did it start seeing did it take to
4:47 start seeing value from ai
4:51 well you know each use case is a little
4:53 bit different right so um you know you
4:56 asked me specifically about miss that
4:59 happened very very quickly because it
5:01 came with a certain
5:03 um
5:04 set of um
5:07 features and and and problems it could
5:10 solve straight out of the box right so
5:12 so immediately we we could see
5:15 um
5:16 we could see a
5:19 ticket resolution things like that um
5:21 other solutions uh have different
5:24 requirements to get them going so
5:27 um as as we've implemented some chat bot
5:30 technologies right which people consider
5:32 ai because in theory
5:35 you know it's it it it's it's talking to
5:37 a machine and getting answers um in that
5:40 case there there was a lot more work to
5:43 do to get effectiveness and maybe we
5:45 never with with the particular
5:47 technologies we've played with so far
5:49 we've never gotten quite as far as we'd
5:51 like because
5:52 there was so much work we had to do
5:55 which required an army of people we
5:57 didn't have
5:58 to prep the data
6:00 to create a knowledge base to structure
6:02 questions properly
6:05 that
6:06 it
6:07 we never really reached that full
6:09 potential so i think you know people
6:11 have to understand that there's a range
6:14 of of ai type solutions all the way back
6:18 to the the simplest which is just
6:20 um you know predictive analytics right
6:23 where where you're using
6:25 ml models to
6:26 to draw conclusions and take next
6:28 actions and in that case
6:30 um you know you may be building it from
6:33 the ground up and then really preparing
6:36 the data is the largest job and can take
6:39 some time so i think each company has to
6:42 understand
6:43 um the value of the use case that
6:45 they're trying to solve really
6:47 understand the products they're buying
6:50 what needs to go
6:52 into
6:53 um
6:54 training the model there is it is it
6:56 trained out of the box and then is gonna
6:59 adapt and learn more about you and get
7:01 more specific about you
7:03 over time or are you building that model
7:06 from scratch because there's no way that
7:09 that problem can be solved because it's
7:11 truly uniquely about your company and
7:13 then there's going to be a different
7:14 level of effort and you ought to be
7:16 convinced that the problem you're
7:18 solving
7:19 is has that business value that that's
7:21 worth that additional effort
7:23 yeah so it sounds like there's in some
7:25 ways two types of ai there's the
7:27 embedded
7:28 uh kind of invisible ai that people
7:30 don't even know is there that helps the
7:31 network run better
7:33 right and then there's other ai that
7:35 the engineer would interact with that
7:37 does need a bit of tweaking and training
7:39 and things like that like with chat bots
7:41 that actually changes the way they work
7:42 is that a good way to think about it
7:44 yeah and again you know ai when i i'm
7:47 speaking about ai i'm not speaking just
7:49 about ai to serve the network but
7:51 ai to solve a broad range of problems
7:53 right so we use ai to make decisions in
7:56 supply chain
7:58 we use
7:59 you know the chatbot example
8:01 we have chat bots at the help desk we
8:03 have chat bots through the procurement
8:05 tools we have chat bots you know to help
8:08 our employees navigate
8:11 how to find things in in the marketing
8:13 department so
8:14 they get applied to all different kinds
8:16 of problems and each
8:18 implementation um you know watson is
8:21 different than servicenow's um chatbot
8:24 which is oracle's chatbot and what they
8:26 expect you to do and in some cases
8:29 some companies that provide those
8:31 technologies out of the cloud
8:33 they bring as part of their professional
8:35 services offerings the people who do all
8:37 of that data work because they've done
8:39 it a bunch of times before with
8:40 companies and they're not learning on
8:41 the fly so there's there's a whole range
8:44 of level of effort depending on the
8:46 nature of the solution
8:48 yeah the ios caution people do when
8:50 they're using ai to interact with
8:51 customers
8:53 make sure it works well because you can
8:55 actually have a very negative reaction
8:56 if it doesn't work well i think we've
8:58 all experienced that chat bots that
9:00 you know don't really understand what
9:01 you're saying but they've come a long
9:02 way you know in the last five years and
9:04 so i think for companies that had a bad
9:06 experience with let's say five six years
9:08 ago it's really good again i'm gonna
9:10 bounce back to you what was your you
9:12 know you set up in your own house which
9:14 is kind of cool can you just kind of
9:16 double click on the learning process
9:18 that you went through for your iot setup
9:21 yeah well at first it was just adding
9:23 all the sensors and that's basically
9:25 where all the magic starts because at
9:27 the beginning i just had a bunch of
9:28 sensors and yeah i had a pre-trained
9:31 model for this whole ai setup but it
9:34 wasn't really aware of what i wanted in
9:36 my house so it was in this constant
9:38 learning phase for about i would say six
9:41 to seven months
9:42 um
9:43 and at some point i even had to tell it
9:44 okay if there is now a snowstorm in june
9:48 for example because sometimes the
9:50 weather is just crazy for one day um
9:52 feel free to ignore that and stuff like
9:54 this because uh yeah if i'm if i
9:56 wouldn't have told it that um snow and
9:59 summer is something that it has never
10:00 experienced before so even for me that
10:02 was very weird so uh
10:04 yeah
10:06 and it was very interesting because at
10:08 at the beginning it's it almost feels
10:10 like a very
10:11 dumb piece of software
10:13 um and then the more you interact with
10:15 it the more you train it the more you
10:18 work with it every day almost like you
10:20 would work with a new co-worker the more
10:23 it learns about your let's call them my
10:26 needs like what temperature does he like
10:27 or what humidity does he like and
10:30 when do i need to open the window and
10:31 close the window when do i need to shut
10:33 the blinds because it's getting too
10:35 bright and stuff like that um
10:37 yeah now it's just working it's like
10:39 magic i mean i'm walking through my
10:41 house wherever i come into the room the
10:44 light gets turned on when i leave the
10:46 room the light gets turned off and yeah
10:48 it basically learned all of that from
10:49 the behavior that i showed it
10:52 so uh yeah yeah
10:54 that's interesting
10:55 i find sometimes those systems can
10:56 always get too smart i've got some smart
10:59 stuff
11:01 oh yeah
11:02 it shuts things off and you don't want
11:04 it to and things like that but i guess
11:05 that's that's all part of the learning
11:07 process what does it take to sort of vet
11:09 an ai solution or vendor like how do you
11:12 evaluate them
11:13 yeah so
11:14 i think first of all
11:16 you have to really understand the
11:18 problem you're trying to solve
11:21 and
11:22 then
11:22 understand
11:24 what went into the vendors thinking
11:27 about the problem they were solving and
11:31 the first thing is are they
11:33 closely enough aligned that
11:36 the models that got baked into theirs or
11:39 that they're going to apply to your
11:40 problem are actually applicable and
11:44 going to solve the problem after that
11:46 you then have to especially in the case
11:48 of sort of the prebaked
11:51 ai you need to understand the types of
11:53 data that it was trained on and whether
11:56 again that data closely enough
12:00 reflects or is broad enough
12:02 to at least start creating that value
12:05 out of the box so that it can learn and
12:07 continue from there you have to
12:09 understand
12:10 how does the vendor think about keeping
12:13 its models up to date so you know you
12:15 saw
12:16 during the pandemic certain ai solutions
12:19 that
12:20 were built based on
12:22 well-understood behaviors about how the
12:24 world worked
12:26 so you know for retail there were you
12:28 know years and years of data about
12:30 people going in and out of stores all of
12:32 a sudden it stopped well since the data
12:34 is the fuel for the model suddenly that
12:37 model didn't work anymore in answering
12:40 some of the questions that retails one
12:42 example you saw
12:43 you saw a number of others obviously
12:46 certain things in the supply chain
12:47 changed pretty radically so
12:49 you have to understand where data
12:52 discontinuity might break that
12:54 technology
12:55 and what's your likelihood for that so
12:57 it's it's it's really all about
13:00 primarily that many of the algorithms
13:02 that are the basis for ai
13:05 they're well understood statistical
13:07 analysis and so first of all you know
13:10 are they applying the right one to the
13:11 problem most likely if they've had any
13:14 success in the market they are but then
13:16 it's really about the fuel and the data
13:18 that that feeds that model
13:20 how do they deal with the drift that can
13:23 happen so those are some of the
13:25 considerations i think you know you need
13:27 to look at but
13:28 it's always first
13:31 does does the solution match my problem
13:33 and do i really deeply understand my
13:35 problem that i'm trying to solve and i
13:37 think that underscores the importance of
13:38 pre-built uh ai systems uh obviously
13:42 you know the the more
13:44 turnkey it is the
13:46 the easier it is to implement i think
13:48 you know from an engineer's standpoint
13:50 where i've seen
13:51 some hesitation uh from it pros
13:54 is that uh there's a fear that you need
13:56 to be a programmer and a data scientist
13:58 and frankly um as part of the media
14:01 right i read a lot from media the we
14:04 that was a big theme many years ago that
14:06 everybody in the world would turn into a
14:08 programmer and everybody would be have
14:10 to become a data scientist
14:11 and i think that scared some people and
14:13 that's not true i do think from a skill
14:15 set perspective though um
14:18 engineers do need to think more about
14:19 becoming a software power user right but
14:22 that's with or without ai you look at
14:24 the sophisticated network systems today
14:26 not just from yourself but even from
14:28 your competitors everything's you know
14:30 tapped into with apis you know every you
14:32 know everything's available via sdks and
14:34 things and so um you know software is
14:37 the way forward and that lets you access
14:39 a lot of these great features so like i
14:41 said you don't need to be that the data
14:42 scientist and things like that but you
14:44 do need to be comfortable with software
14:45 so
14:46 um you know and christian is that would
14:48 you like to you know maybe expand your
14:49 own insurance i do
14:51 yeah i mean it gives you a lot more
14:52 flexibility with this new skill set that
14:55 you build your like you said you're
14:56 becoming more of a designer you don't
14:58 need to be the one
15:00 um coming from a network implementation
15:02 point of view where you go to every
15:04 switch and configure every vlan on every
15:06 port you simply design okay this is the
15:08 vlan that i want here are my devices go
15:11 for it um so yeah you have the weekends
15:14 back you have all those night shifts
15:15 back so uh
15:17 i think it's a it's a great thing so
15:19 it's not threatening the jobs at least
15:21 from the way that i see it and what i've
15:23 seen with customers
15:25 is it's not threatening the job it's
15:27 giving them more air to breathe so they
15:29 can focus on the important business
15:31 things and not need to waste any time on
15:34 those yeah little
15:36 times the reality is i'm not going to
15:38 sit here and say that
15:40 the infusion of ai in technology isn't
15:43 going to get rid of some jobs because it
15:45 is but
15:46 this this is just part of technology
15:48 maturation we don't have mainframe
15:50 engineers today we don't have people
15:51 working on token ring you know things
15:53 change over time and everything right
15:56 and every engineer i talk to the one
15:58 piece of advice i give them is if you're
16:01 doing things today forget about your the
16:03 company you work for think about your
16:04 own selfishness your own needs and your
16:06 own job if you're doing things today
16:08 that aren't
16:09 additive to your resume right don't do
16:12 them find a way to automate them out of
16:14 your job and that's really the power of
16:17 what you were talking about christian
16:18 that ai can do it's like you don't have
16:19 to come in on weekends you don't have to
16:21 spend hours and hours updating vlans and
16:23 frankly nobody's going to hire you for
16:25 your next job yeah because you can do
16:27 those things right so
16:28 exactly
16:30 and when i think about um you know ai
16:32 and the network i do think one of the
16:33 things to look for though is a solution
16:36 that works across the network it is
16:39 valid because the experience that a user
16:42 or customer gets is based on how
16:44 applications work across the network not
16:47 just within specific silos and
16:49 historically we've treated networks and
16:51 styles and that's why even today you'll
16:53 see
16:54 ai solutions for wi-fi ai solutions for
16:56 sd-wan ai solutions to the data center
16:59 things like that and and while those
17:01 may
17:02 work
17:03 well they don't really give you a view
17:06 of the end-to-end network and so you
17:08 know in data science says there's an
17:10 axiom right that states that good data
17:11 leads to good insights well
17:13 silo data will lead to solid in size or
17:15 fragmented insights and i think uh you
17:18 need to have that kind of end-to-end
17:19 view so here i'm just i don't know
17:22 yeah so so zeus
17:25 i i
17:26 as kind of a cio who has to not only
17:29 integrate the network but
17:31 a broad series of applications and
17:34 experiences both for our customers and
17:37 and our employees
17:38 um
17:39 across a broad spectrum of you know
17:42 functionality
17:44 because
17:45 you know the pre-built ai solutions
17:49 they tend to be fairly narrow in focus
17:51 again because you have to curate
17:54 uh a data set
17:57 um that's
17:59 unique to that problem and so
18:02 we haven't yet seen the company
18:04 you know sort of like oracle and sap
18:07 came in and said well i'm going to
18:08 integrate everything from financials
18:11 through supply chain and then you know
18:14 work their way back to you know order
18:16 management the sales front office right
18:18 you've seen these companies come in and
18:20 and provide
18:22 application suites that that you know
18:25 cover very broad spectrums of
18:26 functionality and it took them years to
18:28 build it you haven't really seen that
18:30 use it you know across
18:33 that sort of cross-functional ai view so
18:36 you know i i joke sometimes that we're
18:38 going to have the war of the bots right
18:39 where
18:40 the bot from vendor a is gonna give you
18:42 a different answer and start fighting
18:44 with the bot from vendor b
18:46 um and i what i what i see starting to
18:49 happen is um some of the
18:52 sort of incident management solutions
18:55 that people tend to interact with right
18:57 so a person submits a problem
18:59 and then a person's on the receiving end
19:02 of that you know it might go through
19:03 some escalation path but ultimately you
19:05 get to a subject matter expert
19:07 who
19:08 you know will solve your problem
19:10 well
19:11 you know as you've seen in this world of
19:13 sre and devops it's rarely one subject
19:16 matter expert if the problem is complex
19:18 enough well now some of these solutions
19:20 are starting to treat the bots as
19:23 um
19:24 a part of the conversation um and so you
19:27 might have one or more bots combined
19:29 with five people
19:31 offering insight into
19:33 what might be the solution to the
19:35 problem
19:36 but that's still a step away
19:38 from
19:39 you know some kind of integrated
19:41 perspective or
19:43 integrated world view that i think will
19:45 will ultimately need to have
19:48 in certain areas right um
19:51 but it is because of the nature of how
19:53 these solutions are built um and how
19:55 important curated data sets are
19:58 yeah i think that's uh
20:00 the the war that both already happens in
20:02 social media
20:07 we just we don't want to come to our
20:08 corporate systems though so
20:10 um
20:11 anyways i just have one more question uh
20:13 for both of you uh and we'll do this um
20:16 you know i'd like to ask this question
20:17 because i think it's helpful for people
20:19 watching this uh what's one piece of
20:22 advice that you would give to it pros as
20:26 the 19 leaders as they embark down their
20:29 i.t journeys and that or their ai
20:31 journey they'll actually go first and i
20:32 think
20:33 it kind of parlies off what christian
20:35 said
20:36 um i think you need to be patient with
20:38 ai and you need to understand um and
20:40 this can actually help you evaluate real
20:42 ai solutions versus fake i solutions ai
20:45 washington is rampant out there and
20:46 every system used says they use ai
20:49 and a good way to understand if
20:51 something's real ai or not
20:53 is to see how it progresses and how it
20:55 learns over time as christian said at
20:57 first it may be kind of dumb you may
20:59 look at it and go well this isn't very
21:01 helpful at all but over time
21:03 it does get better and it gets smarter
21:06 and it understands your environment
21:07 better in fact i think juniper does a
21:09 nice job of highlighting the efficacy of
21:12 it and you know being actually
21:14 demonstrating you know the
21:16 the quad and quantifying the how it's
21:18 changed over time and so real ai isn't
21:21 just fancy rules based systems and if
21:23 you go with them one of those those
21:24 rules based systems um you're going to
21:26 be constantly updating it and constantly
21:29 changing the rules
21:30 um and so you have to understand that ai
21:32 is not perfect out of the gate but it
21:34 does get better over time and it will
21:35 help you more and more and so you need
21:37 to be patient with it and so sharon
21:40 what's some advice from you
21:42 the hardest part of adopting technology
21:44 is fear of change right and
21:47 and
21:48 to your point that you made earlier is
21:50 this which was
21:52 you know yeah some things are going to
21:54 change and some types of roles and work
21:56 will go away
21:58 um
22:00 it's part of the natural evolution of
22:02 technology so as you know i i say
22:05 experiment right try try different
22:08 things try different flavors see what
22:10 works for you
22:12 understand the nature of your company
22:14 and your business
22:16 and experiment um
22:18 and and some things are going to be you
22:20 know as we've discussed much more useful
22:23 straight out of the gate and some are
22:25 going to be um acquired taste because
22:27 the more the more unique
22:30 you know where
22:31 you when you apply
22:33 ai technology to something that makes
22:36 you really special and unique you're not
22:38 likely to find it out of the box right
22:40 again because of that
22:41 curated data so
22:43 you know i say kind of play with a lot
22:45 of different things and and and try
22:48 um and learn yourself from them um where
22:52 you're going to have to change and adapt
22:55 and
22:56 um
22:57 like i said just don't be afraid and get
22:59 people comfortable
23:00 um slowly and that that may go back to
23:03 your patience right i mean as is always
23:05 the case
23:06 um
23:07 technology
23:08 at some level it creates that that's
23:11 disruptive creates change that people
23:13 are uncomfortable with and i think part
23:15 of being patient with ai is being
23:17 patient with the people you're trying to
23:19 get
23:20 to use it so um a lot of experimentation
23:25 experiment and uh be patient experiment
23:27 christian uh what advice would you give
23:29 here yeah i can also echo that don't be
23:31 afraid of it um when i when i started
23:33 with this it almost felt like i'm
23:35 teaching a child or a new colleague all
23:38 of the stuff and at first it felt like
23:40 i'm doing more work
23:42 and i thought like okay if i now have to
23:44 explain everything and basically teach
23:46 everything will this really benefit me
23:48 but after some time you will see the
23:50 benefit and
23:51 the model learns and everything comes
23:53 together and yeah like i said you will
23:56 get
23:57 more
23:58 more free time to do the stuff that you
24:00 really want to do and less of the
24:02 hideous little tasks that yeah just
24:05 waste your time so be patient work with
24:07 it
24:08 um yeah make it your colleague basically
24:11 explain everything at first it's it's
24:13 yeah you need to explain everything of
24:15 course because for the ai it's brand new
24:17 what you're just telling it
24:18 depending on the type of model that you
24:20 use but uh yeah it will be
24:23 so
24:24 worth it so don't be scared
24:27 all right well uh that was a great
24:28 discussion i'm just gonna i think wrap
24:31 up by saying uh
24:33 alekko said don't be afraid of it ai has
24:36 already proven in many industries it
24:38 makes us better drivers it makes contact
24:40 center agents smarter right and i think
24:42 from an engineer's perspective it's
24:44 going to make you a better smarter
24:46 engineer it's going to let you do a lot
24:48 more fun things right and as a former it
24:51 pro i can tell you nobody here in it pro
24:54 to sit and look through router logs and
24:56 update vlans all day long to just that
24:58 just boy that's just if you get
24:59 enjoyment out of that maybe
25:01 you know um you know
25:03 you should you know seek out some other
25:04 forms of enjoyment or something so
25:06 anyways uh ai's coming uh there's really
25:09 you know it's gonna be infused
25:10 eventually in everything that we do it
25:12 already is on the consumer side so uh
25:15 you know christian sharon thanks for
25:17 joining me today i'm zeus caraval from
25:18 ck research and thanks for watching this
25:20 episode of ai skeptics
25:24 [Music]
25:29 you