Juniper Mist Location Services
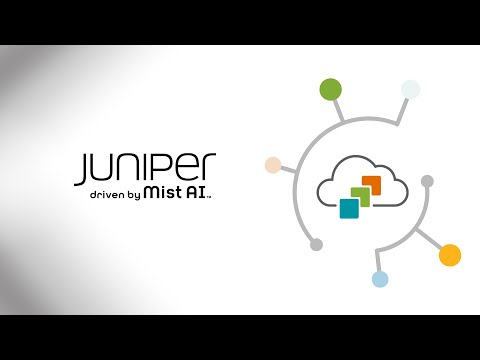
Learn about the different technologies that come together to make Juniper Mist Location Services work.
You’ll learn
How Mist Location Service works
Key challenges for providing consistent user-experience
Who is this for?
Transcript
0:00 foreign
0:06 SDK is responsible for receiving the ble
0:10 RSSI information and combining it with
0:13 sensor data from the mobile device and
0:16 sending that back to the cloud every
0:18 second while the mobile device is moving
0:21 in this machine Learning System
0:22 continuously adapts to changing RF
0:25 environments without the need for site
0:27 surveys
0:29 unlike single antenna ble designs Mist
0:32 uses a single transmitter to drive eight
0:35 unique directional antennas each beam
0:38 contributes to the likely location of
0:40 the device the Miss technology examines
0:42 all the probability surfaces one for
0:45 each directional beam and combines them
0:47 to find the most likely point in the map
0:50 where the device is located
0:52 so two terms of importance are ple and
0:55 intercept
0:56 ple is the path loss exponent Mist uses
1:00 this formula to determine the expected
1:02 signal strength at various locations on
1:04 a map based on a gain at that direction
1:07 from the antenna
1:09 taking into account orientation and
1:12 ceiling height
1:13 The Intercept is another constant that
1:15 must be derived it is much like The
1:18 Intercept of a line and indicates the
1:20 expected power at one meter from the
1:22 antenna
1:24 individual RF environments and even
1:27 device types have different optimal ple
1:30 and intercept values this ensures the RF
1:33 model adapts to the RF environment as it
1:36 changes and accounts for the differences
1:37 between mobile device types providing a
1:40 consistent user experience
1:42 the essential element behind miss
1:44 machine learning is to seek the maximum
1:47 agreement between the results of
1:49 increasingly many location estimates
1:51 from that construct individualized path
1:55 loss formulas
1:56 as the machine learning algorithm begins
1:59 it's using the default plf for a device
2:02 type meaning an iPad an iPhone 6 an
2:05 iPhone 6s
2:07 machine learning is running continuously
2:10 the only time you would see an AP at
2:13 zero learning is when it was just added
2:15 to the map or something has been changed
2:18 in its configuration regarding ceiling
2:20 height orientation Etc
2:23 the thing to look for here is to make
2:25 sure all of your APS are learning to
2:27 reach level one usually only takes a
2:30 couple minutes for a device and that's
2:32 the most important level because 95
2:34 percent of the work is done reaching
2:37 level one learning after that the
2:39 algorithm is looking for new unique data
2:42 points
2:43 we have created this visual to help you
2:45 quickly understand the status of
2:47 location machine learning
2:49 if an AP is not learned on a device it
2:52 will be gray when all the devices are
2:54 learned they will show Green
2:56 so what is going on here using the show
2:59 all button you can see the raw data and
3:01 note you can also choose to expose just
3:04 a single device
3:06 what the UI has done
3:08 is to add up the ple and intercept then
3:11 a medium value is derived per device and
3:13 per AP of variance from that median is
3:16 shown
3:17 in a normal environment we would expect
3:19 this variance not to be more than one or
3:22 two if it is three or four the tile will
3:25 show yellow and more than five it will
3:27 be red
3:28 this is to alert you to take a look at
3:30 why this AP may be so different from the
3:33 others
3:34 so in this case this is what I would
3:37 expect
3:38 if you saw a five or a six something
3:41 that's kind of radically different from
3:42 the others immediately I would go look
3:44 at the floor plan and see if that AP is
3:47 in a strange spot that would justify it
3:50 having a much different plf than the
3:52 rest of the aps
3:58 foreign