AIDC Now: Backend AI Training Data Centers
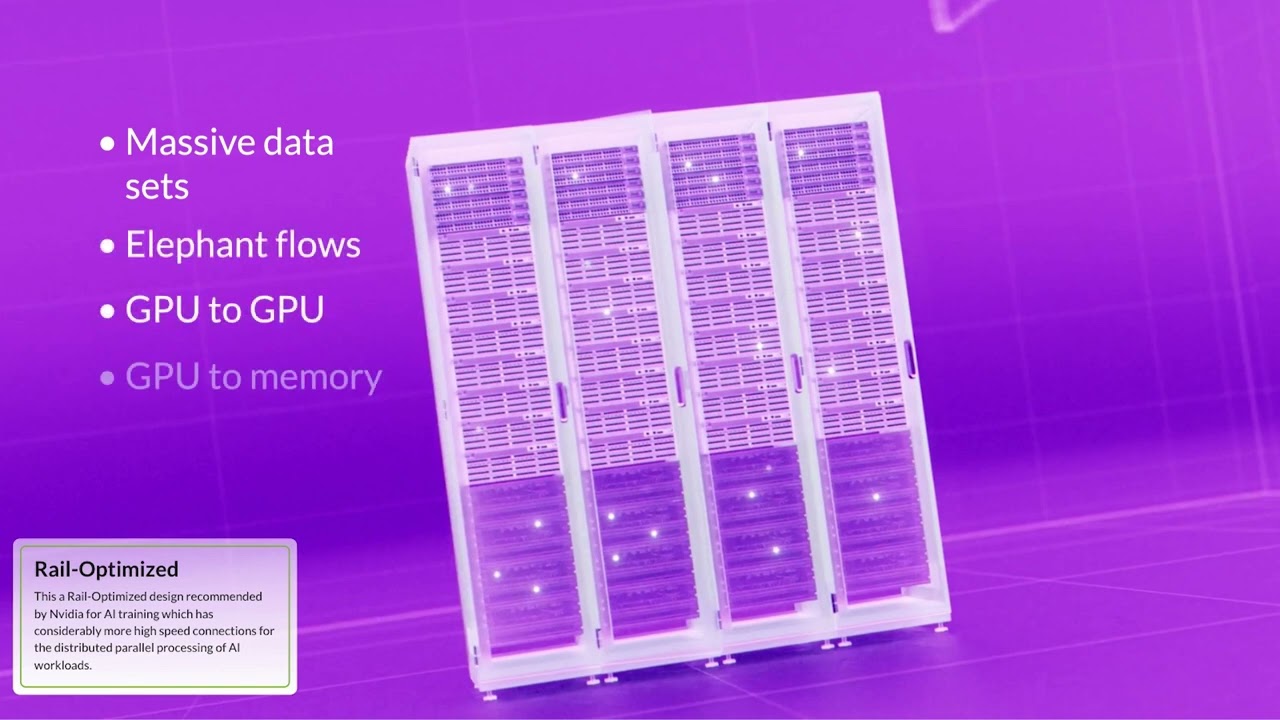
AIDC Now: Backend AI Training Data Centers
In this AIDC Now demo, we’ll share how quick and easy it is to configure a backend data center for AI training with JVDs specifically designed for rail-optimized deployments. Rail-optimized designs maximize performance and efficiency, while reducing network costs.
You’ll learn
How Apstra helps automate rail-optimized designs
Who is this for?
Experience More
Transcript
0:00 With this demo, I'm going to share how our representative customer
0:04 A1 Arcade, can quickly and easily build a back end A.I.
0:08 training data center.
0:10 This DC is made of racks built with two spines, eight leafs and 16
0:14 GPU servers for a total of 128 GPUs per rack.
0:19 Apstra allows us to drill down for a closer look at the network template,
0:23 which in this case is a relatively small data center of 512 GPUs.
0:28 Drilling down further, we can see the actual data center
0:31 design and look at more details of the links and nodes.
0:34 As you can see, this particular network template is
0:37 made up of four of the 128 GPU racks.
0:41 By zooming in on one of these racks, it becomes clear
0:44 that this isn't a normal clos fabric.
0:46 So let's take a second to talk about the fabric design.
0:49 This is a rail optimized fabric introduced by Nvidia, which provides a dense
0:54 number of links and the capacity to handle massive datasets, elephant flows,
0:59 GPU to GPU communications
1:02 GPU-to-memory and GPU-to-storage traffic.
1:05 So what does the training process look like in this scenario?
1:09 Training requires many cycles
1:11 or epochs to train an A.I. model.
1:13 Data sets are chopped up into smaller flows, which are distributed
1:17 across a network fabric to GPUs, where parallel processing shortens
1:21 the compute cycles.
1:23 That data is again sent across the fabric
1:26 where it is combined before another epic can be run.
1:29 This is where network performance becomes so critical.
1:32 It's your key in reducing tail latency and shortening the job completion time.
1:37 So while your network is the smallest investment in an
1:40 AI data center, it's also the most critical to A.I.
1:43 training and GPU efficiency. Do it right.
1:46 Everything works together.
1:47 Do it wrong, and you waste vast amounts of time and money.
1:52 To optimize GPU efficiency, increase speed and minimize cost.
1:56 We use these rail optimized designs along with protocols like ROCEv2
2:01 to provide one hop connectivity between GPUs.
2:04 In this design, GPU1 from each server
2:07 is connected to leaf switch 1, which is called a rail.
2:11 GPU2 from each server is connected to leaf switch 2 and so on.
2:15 Typically across eight switches something Juniper refers to as a stripe.
2:20 As shown in our Apstra overview demo,
2:22 we can simplify the implementation of these fabrics by leveraging JVDs
2:27 that are specifically designed for rail optimized deployments.