AIOps in Networking
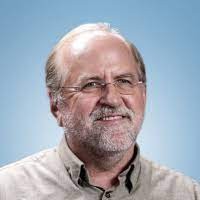
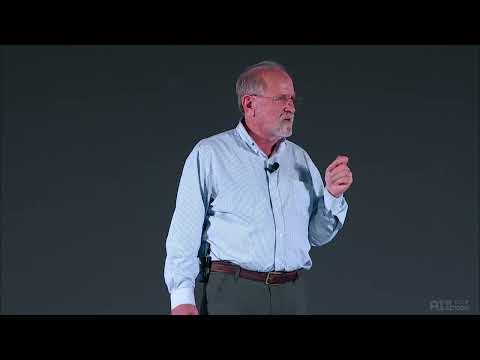
At AI in Action, Chief AI Officer, Bob Friday, highlights the journey to an AI-driven network, key components of our Virtual Network Assistant, Marvis and the future of network automation.
0:49 AI Reality vs. Hype
2:34 Our Mission & Managing Client-to-Cloud
5:38 The Journey to an AI-Driven Network
8:40 The Key Components of Marvis
10:10 The Evolution of Automation
12:32 AIOps & Organizational Structure
14:51 Continuous User Experience Learning
16:00 Marvis Adopting LLM Technology
17:21 Closing Statements
You’ll learn
Key components and the evolution of Marvis
Who is this for?
Host
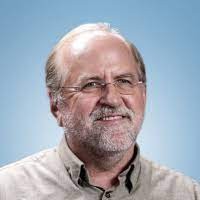
Transcript
0:00 [Music]
0:10 thank you
0:12 hey good afternoon everyone it is great
0:14 to be back in Las Vegas and if we had
0:16 been here 40 years ago when I started my
0:19 career as a wireless networking engineer
0:20 and we had bet one dollar that would end
0:23 my career as a chief AI officer we'd all
0:26 be billionaires going to Tahiti instead
0:29 of here
0:30 Zoe but besides beating the odds you
0:33 know what it really highlights is how
0:35 fast this industry is changing and how
0:38 important AI is becoming
0:41 to the industry you know you look over
0:43 our careers how fast things have changed
0:45 that I'm now a chief a officer at
0:47 Juniper
0:48 uh you know for me personally
0:50 the missed AI Adventure started around
0:53 2011 when I was at Cisco
0:56 that's when I saw Watson play Jeopardy
0:59 right and that's when we saw Watson beat
1:02 those Jeopardy championships and that's
1:04 when I realized you know if they could
1:06 build something that can play Jeopardy
1:09 we should be able to build something
1:11 that plays networking Jeopardy
1:14 right and that's when the adventure
1:16 started and you look at what we've seen
1:19 AI touch all aspects of society right AI
1:23 is going to be on par with the
1:25 Industrial Revolution the Internet it's
1:27 going to touch all aspects of things
1:29 that we work with you know if you look
1:31 what's happening in healthcare AI is
1:33 becoming a necessity for helping
1:35 diagnose cancer right you're going to
1:38 want your doctor to be using AI when
1:40 you're in there visiting him if you look
1:42 what's happening in our farming industry
1:44 right they're building AI now they can
1:47 identify each weed and just apply the
1:49 right pesticide to each weed you know
1:52 this is going to like double or triple
1:54 the production of our agriculture
1:56 industry you know and we look at what
1:58 happening with cars you know I would
2:01 also predict In Our Lifetime it will be
2:03 illegal to drive a car
2:06 you know these autonomous vehicles will
2:07 get so safe
2:09 you know that driving will be outlawed
2:12 and finally you know we also chat gbt
2:14 you know we're starting to see this
2:17 technology transfer become even more
2:19 transformational I personally like to
2:21 thank I send openai Christmas card
2:23 you know they've reduced the number of
2:25 skept cases when I started this five
2:26 years ago
2:27 I would tell you over half the room
2:29 would be AI Skeptics that has gone
2:31 significantly down
2:34 you know in the networking space
2:37 you know when sujay and I left
2:40 Cisco in 2014
2:42 . what we realized was it was going to
2:45 require a new
2:46 architecture to build Cloud AI it was
2:49 going to require a blank sheet of paper
2:52 when we left Cisco to start this
2:54 adventure
2:56 right and when we started the adventure
2:58 we started the adventure with the
2:59 wireless access point it's not because
3:01 we thought the industry needed another
3:03 access point uh be honest most of you
3:05 guys thought we were crazy Tom was one
3:08 of them he's like why are you guys
3:09 starting another wireless access point
3:10 but they didn't realize we really had a
3:13 different Vision the vision was
3:15 basically to solve this Paradigm Shift
3:17 going from managing Network elements to
3:21 one of managing the cloud we still have
3:23 to creep all those Network elements
3:24 green right we still got to make sure
3:27 I'm green but the rule paradigm shift
3:29 and we heard this from you when we were
3:31 there at Cisco was customers were tired
3:34 of controllers from crashing right
3:36 before they were going to put any
3:37 business critical app on that Network
3:39 they wanted those controllers to stop
3:41 crashing they wanted them to transform
3:44 faster right they didn't want to have to
3:46 wait a year for an update you wanted to
3:48 be able to keep up their digital
3:49 transformation they want things to
3:50 happen in the order of weeks but most
3:52 importantly they wanted to make sure
3:54 that that user was going to have a great
3:56 experience when they put that App or
3:58 that application on the network
4:01 and we started that Journey with the
4:03 wireless piece because when we were
4:05 trying to answer the question of why are
4:07 you having a poor internet experience
4:09 the access layer has about 80 percent of
4:12 the information you need to answer that
4:13 question
4:14 and we built those access points to
4:16 basically move Telemetry back to the
4:19 cloud
4:20 and so that's where we started the
4:22 adventure since we've joined Juniper now
4:24 you've seen us basically extend Marvis
4:27 and AI Ops across the wireless AP to the
4:31 switch and to the route you know the AP
4:35 brought all that connectivity
4:36 information
4:37 the router that sd-wan router brings all
4:40 the applications starts to help us to
4:42 answer questions about the applications
4:44 and what we just announced last week a
4:47 week or two ago a Mobility field day is
4:49 something called continuous learning
4:50 it's you know when you're in the data
4:53 science business
4:54 you talk to data scientists label data
4:57 as gold
4:58 it is very hard to get good label data
5:02 you know what we're doing with these
5:03 Cloud applications like zoom and teams
5:06 now is basically getting labeled data
5:08 from that user experience application
5:10 they know when you're having a bad
5:11 experience
5:12 you know and with that label data now we
5:15 can join it with network feature data
5:17 and actually build models that can
5:19 accurately predict your Zoom team's
5:22 performance
5:23 and once you can do that
5:25 you can now start to explain why you're
5:27 having bad teams so that is where AI
5:31 deep learning is starting to transfer
5:32 transform what we're doing in the
5:34 networking space
5:38 now as I said we started the journey
5:40 with the data you know if you look at
5:42 the access point
5:43 that I built 20 years ago at airspace
5:46 that access point was sending data back
5:49 to the controller every minute every two
5:51 minutes
5:52 it was sending you back synchronous you
5:55 know the reason we built the access
5:56 point was because I really did not trust
5:58 Cisco in Aruba they give me the data I
6:02 needed to answer that question
6:04 so we look at the data side that is
6:07 where we're starting to get user state
6:09 in addition to that synchronous data
6:11 we're starting to get asynchronous data
6:13 right every time that user State changes
6:16 I want that access point to send me back
6:18 to State I want to know that user State
6:20 when it changes
6:22 and the next big part of this puzzle was
6:24 really what we call these AI driven
6:26 perimeters
6:27 was what we found out
6:30 promote from you
6:31 AI aside was you wanted the cloud just
6:35 getting the data to the cloud
6:37 and that took us about a year you know
6:40 Randy got the data built you know got
6:43 the network built and then we had to
6:44 spend a year trying to figure out why
6:46 the support team was still sshing into
6:48 radios to solve problems
6:51 right
6:52 you because when you do this Cloud a
6:54 you've got to get that data back to
6:55 compute storage you gotta bring the data
6:58 to where the compute storage is where
6:59 you have unlimited compute storage
7:01 and the good thing about AWS and Google
7:03 we have unlimited compute storage
7:05 they only have to worry about is my bill
7:07 I get a bill from those guys every month
7:09 and I still that still limits you to
7:11 some extent but once you're there
7:12 there's no limit on what you can do with
7:14 the data and you guys appreciate that
7:16 because once you get the data there we
7:18 all have access to the same data
7:20 everyone in the team has access to it
7:22 the next big piece of the puzzle was
7:24 really around the data science
7:26 and it's really not around the
7:27 algorithms this is around the team
7:30 right and the big thing we did inside of
7:32 a Miss is really get our data science
7:35 team next to our customer support team
7:39 because that support team represents our
7:41 customer
7:42 and then the fourth thing the thing I'm
7:44 most proud of this is something uh
7:46 four or five years ago when I started
7:48 the whole conversational interface for
7:49 severe you know there was a big argument
7:51 whether or not we really need
7:53 conversational interfaces
7:54 you know and what I firmly believed was
7:56 in the industry we all started our
7:58 careers with CLI we're all CLI jockeys
8:00 20 years ago very proud of it and then
8:03 we slowly went to dashboards and it
8:05 makes life a little bit simpler the next
8:07 big transmission transition in the user
8:09 interface is going to be around these uh
8:12 these llms in CI you know you're going
8:15 to be talking to your network
8:17 like we see on Star Trek that is going
8:20 to be the next big trans is going to
8:21 make life easier for RIT to actually
8:23 start troubleshooting networks and
8:25 finally Studio's favorite is actions at
8:27 the end of the day
8:28 ah I don't care I don't need to know why
8:31 there's a problem I just need you to fix
8:33 it if you know why there's a problem
8:34 just go ahead and fix it and not bother
8:36 me anymore
8:40 and then finally if you look at the key
8:42 components of Marvis
8:44 you know we started with
8:47 the VNA that was our conversational
8:49 interface that's where we started the
8:51 journey four years ago you saw that with
8:54 nlu
8:56 you know what you're going to see us
8:57 start doing is start basically
8:59 integrating llm into that conversational
9:01 interface we've always had the natural
9:04 language understanding piece of the
9:05 puzzle the next piece of puzzle is to
9:08 start to generate answers that sounds
9:10 like a human and that's what llm is
9:12 trying to bring to the party is that
9:14 natural language generation part that
9:16 starts to bring your network to life
9:18 the action framework is a self-driving
9:20 piece this is the piece where you want
9:23 your network to start taking actions and
9:25 this is really going to become on an
9:27 element of trust
9:29 right this is the piece of the puzzle
9:30 where
9:31 you have to we have to earn your trust
9:34 right we have to earn your trust as an
9:36 AI assistant and I always tell people I
9:39 don't care if you're a virtual assistant
9:40 or a real person most I.T people are not
9:43 going to let you touch the knobs on
9:44 their Network
9:46 until you've earned their trust somehow
9:48 they've got you've got to make sure that
9:49 you trust that virtual assistant to
9:52 actually twist the knobs or make it
9:53 change your network and the third piece
9:55 that we're adding and this is a new
9:56 fundamental Lake in the marvelous
9:58 adventure is this continuous learning
10:00 feedback
10:02 this is where we're starting to bring
10:03 label data back from your Cloud apps
10:10 now
10:12 people ask me to AI ml for me AI is not
10:16 an algorithm AI is a concept
10:19 right and it's really the next step in
10:22 the evolution of automation
10:24 you know what we're doing with AI is
10:26 really Building Solutions that can do
10:29 tasks on par with human domain experts
10:33 right we're building solutions that
10:34 typically require cognitive skills you
10:38 know we've all built automation of
10:39 basically automate some deployment
10:43 configuration those are very basic
10:45 scripts the next step is really doing
10:48 something that typically would require a
10:50 human to do
10:52 and if you look what's going on under
10:53 the hood of Marvis
10:55 I usually break it into two sets of
10:57 algorithms you know we have kind of
10:59 these regression ml this is what we've
11:01 been doing for decades they've been
11:02 around for decades you know what really
11:04 changed in 2014 was the addition of
11:08 these deep learning networks you know
11:10 and interestingly if you look at Google
11:14 you know you look at the Google search
11:16 statistics
11:17 on AI ml it is in 2014
11:22 when AI went from Mostly a research
11:25 topic
11:27 to a reality 2014 is when we saw kind of
11:30 that perfect storm of compute storage
11:33 low-cost cloud storage again good we saw
11:36 tensorflow and all the tool sets needed
11:38 to build these things become real
11:40 you know that was the year when things
11:43 started to come together for AI
11:45 and these deep learning algorithms these
11:47 are the ones they're disrupting the
11:50 industries
11:51 chat DBT that was Transformer right
11:54 that's all the Transformer technology
11:55 that came out in 2017 from Google
11:58 lstm or the Deep learning algorithms
12:01 that are allowing us to do anomaly
12:02 detection now with very low false
12:04 positives kind of a very basic
12:06 networking capability we all talk about
12:08 that I've never really achieved because
12:10 there's always too much noise coming out
12:13 of those things waking you up at three
12:14 o'clock in the morning
12:15 and then what we're talking about now is
12:17 shappy for those who have not heard
12:19 about shapley this is basically a
12:21 technique that lets you make these AI
12:24 models explainable like you understand
12:25 what features are really driving a
12:28 prediction
12:35 and I think the other quick thing you're
12:37 going to hear Randy come up talk a
12:38 little bit about this more
12:39 was in addition to having to build a
12:43 whole new Cloud infrastructure
12:45 right that had the microservices
12:48 architecture had the pipelines when we
12:52 wanted to build real-time data
12:54 processing
12:55 and Susie will quietly reminded me Bob
12:58 when we started this we really weren't
13:00 talking about AI
13:02 back then we were really talking about
13:03 trying to build an architecture that
13:06 could do day two operations and process
13:08 data in real time
13:10 now it turns out that is the foundation
13:12 you need if you want to do AI you have
13:14 to build Cloud infrastructure they can
13:16 process data in real time
13:18 but more importantly
13:19 you know
13:21 the need for the blank sheet of paper
13:22 was around organization
13:25 right in addition to having to get all
13:27 this technical stuff done and built
13:30 we had to basically get the domain the
13:32 data science team
13:33 tied to the
13:36 support team those are your domain
13:38 experts
13:39 you know and if you look at our Cloud
13:42 support team what you notice is the Miss
13:45 support team is represents you
13:49 right we all have access to the same
13:51 data Cloud the customer the support team
13:54 the data science team you know and the
13:57 data science team's Mission and life is
14:00 to keep the support team happy
14:02 the fewer tickets that that support team
14:04 sees
14:05 is a few tickets that you guys are
14:07 sending our way
14:09 right and that is the key and I will
14:11 tell people that you know if you talk to
14:13 an infrastructure vendor if their
14:15 support team is not using their own AI
14:18 Ops tool they have not started the
14:20 journey
14:21 because this journey starts with making
14:23 sure your data science and your domain
14:25 experts are tied to the hip and that's
14:27 another thing that's very hard to do in
14:29 a large organization like Cisco and
14:31 Aruba it's hard to organize actually
14:32 change those type of things and I always
14:34 tell people
14:35 if you want to look for architecturals
14:37 changed in an industry
14:39 if you see Cisco try to get three
14:42 different bus to work together
14:44 you know something's up that is a sign
14:46 that something's changing
14:48 so keep an eye out for that
14:51 now this is what I've been talking about
14:53 I'll go a little bit deeper on this if
14:55 you look what's fundamentally Happening
14:57 Here
14:58 we're not getting label data
15:00 from your zoom and teams we're taking
15:04 that label data we're joining it to the
15:07 network features
15:09 and now we're building models that can
15:11 predict the audio latency the video
15:14 latency and really the performance
15:18 of your Zoom teams experience
15:22 now once you have a model that can
15:24 actually predict something accurately
15:26 the magical thing called the shapley
15:28 is now we can explain to you what
15:31 feature
15:32 is causing that performance so when you
15:35 have a latency if the average latency is
15:37 100 and your CEO has an average latency
15:41 of 150
15:43 we can now explain you know is the
15:45 wireless is it the client or is the WAN
15:48 who actually contributed to that latency
15:51 problem
15:52 so that's the the power of these deep
15:54 learning models that are going to be
15:56 transforming how we solve and
15:58 troubleshoot and manage networks going
15:59 forward
16:01 the other big piece of this is really
16:03 around natural language what we've done
16:04 to date you know in your current Marvis
16:06 interface
16:07 is we have Ross and nlu there so we do a
16:10 breaker job of understanding your intent
16:12 but we've never done a really good job
16:14 of basically giving you an answer that
16:16 sounds like a human wrote it you know
16:18 and that's going to be our first step on
16:20 the journey to L and we're going to
16:21 integrate lym into Marvis starting with
16:24 knowledge-based questions
16:26 now our goal is to get Marvis to the
16:28 point that it can actually pass
16:30 a networking certification test so
16:33 you'll basically have a network
16:34 certified engineer by your side helping
16:37 you troubleshoot things
16:40 the next step in this adventure
16:43 is to put this on top of your database
16:47 you know where we actually translate
16:48 text SQL
16:51 so you can now start talking to your
16:53 network like you would talk to a Star
16:55 Trek computer you know so this is my
16:57 other thing you know we all all the star
16:59 Tommy Star Trek fans that we have in the
17:01 crowd
17:01 you know okay talking computers that
17:05 technology is about to become real
17:07 we are down to the teleporter the only
17:09 thing left will be getting this tell a
17:11 problem solving talking computer is
17:13 within our lifetime within my lifetime
17:15 maybe let me shortly shortly here
17:21 okay with that I'm all invite said
17:23 you're back up
17:24 partner crime is going to take you
17:26 through reality and get us off some
17:27 PowerPoint awesome thank you Bob thank
17:29 you very much
17:32[Music]