The Q&AI: AI Agents & Cybersecurity - A Conversation with Claudionor Coelho
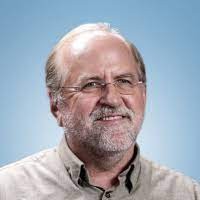
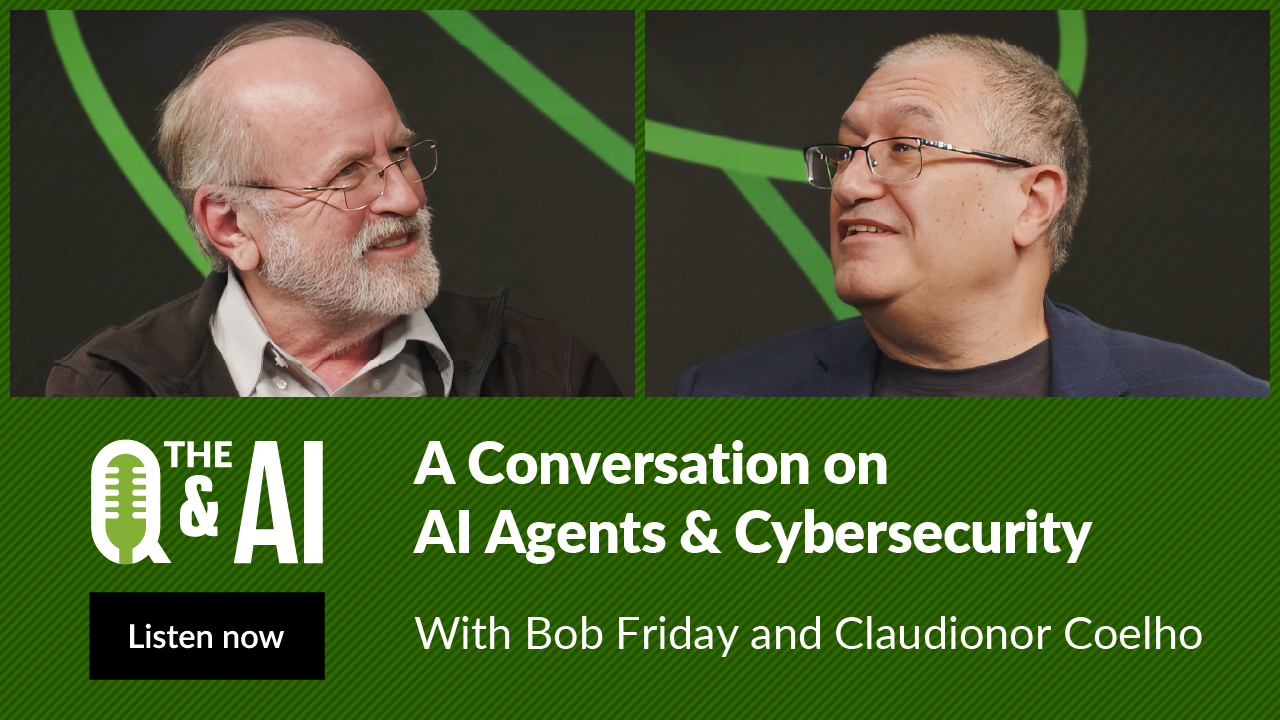
The Q&AI: AI Agents & Cybersecurity - A Conversation with Claudionor Coelho
In this episode of The Q&AI Podcast, Bob sits down with Claudionor Coelho, Chief AI Officer at Zscaler, to unravel the complexities and transformative potential of artificial intelligence in today's rapidly evolving technological landscape. They explore Claudionor's unique journey from semiconductor research to leading AI strategy in cybersecurity, providing insights into the crucial role of a Chief AI Officer and the imperative of securing AI in an interconnected world. Tune in as Bob and Claudionor discuss the shift from LLMs to AI agents, the challenges of hallucination, and the future of AI in various industries.
You’ll learn
The critical importance of secure AI: Addressing vulnerabilities and ensuring responsible AI use in an increasingly connected world
AI's impact on cybersecurity: Leveraging AI for threat detection while securing AI systems themselves
About the shift to AI agents: Exploring the capabilities and implications of multi-agent systems beyond traditional LLMs
Who is this for?
Host
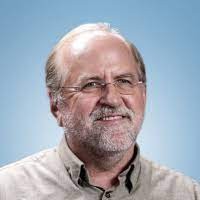
Guest speakers
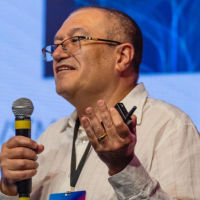
Transcript
Bob: Welcome to another episode of Q&AI. Today I have Claudionor Coelho Chief AI Officer at Zscaler here with me today. And today we're gonna be looking at the role of a Chief AI Officer, and we're gonna be talking a little bit his path to AI. With that, Claudio, welcome. Maybe we start with a little bit for the audience, a little bit about your background and how did you get into AI?
Claudionor: Okay. First of all, thanks a lot for having me here today. And I started my career not in AI. I started in semiconductor and I did my PhD and I worked in several companies before, work on techniques that end up being what people are using to build, AI accelerations right now. And then that's when I made, and I started working on semiconductor and that's when I made my journey to AI when AI started.
And after that, I basically created some techniques in Google that was used by CERN to search for subatomic particles. And then after we got a paper on nature, we were thinking how we could contribute more to society. Then I started going to more deep into AI and into, cybersecurity, and that's when I end up being like a Chief AI Officer.
Bob: Okay, so, so your PhD, what year was this now?
Claudionor: 1990.
Bob: So, this, this is back in the early 90s, you were already doing stuff with AI in some form or fashion. And from what I understand, you got, you're on the cover of Nature.
Claudionor: Yes, there was no, target application for what I did at the time. And it took almost, I joke saying it took me like 30 years. To have a killer application for the techniques that I created in my PhD, which was like when AI started. And the, the work that we did that appeared on the Nature was the result of that work.
Bob: Oh, very interesting. What particle were you chasing down at the time? In the 90s, I'm trying to visualize.
Claudionor: No, there was no particle. We were just creating EDA tools, Electronic Design Automation tools. It was like a very hot topic back in the 90s. To assist like a semiconductor industry by creating tools to the help design chips.
Bob: Yeah, so the other question I always ask my guests is kind of like when did AI go from research to practical reality for you? When was that transition because like you say we've all been working with AI for most of our lives. At some point, if it went from research to reality.
Claudionor: So that's a very interesting question. So, I worked in companies doing formal verification before, and at some point we had the fastest SAT solver in the world and we need to jump ahead of competition.
And then I started using AI to fine tune SAT solvers so that we could even run it faster. And this was back in 2008. And it was very, very funny because, last week there was like the AAAI conference, and there was a tutorial there talking about using AI to speed up solvers, which is something that we did back in 2008.
Bob: Okay, so you think in 2008 something happened in that time frame, because I always tell people when you look at Google, the trends for, search trends for deep learning, machine learning, that's around 2014, we started to see a lot of startups coming into play.
Claudionor: At that time we were not using like a deep learning AI, but actually in 2016 I was working for Synopsys and I was starting to build like what people call now agents and copilots. This is before Transformers Network.
Bob: Now, somewhere along this journey, it sounds like you started the adventure in this silicon formal verification process and then somehow you went to security. You're at Zscaler now. When did that transition happen?
Claudionor: So that transition happened in 2020 and after with our paper had just been accepted for publication at Nature and then you know that you're saying okay.
What do I want to contribute next in my life? And then I said I want to contribute to cyber security because there is like this war with no soldiers that we need to protect infrastructure. We need to protect companies because there are people trying to attack and steal information from people and from companies all the time.
And that's when I ventured to cyber security. Initially, I was working on predictive machine learning like forecasting and trying to do anomaly detection later on copilots. I built in a previous company like a copilot to and then after that, like, I went to semiconductor manufacturing to try to protect using in private clouds.
And finally Zscaler as the Chief AI Officer, basically building the strategy for the company and helping build the product in AI.
Bob: So Zscaler is when you became a Chief AI Officer. They hired you as the Chief AI Officer there?
Claudionor: Yeah, but actually my previous company was Advantest and I was the Chief AI Officer already.
Bob: What year was this now?
Claudionor: Oh, 2022.
Bob: Okay, so 2022. I'm trying to, same time frame. So, maybe, I'm always interested to get other people's perspective. When you think of the Chief AI Officer role, how do you describe it?
Claudionor: That's a very interesting question. So, what happens is that, If you look at corporations right now, and whenever like, CISOs and CEOs or even boards ask me to come and talk to about AI, AI has become very important and strategic for companies.
And they need to create a roadmap on how they're going to start adopting AI. And if they're going to create new business units using AI, they need to figure out how they're going to use AI. And I joke saying, I was talking to a lawyer once saying, even for me, to understand like AI, because there's so many papers, so much going on, it's sometimes it's hard to follow through and make sense of what's going on.
And I imagine for people who have no technical background, how they're going to follow through and figure out how to create a strategy for a company using AI.
Bob: Now, maybe I kind of play the Chief AI Officer, CTO role. And I always tell people, Chief AI Officer is kind of a horizon one trying to organize.
The media, internal, external efforts, and CTO is more Horizon 2. How do you kind of blend together the Horizon 1 aspect of Chief AI Officer role with the Horizon 2 CTO aspect of it?
Claudionor: So, there are several aspects, at least in my role. One is operational, that I help like our business units in developing new products using AI, special generative AI.
And the other one is, is external facing that I go and talk to companies saying how they're going to use AI from now on, and when they have to use AI, they need to secure AI. And that's when I bring security because people don't realize that a lot of stuff can go wrong when they deploy AI or AI agents.
Bob: So, I think, I think you touched a little bit upon, there's kind of this customer facing component of, trying to integrate AI into your product. And there's this broader internal effort. Where do you think you spend most of your time? Do you think you spend most of your time on the external, customer facing product side? Or more about helping, Zscaler and the company become more efficient in leveraging AI?
Claudionor: I think it's probably like half and half. Because whenever I go to talk to CEOs and to C- level executives about what AI is going to bring to them, then I have to introduce that they need to secure AI. And it's a very important thing people need to realize.
That they're not preparing to secure AI. They think it's just connecting to LLMs initially, but even if you think about, HR department, and they decide, let's say, terminate Claudionor, and they put, they write a letter of termination, they put the letter on ChatGPT to improve the letter, they may have violated some privacy laws doing that, and they need to make sure that they need to understand what may go wrong. As we go from, LLMs to AI agents, things can get much worse.
Bob: Now, any words of wisdom for the audience out there? Because I think, I think every company is struggling with the, how do they control all this AI activity going across their company? For me, it reminds me of the early days of Wi-Fi when, IT said, hey, bring that access point in, we're going to fire you. So, I think we're in that same boat, right? And, how does IT control, how do you control all the AI tools coming into a company going?
Claudionor: So, you can control when you're basically trying to interface with LLMs, but right now there are several applications that you may be connecting to, and those applications may be using LLMs underneath.
But right now we are at the turning point in society that we're shifting from LLMs to using real AI agents and AI multi agent systems are systems that employs algorithms and employs LLMs and several LLMs and they exchange information among them. And I had a quote from someone from Amazon before and he was saying, imagine that right now when you're going to be buying like a stock in the stock market.
The two entities that dictates the prices of the stock price, they are robots, that they, they negotiate the price and that's the price that everyone buys. So, we are going to soon start going to society where the AI agents are going to be talking and negotiating things among themselves. And then we are just the result.
Suppose that we are interacting here in the future, and I have my AI agent, you have your AI agent, and the two AI agents are exchanging information about our conversation here today.
Bob: Well, now this is a topic dear to my heart, this Gen AI agents. Because I looked at it, it's like, this is a new software paradigm, right? Programming paradigm. I mean, we were basically using non-linear, non-deterministic programming agents to solve problems. We talked a little bit about this earlier. It's like, is this transformational or just another programming paradigm that we need to master?
Claudionor: I think this is like completely transformational because usually when we talk about algorithms, algorithms has, if you provide this input, it's going to give you this output. But you have a little bit, you want to have a little bit of adaptability to the algorithms. And at the same time, whenever you're going to communicate to entities, we need a protocol to communicate. A lot of people have this, discuss how they're going to create protocols. Computer network is just a proto started as proto communication protocols.
How to communicate between two entities. But reality is, English is a, is a very good language. And to communicate between two entities. And if you have two entities that communicates in English, and internally they use algorithms to run some stuff, there you have like a multi agent system running.
Bob: So maybe a little bit, going through this myself right now, if I look at all the little startups out there, if I look at this non-deterministic, non-linear programming technique, it seems like the whole software QA benchmarking is changing, right?
This is not a, we're not creating deterministic programs like we've done in the past. Any tools or words of wisdom for people who are starting down the path of the Gen AI agent?
Claudionor: First of all, I usually tell people they should pay attention to data. Data is much more important than you imagine. I publish like a blog two years ago called the world that needs more librarians and but that blog was referring that you need people to organize the data on as a fun fact, a lot of people who gave like on my LinkedIn page when I published that was basically saying, Oh, yes, we need more teachers and librarians at schools. Apparently, they did not read my whole blog, they just read the title.
Bob: Yeah, but like I told you, I make a barrel of wine, I always tell people, great wine starts with great grapes, AI has the data, so it's like getting your arms around the data is usually the first step on the journey to mastering AI.
Claudionor: And the first is data, second, when you're talking about LLMs and agents, we have to understand that people will not use systems if they have hallucinations, so we need to make sure that those systems will not hallucinate. And the third is protection and, and because we don't want information to be exchanged in the wrong way or toxicity or, or those systems to exchange information that should not be exchanged.
Bob: Yeah, so the hallucination is always an interesting discussion right now. And, I have friends right now that basically say, Hey, all this Gen AI stuff, just stochastic parrots. These are just things we've trained to, speak like a parrot and stuff. Now my reply usually is back to you. You have kids, new employees.
How often do your kids and new employees do something that makes no sense? And you go, hey, are they hallucinating? What's the difference between that and my large language model doing something that makes no sense and my, my kid who does something that makes no sense?
Claudionor: So, I'm going to quote an Uber driver that was taking me to the airport like a few months ago. And he was telling me that he does, he did not like the Tesla full self-driving and, and my wife has a Tesla and I love the full self-driving. But he was explaining to me that whenever he turns on the full self-driving, he expects, because it's an autonomous system, to be flawless. And whenever he sees the flaws, he does not see consistence in the flaws.
So that's a problem with hallucination. You do not see consistence in the hallucination. Sometimes it hallucinates on something very, very easy. Sometimes it makes some answers that it's very complicated and the lack of consistency is what worries me.
Bob: Well, now you pick up Waymo Uber. So, have you been to Phoenix lately?
Claudionor: No.
Bob: Okay. So, if you go to Phoenix, it looks like something out of a sci-fi movie. We have self-driving Waymo Ubers pulling up left and right. I was in them. Now, do you think, I mean, self-driving Waymo Uber, is that AI?
Claudionor: It is AI, and I like to use Russell Stewart's definition. He gave an interview to World Economic Forum a few years ago where he said the definition of AI includes nest thermostats.
Which is a rule-based system, okay? So, rule-based system, it includes AI. But AI also has Predictive AI and it has Generative AI. So, we should be thinking about that we should use the right AI or the right tool for the right task. And what I've seen, right now is that a lot of people over engineering systems saying everything should go to generative AI. Even when something very simple could be used.
Bob: Yeah, because I mean, I think I always tell people, even here at Juniper, it's like, not everything requires fancy math. Although I would, I would have said that the machine language stuff we've done for the last 30, 40 years, that the real differentiation is these large models trained on large data sets. That seems to be driving the next generation of AI that we're seeing right now.
Claudionor: It is, and I have to tell you that, I was at Google and I was a keynote speaker at a VLSI conference in Peru back in 2019. And then I was telling them at the time, since I was in Peru, in Cusco, I did the math about training transformer networks.
Remember transformer was like 200 and13 million parameters and training 40 of those models back then was equivalent of to the fires in the Amazon Forest for one day. Yeah. And the models that we're talking now, they're like 1000 times bigger.
Bob: Well, that's what I usually tell them. So back in the 80s, when I did my master's, I trained a little neural network to decode radio signals and stuff. And, the only difference is thousands of weights. Now we're up to five hundred million weights, going forward.
Claudionor: But remember, you're talking about the amount of energy that you're talking about training those very large language models. Even some people are starting saying that for some applications maybe you should start using like smaller models and fine-tuned models.
And what DeepSeek has shown us is that you can distill those large language models into much smaller models and you can use those smaller models. Actually, there is also a research by Berkeley that was released around the same time as DeepSeek that basically says that you could distill models into much smaller models and still get a lot of them.
Bob: Yeah, I think we're going to see that with these, these Gen AI agent graphs, right? Each, depending on what you're trying to solve, each agent will be optimized for a different model, depending on what you're trying to deal with. Now, maybe, touching upon the Waymo, Uber hallucination discussion, I mean, do you think Waymo and Uber or self-driving cars are going to be safer than Human driving cars. I mean, they don't drink. They don't get distracted.
Claudionor: I'm going to quote a presentation I did at Purdue University last year, and I got these numbers from Tesla, by the way. So, I'm quoting Tesla on this. But Tesla was saying that, on average, Tesla cars engage in an accident in terms of per mile driven, like 10x more miles driven before engaging into an accident, as opposed to like a normal person driving.
So, it's, I think it was like a 1000 miles before a person engaged into like on average on an accident. But in like in terms of Tesla was like close to 8,000, 10,000 miles.
Bob: I think we're going to find out these, AI are going to become on par with humans. They're safer and better on some, some topics and everything.
Claudionor: I think they are safer right now. The difference is that when they make a mistake, their mistakes are very, you say, Oh, why did they make this mistake? For example, I was basically visiting my daughter in Santa Barbara and I was driving at 3 a.m. I basically turned on the self-driving car on Tesla and there was one point on 101 South that there was a bridge and there was like an exit in front, but the bridge followed to the right. And it went straight and I had to take the car and continue and say that's a mistake a person would not make.
Bob: I don't quite take my hands off my Tesla quite yet.
Claudionor: No, I did not take the hands off the Tesla.
Bob: So maybe I touch a little bit, giving you a Zscaler security. So, here at Juniper we have a saying, AI for networking, networking for AI. We were talking a little earlier, it sounds like, in your space, it's AI for security and security for AI. Maybe give the audience, the difference.
Claudionor: So, AI for security, it means, I'll just give like a public number from the scale we process half a trillion transactions per day. There is no way you would be able to process that number of transactions if you're not using AI from inside of the onset of the company. So, we use AI to analyze massive amounts of data everywhere. And that's what I call AI for security.
Whenever I go to customers to talk about AI and talk about strategies on how to use AI, We have to understand we need to secure the AI.We have to make sure that whoever is using AI is not doing something that he or she should not be doing.
Bob: So maybe one final question, typically is like, AI, are we ever going to see singularities in our time? Are we going to start to see these AI actually? Surpass humans and reasoning in solving problem solving skills.
Claudionor: So, I am a truly believer on AI agents and two years ago I was in the World Economic Forum meeting. Everyone was scared about the the ChatGPT and OpenAI. And I was basically telling people, like with AI. We can do a lot of damage. Damage I mean, you can solve a lot of real time problems without having to wait until we reach AGI. And I think with agents are going to they overcome very easily the limitations of LLMs. And the example that I want to give you is that as soon as DeepSeek was released, my daughter was having, like, some problem with, like, a very hard integral. And she asked me, like, can you help me figure out how to solve this integral?
And then I tried DeepSeek on it. DeepSeek in five minutes running on my laptop did not give me an answer. Later on, some people said, if I run the very large DeepSeek 600 billion parameters, after ten minutes, it would give an answer. However, I have Mathematica installed in in my laptop. Mathematica solved the same problem in one second.
So, it's like a 60,000 percent Speed up on a very simple problem and that's usually what I tell people this shows you that you should use the right tool for the right task.
Bob: Okay, so you don't think we're gonna see any AI passing the Turing test anytime soon.
Claudionor: The problem that I have is that LLMs, the current architecture of LLMs They are a forward pass technique. There are several problems in real life, like testing software and finding bugs in software, that you need backtracking. You need to set solvers or SMT solvers that can do that.
So, I can see that for some tasks, if you combine a symbolic engine with like LLM, then you can solve much bigger types of problems.But the current architecture of the LLM, which only uses forward view of the text. It has its own limitations.
Bob: Well, Claudio, I want to thank you for joining us on this episode. I could spend hours with you over a glass of wine, totally entertaining. I want to thank the audience for joining it and look forward to seeing you on the next episode of Q&AI. And please check out past episodes on Spotify.
Claudionor: And thanks a lot for the invitation on the glass of wine. And thanks a lot for having me here.