RDMA Over Converged Ethernet Version 2 for AI Data Centers
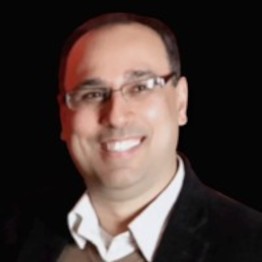
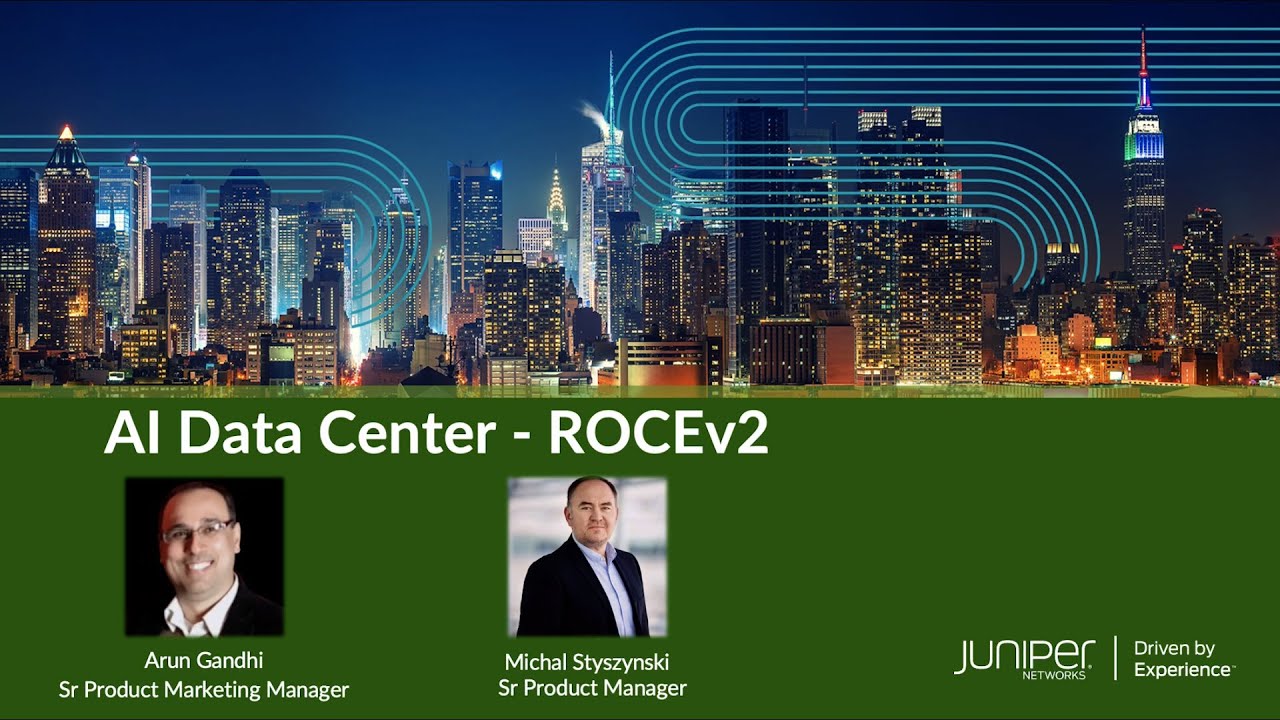
Could ROCE supplant InfiniBand as a go-to solution for AI and ML networking?
AI and ML applications are growing at a fast pace in data centers. Ethernet-based networks are gaining interest as an alternative to InfiniBand in AI data center networking. RDMA over Converged Ethernet version 2 (ROCEv2) encapsulates RDMA/RC protocol packets within UDP packets for transport over Ethernet networks. Juniper’s Arun Gandhi and Michal Styszynski discuss the data transfer protocols and congestion considerations for AI workloads.
You’ll learn
How the value of ROCEv2 has increased with the rapid rise of AI and ML models that require lots of parallel processing capacity at scale
How ROCEv2 components are coordinated inside the data center fabric
Who is this for?
Host
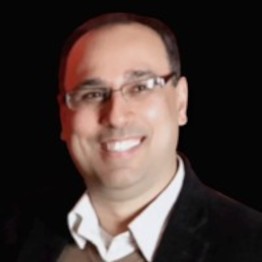
Guest speakers
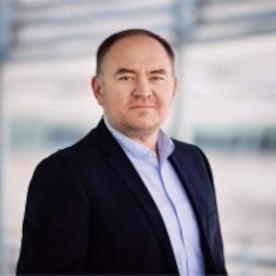
Experience More
Transcript
0:08 hi everyone welcome to another season of
0:10 the video series to learn about cutting
0:13 a Technologies for your data center in
0:16 the last series Almost 2 years ago we
0:19 discussed bgp unnumbered RDMA over
0:22 converge eanet version 2 otherwise known
0:26 as Rocky
0:27 V2 and uh I Fabrics from Modern data
0:32 centers well as you know recent advances
0:35 in generative AI have captured the
0:37 imagination of hundreds of millions of
0:40 people around the world data centers are
0:42 the engines behind Ai and data center
0:45 networks play a critical role in
0:47 interconnecting and maximizing the
0:50 utilization of costly GPU servers that
0:54 perform the compute intensive processing
0:57 in an AI training Data Center
1:00 today I'm joined by my special guest and
1:03 a good friend Michael cinski Michael
1:07 always a pleasure to sit down with you
1:09 and discuss cuttingedge technological
1:12 advances in the data center spaceon hi
1:15 everyone uh thanks for having me AR
1:18 today you are absolutely to the point
1:20 Aruna uh as a matter of fact you know
1:23 after two years uh we see that the
1:25 Technologies we discussed uh just uh
1:28 after the covid ERA with sending we
1:31 realized that in the last two years the
1:33 technology of RDMA over converge
1:36 ethernet just exploded in terms of
1:39 popularity while we we we can say that
1:42 the Baseline of the technology stays the
1:44 same actually the way we use it uh
1:47 changed a little bit and that's of
1:49 course in the context of uh of the
1:52 explosion of the popularity of the chat
1:54 GPT Louge language models artificial
1:57 intelligence and machine learning so
1:59 when building these infrastructures we
2:01 need a a lot of parallel processing
2:04 capacity at scale so it means we are
2:07 using additional components inside the
2:09 server such as the gpus which are
2:12 accelerating the way we can treat the
2:14 data at the same time in parallel right
2:17 so instead of using the CPU approach
2:19 which is a serialized approach of
2:21 processing data using the gpus which are
2:24 in the same server we can actually
2:27 process the data in parallel at the same
2:29 time and deliver the outcomes to the uh
2:31 to the user quickly on time right so in
2:34 order to exchange the data and have the
2:37 cycles of the of the data processing uh
2:41 to occure on different servers we need a
2:44 technology to actually synchronize the
2:45 datas between the servers and one of
2:47 these technology is in fact the rocky V2
2:49 we discussed over two years ago now uh
2:52 so did anything change like you wanted
2:55 to make to to make sure that we are
2:57 actually up to date about this
2:58 technology so there are some components
3:00 that change uh popularity of the
3:02 technology increased but there is a
3:05 reason for that also is because simply
3:07 the technology is offering much more
3:09 better resiliency comparing to for
3:12 example a centralized model of infin
3:14 band where there is also a controller
3:16 which actually uh you know gives the
3:19 access to uh to the resources of the
3:21 fabric in case of Internet IP Fabrics
3:24 where we leverage we transport the infin
3:26 band uh across the the fabric we have a
3:29 fully distributed architectural model so
3:32 that's that's one of the advantage so
3:34 what changed comparing to what used to
3:36 be is for example the scale actually
3:39 requirements are much bigger now right
3:41 so uh we we have for example the
3:44 concepts of uh of running dedicated
3:46 rocket to uh networks in the back end of
3:49 the of the AIML clusters and that's
3:52 where also the technology is use in the
3:54 leaf spine super spine type of
3:56 deployment but the over subscription
3:59 ratio for example example is one: one
4:01 comparing to what it used to be
4:02 traditionally in the data center
4:04 deployments where we had like one to
4:06 three one to2 sometimes even one to six
4:09 so in this case even if the over
4:11 subscription ratios from Leaf to spine
4:13 goes to one to one in some situations
4:16 still rocky V2 uh will play a
4:19 significant role it's also the case
4:21 whenever we have a a rail optimized
4:25 deployment uh where actually we connect
4:28 the gpus on the same switch but then we
4:30 have interconnectivity between different
4:33 Stripes of the leaf devices to go
4:35 through the spin that's where also the
4:37 strip to stripe connectivity may require
4:40 the rocket2 which is available in the
4:42 industry uh for quite some time now so
4:46 that that that's what changed right some
4:47 architectural aspect some additional
4:49 things that we'll be discussing later on
4:51 probably but we we definitely see that
4:54 uh there are advances which actually uh
4:57 confirms that whoever started to work on
5:00 this technology some time ago I did the
5:02 right choice in terms of decision making
5:05 process fantastic I'm glad now that we
5:09 understand uh the value of the
5:11 technology has increased over time you
5:13 know especially when we hearing more
5:16 about Rocky
5:17 V2 uh that brings to my next question
5:21 how are the Rocky V2 components
5:24 coordinating inside the DC fabric I
5:27 recall uh the two components
5:28 particularly the BFC and the ecn inside
5:32 the IB glass fabric no it's it's a good
5:35 point Ain so uh in fact so you cited the
5:38 two big components so I mentioned in the
5:39 first uh uh part of our discussion that
5:43 we want to transport the the the the
5:45 payloads of the infin band using the UDP
5:48 encapsulation across the fabric so
5:50 between the leave devices from one GPU
5:53 uh to another GPU we are just sinking
5:55 these chunks of datas uh using the the
5:57 transport over over internet IP UDP but
6:01 the the the two aspects that are
6:03 important here is to make sure that
6:04 whenever there is a congestion that
6:06 occurs on on the network that we
6:10 actually handle this congestion in the
6:11 right way so you have these two
6:13 mechanisms prial control uh using the
6:16 dscp uh markings and also the ecn right
6:20 so now the question is once you get into
6:22 the situation with the with the
6:24 congestion uh which one will will kick
6:26 in first right so quite often it depends
6:29 on the implementation in case of most of
6:32 the implementations we have the ecn
6:34 which will will kick in first and we'll
6:36 actually sand on the data plane Pockets
6:40 markings of the one to one uh into the
6:43 destination GPU and so the destination
6:45 GP will realize okay there is a there is
6:48 an information that we I have some
6:50 congestion so I need to react to it and
6:52 it's going to S the information to the
6:54 originator of the of the flow right to
6:57 slow down a little bit and then if after
7:00 some time there are still congestions
7:02 occurring only then the PFC will kick in
7:05 on the back end and then will inform one
7:08 by one hop by hop on the point-to-point
7:10 level that there are uh switches on down
7:13 the road to to slow down uh the the the
7:17 the the the speed at which the data is
7:20 is being sent right so there is that
7:22 level of coordination at the per node
7:24 level for these two mechanisms that you
7:26 you mentioned it's good to know that the
7:28 components of Rocky V2 are really well
7:31 coordinated inside the fabric but how
7:34 can an operator be sure that they're
7:36 really working oh that's that's a
7:38 challenging actually question because as
7:40 a matter of fact uh let's be precise so
7:43 the the the mechanisms that we discussed
7:45 the ecn and PFC they are acting actually
7:48 at the subsecond level right so we talk
7:50 about the microsc accuracy here so it's
7:54 it's it's fundamental to make sure that
7:57 actually at the switch level and the uh
7:59 actually lower level of the Asic we have
8:03 also the components which are capable of
8:05 tracking the urance of the of the
8:08 congestions so let's take a a simple
8:11 example so as an operator I want to make
8:14 sure that my uh GPU fabric my AIML
8:19 cluster fabric is actually in the steady
8:21 state so I'm I'm checking on my
8:23 monitoring stations that actually the
8:26 occurence of these congestions pretty
8:27 low right I in in case I see them on and
8:30 on being triggered it means that
8:32 something is wrong in my design or my
8:34 settings of my Fabrics are not good
8:36 right or maybe I over subscribe my my my
8:39 infrastructure so it's important to make
8:41 sure that we have this ecn and PFC
8:43 Telemetry information streamed at the
8:46 perq level uh to the uh station such as
8:49 for example the abstract fabric manager
8:52 where we can visualize okay for this
8:54 component on this component of the
8:56 fabric I do have some situations which I
8:59 need needs to fine tune right so that's
9:01 that's the example and so we can see on
9:04 the slide we have this capability of
9:06 visualizing also the buffer ring
9:08 utilization right so that aspect of
9:10 buffer is key because it means that as
9:13 long as I'm utilizing uh intelligently
9:16 my my my buffering at the ESS ports in
9:19 my in my fabric then in theory I should
9:22 not trigger any of these PFC or ecn
9:26 mechanisms right to control the
9:27 congestions right so so monitoring
9:30 having Telemetry for Rocky V2 per Q is
9:33 instrumental right to make sure that we
9:36 have a full understanding of the
9:37 performances of the fabric so besides
9:39 the ecn and PFC monitoring are there any
9:43 more advanced Rocky V2
9:45 capabilities well yes we we have
9:48 situations where actually we can
9:49 mitigate some of the situations where
9:52 well the fabric maybe uh a little bit uh
9:55 in a in a wrong situation where the
9:57 congestions are accur Ing and so we want
10:00 to make sure that the occurence of the
10:02 congestions inside the fabric are not
10:04 actually having repercussions on the
10:06 rest of the of the GPU uh uh workload
10:10 exchanges right so there is for example
10:13 a functionality called PFC watch do
10:15 pretty popular in the industry where uh
10:18 we actually uh want to go through this
10:22 situation where uh if there is an
10:26 avalanche of pfcs which are which are
10:30 received on the Ingress Port that if we
10:33 consider that this Avalanche is is a
10:35 continuous rate of the PFC so the push
10:38 backs are received on and on from a
10:41 specific uh Upstream switch then we can
10:44 consider okay this is not a normal
10:46 situation and we can simply ignore these
10:49 packets right ignore these packets
10:51 instead of pushing them down uh to the
10:53 uh actually uh Downstream switches we
10:56 will simply say okay I ignore these
10:58 buckets or eventually as an option I can
11:01 say okay in order to mitigate that
11:04 situation of the congestion on that
11:07 specific queue I will decide to actually
11:09 drop the packets in order to stop the
11:12 congestion on a specific segment of the
11:15 fabric right so it goes through the
11:17 distinct three states this PFC watchop
11:19 implementation used on a specific note
11:22 we have this on the diagram where the
11:24 spine one was enabled with the PFC watch
11:27 do which is actually spine AG creating
11:29 connectivities from different Leaf
11:31 devices on which the GPSs are connected
11:34 and so the detection timer will actually
11:36 go through the cycle and check how much
11:39 of these PFC messages were received and
11:41 so if it considers that in the window of
11:44 time it received too much of this it's
11:46 gonna just simply say okay probably
11:49 there it's not a normal situation so I'm
11:51 going to ignore them instead of
11:53 penalizing all the rest of the fabric
11:56 from from The Slowdown right so in order
11:59 to conserve the good performances for
12:01 all the rest of the gpus I will actually
12:04 consider this as a function to control
12:07 how uh actually the the reach of the of
12:09 the PFC is spread across the fabric so
12:12 pretty good uh function whenever we rely
12:15 a lot of PFC I think it's worth to
12:17 consider that kind of of mechanism so
12:20 that's one and then there is another one
12:22 which uh is uh is is trying to help
12:25 actually optimize the way we use the PFC
12:27 the priority flow controls is the is the
12:30 the PFC X on x off so whenever we
12:34 discuss the uh the rocky V2 PFC priority
12:38 flow control we also need to think about
12:40 how the buffering utilization happens so
12:43 if we have a topology you can see on the
12:45 on my uh left hand side so we have the
12:48 the leaf spine topology uh where
12:51 actually on the et2 of the spine one
12:53 there was a congestion that happened
12:55 right so in this situation uh well we
12:58 need to to think about at which moment I
13:01 should start generating my for example X
13:03 off messages which are the PFC uh uh
13:07 control messages which are saying to the
13:09 downstream device in this case for
13:11 example the leaf one to actually slow
13:13 down keeping buffers a little bit the
13:15 packets instead of continuously send
13:18 them and then get the congestions on the
13:20 et2 of the of the spine one so as you
13:23 can see we have this uh opportunity here
13:26 to uh actually control the uh the buffer
13:29 utilization at the per Q level by
13:31 setting something that is called Alpha
13:33 values so by setting different values
13:37 for uh the alpha at the per Q level we
13:40 can actually control how often actually
13:44 the x of messages will be uh sent right
13:47 so if for example I set my uh ex of
13:50 alpha value messages to uh a higher
13:53 value that the chances of the occurrence
13:55 of of the PFC uh will actually go down
13:59 right so if you see on the left hand
14:02 side we have a typical buffer thresholds
14:05 and so the x of X on as well as the head
14:08 Headroom they are representing these
14:10 three thresholds and in function of the
14:12 settings of the either Alpha value or
14:14 Exon offset we can control actually on
14:18 how often uh the the PFC messages are
14:21 actually triggered right so uh in order
14:24 to be actually a little bit more precise
14:26 it's always better to uh to take an
14:28 example of it so I have an example of a
14:32 simple topology we have the two ports
14:34 et0 and et1 connected on the same switch
14:37 for example a qfx
14:40 5230 uh and then we have an outgoing uh
14:43 uh interface et20 right so in this
14:46 situation we have on the same outgoing
14:48 interface two q's q0 and Q3 and they are
14:53 set with two different I of alpha
14:55 volumes so let's say we decide to set uh
14:59 the alpha value of nine for the q0 and
15:02 then the alpha value of seven for the Q3
15:05 so you can see that depending on the
15:07 values of these Alphas the outcome is
15:10 that I'm going to get a different number
15:12 of cells different number of uh buffers
15:16 for each of the cube so I share also the
15:19 formula on how we can actually calculate
15:21 fine tune these these buffers and so in
15:24 reality uh where exactly uh we would
15:27 consider Advanced complicated
15:30 calculations so we may have situations
15:33 where actually the specific large
15:36 language models are more important in
15:38 terms of data processing than the others
15:41 right they need to get the data synced
15:44 faster right so for this kind of L large
15:48 language model we large language models
15:50 we don't want to actually slow down uh
15:52 the the data exchanges and so we would
15:55 typically allocate higher Alpha values
15:58 right that's that's that's one of the
15:59 example so so we can see we have these
16:02 two functionalities I explained one is
16:04 the PFC watch doog the other one is the
16:07 X off Alpha values where actually the
16:11 the the administrator of the fabric can
16:13 fine-tune the fabric to make sure that
16:15 if he gets the best of the
16:18 bandwidth deployed in the fabric so with
16:21 with the best of the bandwidth of 400 G
16:23 800 gig deployed in an AML cluster so I
16:26 think this is important a little bit
16:28 more advanced but this is what actually
16:30 the the the industry uh is proposing to
16:33 make sure that actually this AML cluster
16:36 deployments are really good in terms of
16:38 performance and then case scale at the
16:40 larger volume right this is interesting
16:43 at the as these uh Advanced options for
16:46 rocky2 help to get the proper congestion
16:49 control
16:50 settings but as the Enterprises and the
16:53 cloud providers are building the AIML
16:55 clusters are there any other
16:57 Technologies that they must consider
16:59 Michael good point actually the aspect
17:02 of innovation still is increasing right
17:05 in the context of AML clusters there is
17:08 even more Innovations in terms of
17:10 software where uh instead of having a uh
17:14 traditional load balancing mechanisms
17:17 there are a lot of more efficiency uh
17:20 which uh comes into play especially when
17:22 you consider the AIML workloads the
17:25 entropy of these workloads so the the
17:27 variation in terms of characteristics of
17:29 the flows are relatively low right
17:32 comparing to traditional server uh type
17:35 of communication so in order to make
17:37 sure that we still use the max of the
17:40 capacity of our AIML cluster fabric uh
17:43 there are a lot of enhancements around
17:46 the load balancing uh so some some some
17:49 of them are for example the dynamic load
17:51 balancing where the characteristics of
17:54 the flow are not the only entry but also
17:56 the band with the real time bad with
17:58 utilization of the outgoing links on the
18:01 IP CMP groups are Incorporated in the
18:04 calculation of the hashing that's one
18:06 example another one is for example the
18:08 glb the global load balancing where
18:11 actually we track also the situation on
18:13 the next to next hop node in order to
18:16 check what are the performances on the
18:18 next hop nodes in order to consider the
18:21 the right path locally on the device and
18:24 then the last one is the traffic
18:25 engineering uh inside the fabric where
18:28 as the administration uh task we can
18:31 decide okay that there are for example
18:33 elephant flows and mice flows that they
18:35 they going to always go on two diverse
18:38 paths inside the fabric and will have a
18:41 control on which of the next hope they
18:42 going to use so a lot of advancements a
18:45 lot of innovation around also load
18:47 balancing right which is key inside the
18:49 AML cluster networking our own right
18:52 awesome so once again thank you Michael
18:56 for uh just being p patiently ly
18:58 answering a lot of questions and this is
19:01 uh this is going to be great because uh
19:03 I'm learning a lot of new things as well
19:05 uh as we as we talk and uh thank you to
19:07 all the viewers I would uh my suggestion
19:11 is going to be stay tuned to learn more
19:12 about the AI data center networks in our
19:15 next set of videos with that have a
19:18 wonderful rest of your day thank you
19:25 man