Congestion Management in the AI Data Center
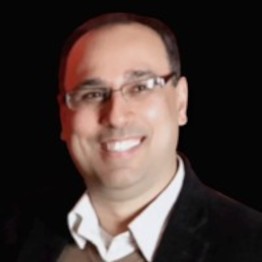

Congestion Management in the AI Data Center
Juniper’s Arun Gandhi, Mahesh Subramaniam, and Michal Styszynski discuss the cutting-edge congestion management technologies needed for the lossless ROCEv2 transport networks when connecting GPU clusters.
You’ll learn
How to practice proactive congestion management vs. reactive
The main building blocks of congestion management
Nuances for techniques in AI data center management
Who is this for?
Host
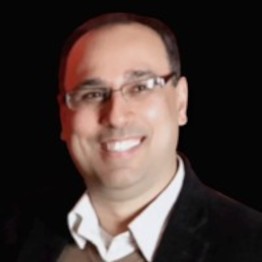
Guest speakers
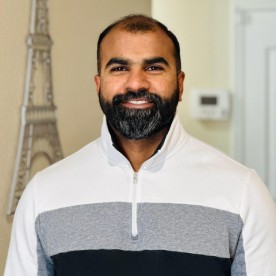
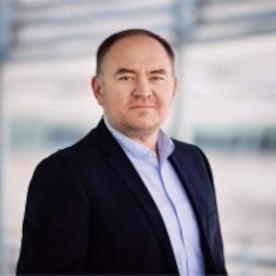
Transcript
0:00 [Music]
0:05 hello everyone welcome to the third
0:07 episode of the video series for the AI
0:10 data centers in the last episode with
0:13 himansu and Mahesh we discussed how load
0:16 balancing is enabled in the AI data
0:18 center fabric with features such as
0:21 Dynamic load balancing and Global load
0:24 balancing improving the efficiency of
0:25 the fabric to kick off our discussion
0:28 today I'm joined by Mr special guests
0:31 and good friends Mahesh suban and Mikel
0:34 cinski today we will discuss the cutting
0:37 edge condition management Technologies
0:39 needed for the lossless Rocky V2
0:42 transport networks when connecting the
0:44 GPU clusters Mahesh and Mikel welcome to
0:48 episode
0:49 three hey Aron hey Mahesh hey AR great
0:52 to see you again hi Aron happy to be in
0:56 your video again and uh looks like today
0:59 I did not get the
1:01 memo yeah so let's start with you Mahesh
1:04 u in the previous video we discussed the
1:08 advanced load balancing techniques in
1:10 the AI there is in the
1:12 Clusters also these backend AI clusters
1:15 often one is to one over subscribed or
1:18 have very efficient load balancing in
1:20 place so why is congestion management
1:24 still needed in the AI clusters yeah
1:27 Aron congestion management even it's
1:29 starts from the efficient and effective
1:31 load balancing everybody knows that in a
1:36 cluster infrastructure the overall goal
1:38 is to have a reduced job completion time
1:41 that's a key kpi but in a data center
1:46 infrastructure our key KP is a lossless
1:49 fabric because during the RDMA
1:52 transition from 1GB to other GPU even a
1:55 single drop will make the job cycle to
1:59 run
2:00 again so the real game here is to how we
2:03 are handling the congestion proactively
2:05 with reactively what I mean here is that
2:08 in a GPU cluster on the fabric uh we
2:11 have to control the congestion
2:13 proactively using a efficient load
2:15 balancing little bit in detail in the
2:18 proactive method uh we'll make sure if
2:20 any congestion happen on the particular
2:22 link or a path we we will switch that
2:25 particular flow from congested link to
2:28 less congested link right during various
2:31 method last video also we discussed like
2:33 a DLB Dynamic load balancing or
2:35 selective DLB identify the BT header
2:38 elephant flows and accordingly will
2:40 spray the packet various methods are
2:41 there but in at any point of time due to
2:46 microburst if any link got congested we
2:50 have to immediately jump in do some
2:53 reactive methods that's where we have a
2:56 proper congestion method coming into the
2:58 picture via ecn and bfcs up today this
3:01 is an interesting start Mahesh can you
3:04 share a few scenarios in the reactive
3:07 approach yeah there are multiple
3:08 scenarios are there but I want to go a
3:11 little bit of basics in any data center
3:14 uh we have a different congestion points
3:17 and we have a called it as in uh incast
3:19 congestion points right what is incast
3:21 means basically multiple input to only
3:24 one output it will make a congestion
3:27 this particular incast congestion uh
3:30 generally it will happen in three points
3:32 where a GPU cluster to Leaf that's a
3:34 point one or Leaf to the spine which is
3:37 point two or spine to the leaf this is
3:40 point three so how we are going to
3:42 handle the congestion in the switch or
3:44 switch fabric that's where we need a
3:46 proper congestion methods and since you
3:49 asked about the scenarios like for
3:51 example we have a kind of a uh in every
3:54 A6 in every switch in our A6 have a mmu
3:57 which is memory management unit this
4:00 memory management unit has two parts one
4:03 is that itm that Ingress traffic manager
4:06 and uh Ingress traffic manager zero and
4:08 Ingress traffic manager one this Ingress
4:10 traffic manager itm will allocate the
4:13 buffer to our switch it can be a
4:16 dedicated buffer or it can be a shared
4:18 buffer depends upon the AC can switch
4:20 what you are using it right in the queue
4:23 in the buffer it got filled then became
4:26 a congestion so we need to avoid the
4:27 congestion that's where we start started
4:30 using some kind of a key congestion
4:32 mechanism for a rock2 traffic we can
4:35 call it as a DC qm that data center
4:37 quantize uh congestion uh method in the
4:40 DC equation is nothing but the
4:42 combination of ecn plus PFC that's what
4:45 we are going to see it this in the video
4:47 in detailed way this is very helpful and
4:50 and uh I want to move to Mel here so M
4:54 can you remind us uh what are the main
4:56 building blocks of the condition
4:58 management yeah yes AR so in fact there
5:01 are two methods right for congestion
5:03 management right the explicit congestion
5:05 notification is in fact the the most
5:08 popular uh portion of the congestion
5:10 management and there is obviously the
5:12 second mechanism which is the priority
5:14 flow control using the
5:17 dscp both of these mechanism run on the
5:20 native IP Fabrics where you have Leaf
5:22 spine super spine topology but in some
5:25 scenarios only the ecn the explicit
5:28 congestion notification is used as a
5:30 primary one which simply informs the
5:34 destination uh server about the the
5:38 congestion right so of course there is a
5:40 prerequisite to put this functionality
5:43 on the switches and without uh having
5:47 that functionality on the ni cards we
5:49 can't really uh rely on that congestion
5:52 management so that's the portion which
5:54 is very important making sure that the
5:56 congestion management we are triggering
5:58 on the switches is also Al supported on
6:00 the ni card itself right so the driver
6:03 of the server have to has to be uh
6:06 capable of of interpretation of the ecn
6:10 messages received from from the from the
6:13 network right and so the ecn is is
6:17 simply mechanism which will inform the
6:20 destination server about the congestion
6:22 when actually it reaches the threshold
6:25 set at the perq level on the switches
6:28 right so for example set in your uh
6:31 spine devices on a specific queue on the
6:33 lossless queue that the when you reach
6:37 that threshold you will start marking
6:39 your pocket with one one bat which is
6:42 the most significant beats of the to uh
6:46 on the IP and you will inform the
6:48 destination server about the the the
6:50 scenario of the uh of the congestion
6:52 right in this case the server will get
6:55 that information and will react to it
6:57 right so it will send back the
6:59 information to the originating server
7:02 and it will just mark it with specific
7:04 values to inform it that he needs to
7:07 slow down a little bit for very short
7:09 time and then you know reduce the
7:11 congestion or simply uh eliminate the
7:13 congestion right so when it it sends
7:16 back the the CNP message to the
7:18 originating server the source of the of
7:21 the of the rocky V2 packet then it will
7:24 get this information with the uh Des CER
7:29 information so that we know exactly for
7:31 which session it needs to reduce the the
7:33 rate and also the partition key is part
7:36 of the information right when it sends
7:38 the CNP packet back to the originator
7:41 right so then the partition uh
7:43 information The Logical information is
7:45 also leveraged so that the originating
7:47 server knows exactly on which of the
7:50 session it needs to reduce the uh the
7:52 rate in order to prevent uh from of of
7:56 of having this congestion inside the
7:57 network right and then the second
8:00 mechanism PFC uh is actually triggered
8:03 just after uh the the explicit
8:06 congestion notification when actually
8:08 the congestion still happens right so if
8:10 we if we trigger the ecn and ecn
8:13 actually reduce the congestion then a
8:15 PFC sometimes is not even triggered
8:17 right back to the originating server
8:19 right so instead of having a situation
8:22 of of of a switch which sends both PFC
8:26 and ecn to the destination PFC actually
8:28 is sent to the original ating Ser
8:30 directly from the switch hop by hop it's
8:32 acting on each of the segments and can
8:35 inform also additionally uh on each of
8:38 these segment to slow down reduce the L
8:40 rate and then again uh you know prevent
8:43 from a from a from a scenario of
8:45 congestion inside the the network right
8:48 both of these mechanisms are are are
8:50 supported on the switching side but
8:52 again I will repeat that it's also
8:54 important that we we have a right
8:55 interpretation of these values on the KN
8:57 card the good thing is that both of
8:59 these mechanisms are the most widely
9:01 supported congestion mechanisms uh in
9:04 the industry there are other ideas about
9:07 how to run the congestion Management on
9:08 the fabric but the reality is that you
9:11 need both of these portions so this one
9:13 is really the most popular one and is uh
9:16 uh is available across multiple vendors
9:19 so you can build your Rocky V2 uh AI ml
9:22 fabric using different vendors and they
9:25 can support both of these standardized
9:27 mechanisms for the congestion management
9:29 right I'm glad you mentioned that they
9:32 are very well known and methods both ecn
9:35 and
9:37 PFC so are there any nuances in these
9:39 techniques for the AI data
9:42 centers that's a good point so uh AR
9:45 last time we discussed about some more
9:46 advanced functionalities of uh how to
9:49 for example manage uh the PFC using the
9:54 uh the concept of alpha values right so
9:56 you can set different values of Alpha
9:58 and then provision the way the ex off
10:02 off is actually triggered to actually
10:04 pause the the the streams to the
10:08 originating uh uh the originating server
10:11 right uh so these values can be actually
10:14 enabled at the perq level and then you
10:17 can for example decide that for very
10:19 specific uh large L model you will
10:23 actually provision a little bit more of
10:24 the buffer so the trigger of the X off
10:27 the PFC messages will will happen uh
10:30 with lower probability right so that's
10:32 one thing the other thing that we
10:35 discussed last time was also the the the
10:37 TFC Watchdog quite popular functionality
10:40 across different vanders where if you
10:43 rely on PFC if it's the case of your
10:45 network you can actually control uh uh
10:49 the situations where where the network
10:52 is experiencing some abnormal triggers
10:55 of the PFC where the rate of the PFC is
10:58 received too often then on the switch
11:00 you can say okay if I get too much of
11:03 these PFC back pressures in the given
11:05 window of time I will simply ignore them
11:07 and just uh you know stop pushing it
11:09 down to the originating servers right
11:12 but you ask me about some nuances right
11:14 there are other nuances so one of the
11:16 nuances is simply at the switch level
11:19 how you handle your buffers right so the
11:22 way you can handle it is of course uh do
11:25 it at the per level for specific
11:27 congestion management mechanisms as said
11:30 using the alpha values for the pfcs uh
11:33 but also on the switch itself you can
11:35 manage these buffers and are reallocate
11:38 dedicated buffers from the interfaces
11:40 you're not using for example on your
11:43 switch reallocate the the buffer memory
11:46 to the shared pool and then give a
11:48 little bit of a more of the of the of
11:50 the buffers for the shared pool in order
11:52 to be used in case of of the congestion
11:55 situation right so that that would be
11:57 another area to to explore whenever you
12:00 have a the spine supine IP fabric
12:02 topology making sure that you have a
12:05 consistency at each of the level in
12:07 terms of the treatment of the of your
12:09 buffers right so very important buffers
12:11 associated with the congestion
12:13 management that we discussed so far
12:15 making sure that the switch is
12:17 provisioned in the right way great
12:19 points Mikel uh I think this been a
12:21 great session so far but before we uh
12:25 close I must ask uh this question and
12:28 I'll you know go to Mahesh here Mahesh
12:31 what other Technologies are being
12:33 enhanced to support the AI data centers
12:36 Aron you asked about a data center in
12:38 the fabric perspective there are two
12:40 things you know that one is that
12:41 congestion management and load balancing
12:45 in a congestion Management in both areas
12:47 there are a lot of things happening let
12:49 me start with the congestion management
12:51 of course there are uh how we are going
12:53 to handle this congestion and queuing
12:56 mechanism at the edge we'll call it as a
12:59 qds HQ datagram services and also of
13:02 course you guys know Amazon already
13:04 started using a scalable uh reliable
13:06 datagram and in the transport level Plus
13:10 in the ultra ethernet Consortium we are
13:12 very well part of it uh there is a f CNP
13:15 we'll call it as a source flow control I
13:17 think a. 2.1 qdw uh the standard which
13:21 is evolving drop congestion notification
13:24 uh congestion signaling how you're are
13:26 going to handle the uh congestion tag
13:28 and how you going to reflect that we'll
13:30 call it as a congestion tag and
13:31 congestion reflection tags how we are
13:34 going to handle it these are things are
13:35 happening in the congestion um uh
13:38 management area and forgot to say one
13:41 more thing there is a credit based flow
13:43 scheduling or control that is also one
13:45 of the key thing it's happening in the
13:47 ultra ethet Consortium we already myself
13:50 Mikel and one of my colleagues also
13:51 started doing a lot of research on it
13:53 and soon it will be uh once it's
13:56 available definitely it will be
13:57 incorporated in our switch as well
13:59 that's for congestion management for
14:01 load balancing side uh there are many
14:04 things happening again uh one of the key
14:07 uh feature under the cognitive routing
14:09 broadcom introducing a glb that is a
14:11 global load balancing mechanism the
14:14 dynamic load balancing is a local
14:15 significance where the glb can
14:17 communicate the quality table and the
14:19 link Q depths from one switch to another
14:21 switch uh using their uh propriety e
14:24 mechanism that already we started
14:27 supporting in our Juniper switch
14:29 portfolios and the second is that in
14:32 network collectives that's going to be
14:34 soon it's going to be the Talk of the
14:35 Town and basically what we are going to
14:38 do how the collectives going to
14:40 communicate from one fabric to another
14:42 fabric via one switch one server to
14:44 another server via fabric that's nothing
14:47 but in network collectives how we are
14:49 going to reduce the data copies and how
14:52 we are going to reduce the data movement
14:54 in the consequence we are going to
14:56 reduce the uh link utilization
14:59 and automatically job completion time
15:01 will be reduced uh these are the two
15:03 things we are working on and of course
15:05 the thermal management power efficiency
15:07 you of the key things uh Even in our
15:09 strategy also we are incorporating uh
15:12 which we will be uh doing a deep dive in
15:15 for the videos as well it's been a great
15:17 learning experience and I'm sure viewers
15:19 are enjoying as well uh listen to both
15:22 of you so with that we conclude our
15:25 today's session uh thank you both and to
15:28 our viewers
15:29 uh stay tuned to learn more and we'll be
15:32 coming up with a new episode shortly
15:37 [Music]