Bob Friday Talks: Getting to know and love Marvis
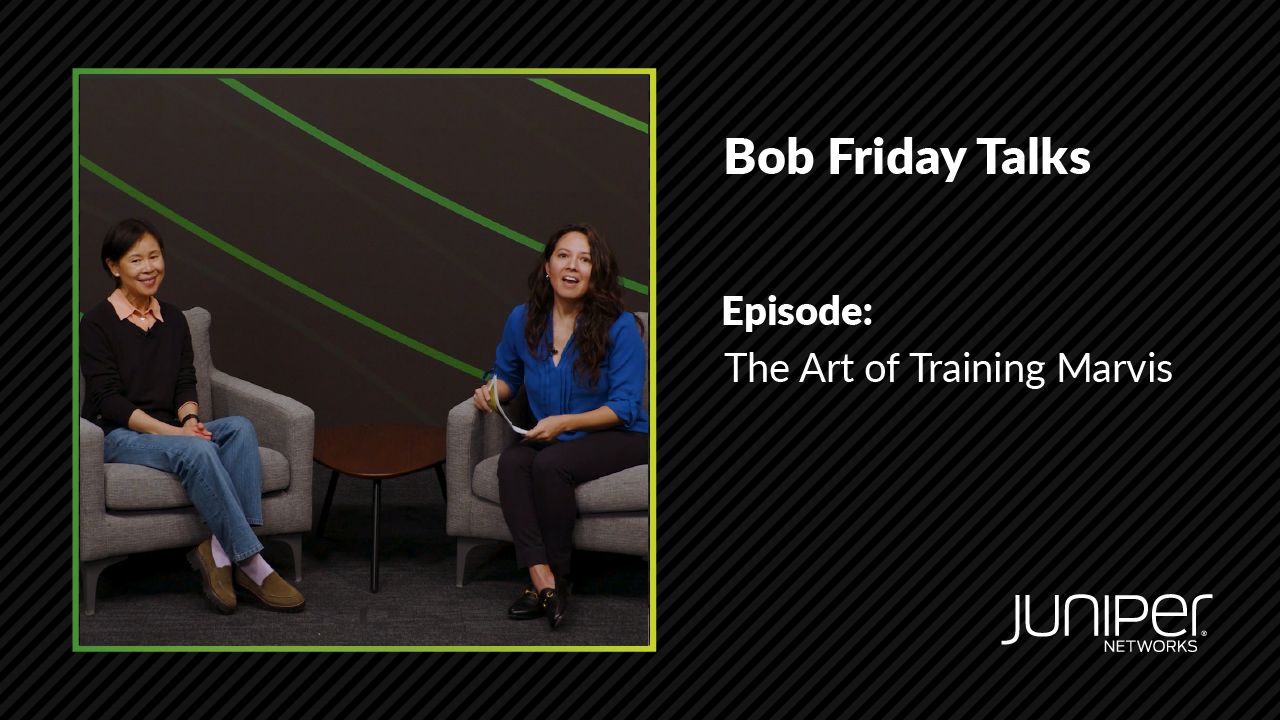
Getting to know and love Marvis
In this episode of Bob Friday Talks, guest host Jessica Garrison sits down with Juniper’s Sr. Director of Software Engineering, Shirley Wu, for a fireside chat on all things Marvis. In addition to an overview of Marvis, they discuss the roadmap of Marvis to date, which teams working on Marvis play the most important role from a data perspective, how Marvis utilizes AI and ML, and the benefits of Marvis Actions UI, aka the Octopus.
You’ll learn
What Marvis is and how it has evolved
Why the firmware and hardware teams are so important to Marvis’ development
How Marvis works from an AI and ML perspective
Who is this for?
Host
Guest speakers
Transcript
0:00 thanks for joining us today we're going
0:02 to embark on a journey into the heart of
0:03 AI networking with our guest Shirley woo
0:06 we're going to dive into her insights
0:08 about Marvis the new iteration with
0:10 Marvis Minis and much more Shirley thank
0:12 you for joining us can you tell us a
0:14 little bit about yourself thanks Jessica
0:16 it's a great pleasure to be here so my
0:19 name is Shy I start the Mist for Juniper
0:23 for last five years right so I'm lead
0:27 Mist a Marv team and I'm senior director
0:31 so for the last five years we had a
0:33 great journey with Marvis and the AI of
0:35 course but now you know it's a great
0:38 question for me to look back for my
0:40 career and I actually just realized that
0:43 my career growth and the development
0:45 path is somehow totally aligned with the
0:48 industry growth so remember I remember
0:51 when I first finished my master's degree
0:54 in computer science I did spend quite a
0:56 few years to find my passion and the
1:00 Direction where I want to be what I
1:01 wanted to do even though I'm you know
1:03 have a daytime job in the software
1:05 development Cy industry so really
1:10 critical moment for me is that I decide
1:12 to jump into the startup so the first
1:15 ever startup company I ever joined was
1:17 in a data analytics uh space so we
1:20 trying to use the customer consumer data
1:23 to find the customer insights to predict
1:25 the customer turn and at that time that
1:28 you know that was before the Big Data AI
1:31 ml became a b word so we have a quite a
1:35 lot of challenges to deal with the data
1:37 Volant how we are going to apply very
1:39 accurate uh um statistical algorithms to
1:42 predict the you know customer turn so
1:46 then the other company had a very
1:48 successful acquisition so after the
1:50 acquisition I'm kind of reevaluated my
1:52 career where want to be what I want to
1:54 do so as that the same time that the Big
1:57 Data Hado and also cloud computing
2:01 became the best word that totally
2:03 revolutionize the software development
2:06 industry so that the moment I also St
2:09 into my second startup I joined the very
2:12 early um stage startup in cyber security
2:16 but even though the business problem is
2:18 different but the technology behind is
2:21 totally different different we utilize
2:24 cloud computing and Big Data tools we
2:27 trying to to identify abnormal user
2:30 behaviors to identify cyber security
2:33 threats so that was a really um uh you
2:37 know exciting Journey you know utilize
2:40 the AI and the big data to solve the
2:44 problem which is very traditional in the
2:46 cyber security space right so that
2:48 company also had a very you know uh
2:52 quite exciting Journey after acquisition
2:55 then you know time run into the Mist so
2:58 at the moment the same thing that hey
3:01 the Big Data actually came to another
3:03 chapter is a very mature space yeah so
3:06 people trying to utilize data cloud
3:09 computing AI trying to revolutionize the
3:11 traditional uh space like networking so
3:15 that's why came to the moment I joined
3:16 the mist and uh the mission is uh
3:20 utilize our Cloud infrastructure data
3:23 and AI how can we revolutionize the
3:26 networking operation so that's the
3:28 journey I came from so the you know the
3:31 I believe the really critical moment for
3:34 me is have a gut to find your passion
3:37 find whatever you believe and uh uh
3:41 trying to utilize data to solve the
3:43 problem so we know that Juniper's
3:45 customers love and use Marvis part of
3:48 juniper Juniper's AI native networking
3:50 platform can you give us an overview of
3:53 maybe the top two or three features of
3:55 the platform so Marvis actually is a is
3:59 a best word represent a lot of thing but
4:01 the core of the Marv I could think about
4:04 right as of today is that two or three
4:07 things first is Marvis is a you know not
4:10 more than one piece of software sits in
4:12 the cloud continue to S through the
4:16 stats and events streamed into the cloud
4:20 from juniper Cloud managed devices so I
4:24 will go through the live data stream to
4:26 we can close to near time near real time
4:29 be able to detect problems on the
4:32 customer's Network so this is the one
4:35 that we can have a we don't need the
4:37 network administrators continuous to
4:39 monitor their Network we can utilize our
4:42 data and the cloud to help the customer
4:44 to monitor the network corporations this
4:46 is a fundamental key difference compared
4:49 to traditional networking companies and
4:52 vendors so second important feature is
4:55 Marv not just detect the problems we
4:58 also can identify cause of the problem
5:01 if the problem is self fixable Marvis
5:04 will fix it but if the problem Marvis
5:07 cannot the fix they will gener create
5:10 the recommendations and send a web hook
5:12 email notification to the customer to
5:15 how to address this issue so this is the
5:18 fundamental two features but in the
5:20 recent news release you probably heard
5:22 about Marvis Mimi this is another great
5:25 feature for Mar that we release a
5:28 feature be able to auto made self
5:30 diagnostic analysis and be able to gener
5:33 report so that we can find the problems
5:36 before humans encounters them for our
5:39 customer so you just spoke about Marvis
5:41 minis which is one of the newer features
5:43 how have you seen Marvis evolve over
5:45 time when I first joined the m and the
5:47 Juniper early days that the Marvis just
5:50 be able to answer simple questions like
5:53 we call the virtual Network system so we
5:57 collect the stats events we build the
5:59 the time series and we can answer very
6:03 limited user queries that's early the
6:05 Maris so we when we join the Builder the
6:08 Marvis team we spend a significant chunk
6:11 of resources to to develop the Marvis
6:14 platform which be able to streaming
6:17 through um the live data to identify the
6:20 problem abnormal behaviors about the
6:23 network and also identify the um the
6:27 events and generate the root causes so
6:29 this Marvis event action framework we
6:32 developed on the cloud so this the
6:34 second phase of the Marvis now you know
6:36 the third is you know the we based on
6:39 the detected events actions we develop a
6:42 self um remediation framework and also
6:47 self optimization framework another one
6:50 is the latest Mar meaning we're talking
6:52 about the self dtic
6:54 framework so this is a mar on the road
6:57 map we have so far yeah
7:00 so you talked about like the different
7:01 layers there right and at the beginning
7:04 of it was the data which is where your
7:07 interest mostly lies um so what are the
7:10 foundational Technologies in terms of
7:13 the data piece of it that Marvis uses so
7:16 you brought another good question
7:18 typically people talk about Marvis is
7:20 hey is a data science team Marvis team
7:22 so I feel very embarrassed actually yes
7:25 we as a Marv team data scientists and
7:27 machine learning Engineers we contribute
7:29 a lot to maris's success but on top of
7:32 that actually we have a lot of Engineers
7:34 that are working on the cloud
7:36 infrastructure but most importantly our
7:38 firmware and Hardware teams they are the
7:42 the core to make sure our devices be
7:45 able to generate meaningful accurate
7:48 data that data is a source for Mar's
7:52 success so for Marvis you know one hand
7:55 is a lot of softwares infrastructure we
7:57 build on the cloud on top of that is
8:00 human human is you know data scientists
8:03 machine learning Engineers but on top of
8:05 that we also have a cloud infrastructure
8:08 engineers and the most important domain
8:10 experts and our AP Hardware switch and
8:15 SD van Hardware software Engineers
8:17 collectively work together to create
8:20 developed intelligence for the Maris so
8:23 you just kind of talked about all the
8:24 different teams that it takes to create
8:27 Marvis um can you give us a little bit
8:30 more about how it works from the AI
8:31 perspective so typically uh for Marvis
8:35 we start actually from a customer
8:37 support tickets so when customer we open
8:40 the support tickets we go through that
8:43 tickets with our C security team
8:46 identifies certain Cur tickets actually
8:49 the we could Marvis has already have an
8:51 answer for those Marvis does not have an
8:54 answer we work together with our domain
8:57 experts trying to identify that you
8:59 first of do we have the data in the
9:01 cloud can I capture that type of
9:04 behavior or problem second if we do then
9:08 you know we will have a multiple working
9:11 sessions with our domain experts the
9:14 working session of course we are data
9:16 science team and machine learning
9:17 Engineers working with the domain
9:19 experts we share our data analysis
9:22 results with them and the based on this
9:24 recommendation we continues to fine tune
9:27 our data analysis results and apply that
9:30 alysis apply to Mis Universe we
9:33 generally call the Mis Universe which
9:35 including our multiple Cloud production
9:38 environments to validate the results and
9:41 the based on the validation results then
9:44 we decide this is a um from machine
9:47 learning perspective this is a you know
9:49 the classification problem or is a
9:51 prediction problem then we can decide
9:54 what type of a machine learning
9:56 algorithms we want to use and on top of
9:58 that have to evaluate the you know Cloud
10:02 computation cost yeah and the latency
10:05 how how you know if we going to put in
10:08 the streaming or put in the platform the
10:11 batch process to do this to to implement
10:15 this use case how much of latency we can
10:18 tolerate and how much of the cloud cost
10:20 and of course most importantly is um and
10:24 how difficult to debug this issue if we
10:27 deploy in certain environments actually
10:30 the we may not be able to have access
10:33 like a go Cloud environments right so
10:36 based on this Factor we kind of finalize
10:39 our implementation then we deploy the
10:42 solution to the cloud then we are not
10:44 making customer faion we continuously
10:46 validate validate results with our
10:49 domain experts and you and also trying
10:52 to Val dat cases with friendly customers
10:56 until achieve certain confidence level
10:59 this solution can apply to Miss Universe
11:04 doesn't matter is gcp or in the databas
11:07 doesn't matter you retail customer or
11:09 university campuses we make sure our
11:12 solution is be able to adopt to
11:15 different environments then we'll make
11:17 it customer facing features so this kind
11:20 of general that the uh the development
11:24 cycle we have for any Mar Solutions so
11:27 it's not generally just a AIML about
11:30 data a lot of interactions with the
11:34 domain experts continues valid dat with
11:36 the customer use cases and their
11:39 specific environments so so this is kind
11:41 of the Journey of a mar is that how we
11:43 gain The Confidence from our customer
11:46 it's not just the data scientists that
11:48 qu the numbers get the statistical
11:50 results a lot of times we realize that
11:53 statistics accuracy may not represent
11:56 the real world uh to use cas two
11:59 scenarios so you just mentioned some
12:01 machine learning algorithms can you go
12:04 into how Marvis uses them for
12:05 troubleshooting so Marvis utiliz
12:08 multiple machine learning algorithms
12:10 like decision tree logistic regression
12:14 lstm and just a few right XG boosts and
12:18 the sharly those is all the different
12:20 techniques inside the ml Ai and so I can
12:24 give you specific one example like how
12:27 we utilize lstm that's uh you know
12:30 launch the neuron networks to do the
12:32 normal detection so why we want to use
12:36 the neuron Network such a complex
12:38 approach to do the normal detection the
12:40 reason is that we want to be able to
12:42 capture the seasonality think about it
12:44 if you're a um Camp University campuses
12:48 so during the weekend versus weekday
12:51 during the summer break versus normal
12:53 school hours the number of the Wi-Fi
12:57 devices is different so the behavior
13:00 activity on your campus is different in
13:02 order to identify a normal normally that
13:05 we need the machine learning model is
13:08 sophisticated enough to identify the
13:10 regular pattern you know because it's
13:13 weekend versus uh the during the normal
13:18 and school school days so that's why we
13:21 utilize the LM which also utilize more
13:25 than two months of data as a training
13:26 data so we can identify enough patterns
13:29 for your weekend resus day then we use
13:32 that model to continuous to predict
13:35 what's going to be the next hour that
13:37 the errors events occurred on your
13:41 network if anything is deviate from our
13:44 prediction we identify that as uh
13:47 normally so this kind of just you know
13:50 one of a um high level introduction
13:53 about how we want to use the STM to do
13:56 the normal detection so that when we
13:58 identify normal so we can report the
14:00 customer we can show the supporting
14:02 evidence based on your last six weeks of
14:04 data we can show you your behavior
14:07 pattern but as of today your behavior is
14:10 different so we have a timeline to show
14:12 you the supporting evidence to tell you
14:14 something not right on your network and
14:17 can we also doing the mutual information
14:20 identify this so normally mostly come
14:22 from the particular DHCP server or could
14:25 come from a
14:26 particular W lens so that save the time
14:29 for the customers administrators to
14:31 troubleshooting the issu they can just
14:33 we can pinpoint the specific domain of
14:36 the problem so it's easier for them to
14:39 find the root cause can you talk a
14:41 little bit about Marvis actions UI
14:44 otherwise known as the Beloved octopus
14:46 so yeah so I wish you we have a better
14:48 name for the octopus UI so yes that's a
14:51 my action page that's a key
14:54 differentiator u compare Juniper with
14:57 our competitors Marv action page provide
15:00 the highly focused view about the
15:04 customers Works what their top issues so
15:07 for their administrator they should just
15:10 look at the Marv action page to identify
15:12 the issues that they need to fix and
15:15 also in the same page we provide the
15:18 recommended actions and also supporting
15:21 evidence so from our Network customers
15:23 Network administrators they don't need
15:25 to do the debugging to S into the device
15:29 and uh capture the pcaps slicing dicing
15:32 the data to find the problem and uh you
15:35 know find the what's a problem what's
15:37 the solution we have the single point of
15:39 view given all the answers they need
15:42 second we reduce the noise we don't show
15:45 them tons of the graph tables nine
15:49 charts for them to compare the notes
15:52 here and also the answers we make sure
15:55 Mar setion is at the manageable level so
15:58 even though you manag you know thousands
16:00 of stores for retail customer versus uh
16:04 thousands of AP of the University campus
16:07 we make sure MAV action is only
16:09 manageable by the network
16:11 administrators so this is a key
16:13 differentiator we reduce the noise Make
16:16 the network administrators really focus
16:18 on the issues raised in the Marv action
16:21 page so that's a great example about how
16:24 visibility is such a big part of the
16:26 Marva solution so I want to say thank
16:28 thank you for joining us today um we
16:31 really appreciate all the knowledge that
16:33 you brought to us surely it's a great
16:35 pleasure to be here thank you Jessica so
16:38 and thank you for listening we look
16:40 forward to you joining us for our next
16:41 episode