Bob Friday Talks: From protein folding to teaching Marvis about Zoom
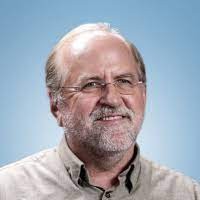
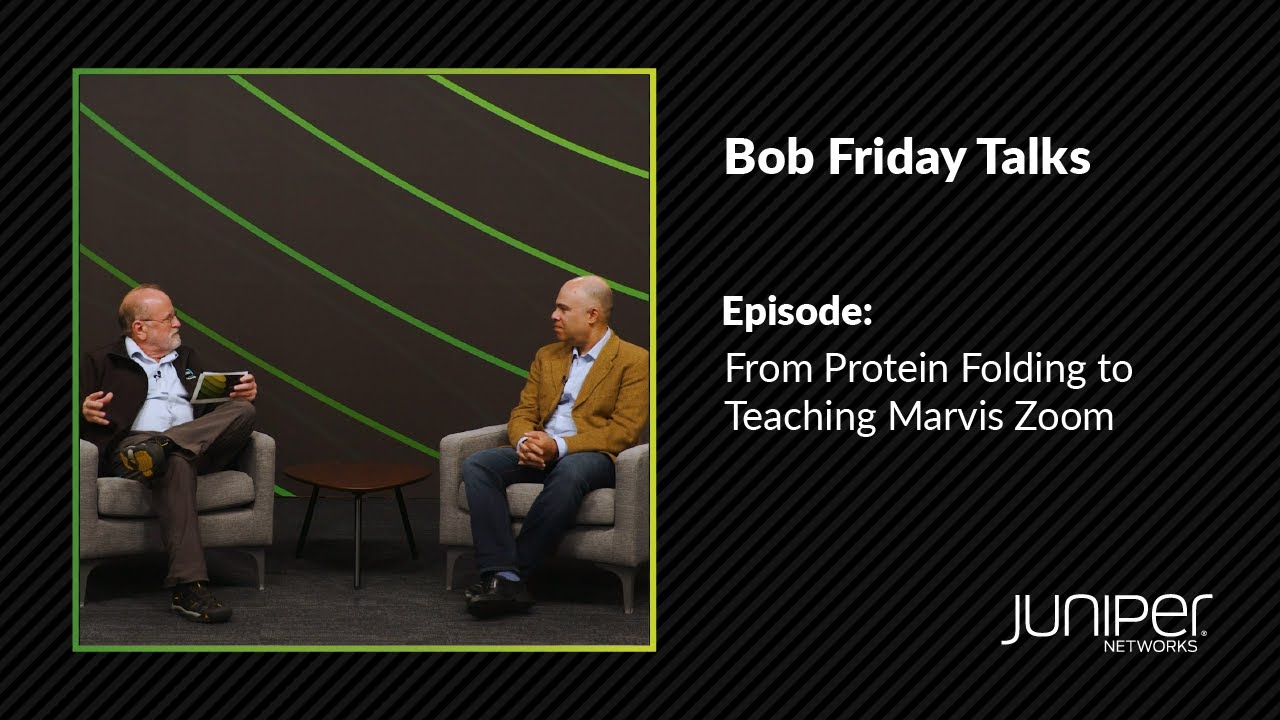
From protein folding to teaching Marvis about Zoom
In this episode of Bob Friday Talks, Bob sits down with Navraj Pannu, Data Sciences Director at Juniper Networks, for an engaging chat covering a range of topics related to AI’s role in solving problems. From his early career in structural biology to his current role in networking, Navraj’s love and excitement for applied mathematics and algorithms is clear. Pull up a chair and enjoy this fascinating conversation on combating human disease, reversing the aging process, synchrotrons, DeepMind, and, of course, solving networking-related issues.
You’ll learn
Why it is important to solve the three-dimensional atomic coordinates of DNA molecules
How AI was used to successfully predict the fold of a protein molecule
How solving problems in structural biology and Zoom and Teams calls are related
Who is this for?
Host
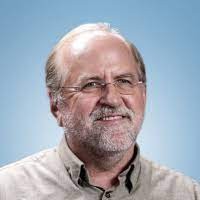
Guest speakers
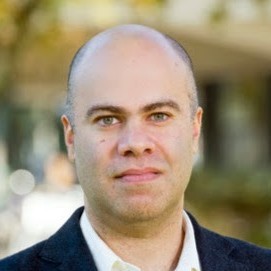
Experience More
Transcript
0:01 hello I'm Bob Friday and thanks for
0:03 joining us on another episode of Bob
0:05 Friday talks I am joined today by former
0:08 professor of structure biology at Lon
0:10 University and now director of data
0:12 science here at Juniper Miss naaj panu
0:16 today we're going to be discussing his
0:17 journey from professorship to network
0:20 data scientists welcome naaj you know
0:23 maybe we can start our conversation
0:24 today a little bit about how did you you
0:26 know what got you in be a professor I
0:30 think what got me into being a professor
0:32 but also the transition to from
0:35 structural biology to networking is my
0:37 love of mathematics that's a common link
0:40 I love Applied Mathematics I love
0:42 solving problems with mathematics
0:44 testing out the problems by writing
0:47 software and then validating it okay now
0:50 now structured biology you know exactly
0:52 what does that mean so you were working
0:54 on so what we were trying to do was
0:59 developing algorithms to solve the
1:01 three-dimensional Atomic coordinates of
1:04 protein or DNA molecules so why is that
1:07 important essentially they are the
1:10 building blocks of life with that
1:12 knowledge of the three-dimensional
1:14 structure we can understand how the
1:16 protein functions and the basis for
1:18 diseases so many diseases are caused by
1:21 mutations in that protein with that
1:23 structure we can correlate to the
1:25 function and then develop drugs to
1:28 combat disease Okay so and I've read a
1:30 couple of your papers it sounds like you
1:32 know you know before joining us you're
1:33 working on solving the problem of
1:35 immortality aging you know I almost
1:38 regret hiring you you know well know so
1:42 tell me exactly how the proteins aging
1:44 all relate to each other so at the
1:47 fundamental level aging or cancer is
1:51 caused by damage in DNA or can be caused
1:55 through damage in DNA we our body and
1:58 has a whole system
2:00 of DNA repair proteins that repair
2:03 protein repair damage DNA all the time
2:07 so that these mutations aren't
2:09 propagated to have malfunctioning
2:11 proteins and as we get older or if we're
2:15 exposed
2:16 to radiation for example Sun the DNA
2:20 damages more and hence we have these
2:23 problems so I one of the uh aspects I
2:27 was working on in the Netherlands was in
2:30 collaboration with experts trying to
2:33 determine these DNA repair proteins and
2:36 understanding the mechanism of action
2:38 okay so I've got to ask you now so
2:41 you're working on networking trying to
2:42 solve networking problems and Building
2:44 Solutions on par with domain experts how
2:47 close were you to solving this aging
2:49 problem oh I think I was more into uh
2:53 solving the particular DNA repair
2:55 Pathways associated with certain
2:57 diseases the Aging problem is still
3:00 ongoing trying to understand how we can
3:03 reverse aging it's an active area of
3:05 research at the moment and but with more
3:08 knowledge that we have on all the
3:10 different Pathways and how you know our
3:14 DNA can become damag the more we'll get
3:17 to solving this problem okay I don't
3:19 want to feel too bad about hiring you
3:21 like you know solving aging that's a
3:22 pretty critical problem to be solve now
3:24 I understand also that you know when
3:26 you're working on this problem really AI
3:29 wasn't around at the time maybe describe
3:32 a little bit to the audience you know
3:33 what techniques I know you were visiting
3:35 linear accelerators and stuff whatever
3:37 you know when the hell did you need to
3:39 go to linear accelerator for so we
3:42 employed statistical methods in order to
3:45 solve uh the structures of proteins so
3:48 what we had to do first was collect the
3:50 experimental data so we first grew
3:54 crystals of the protein of interest and
3:56 then we would take it to for example
3:59 synchrotron
4:00 at that synchrotrons we had access to
4:04 highly intense x-ray beams with that we
4:07 could collect a defraction pattern that
4:09 defraction pattern gave us the
4:11 information we needed to solve the
4:14 three-dimensional structure of
4:16 proteins and through statistical methods
4:19 we are then able to determine the atomic
4:22 coordinates and um analyze the molecules
4:26 okay so you were building a big
4:28 simulator you're were trying to build
4:29 simulation to simulate how all these
4:31 proteins and amino acids were folding
4:33 was that the basic technique so indeed
4:36 we developed a model in order to
4:39 determine the atomic coordinates but it
4:41 was based on the experimental data but
4:43 then as I moved to the Bay Area a
4:47 transition occurred and that was
4:49 actually using AI methods So based on
4:53 the hundreds of thousands of protein
4:55 structures solved Deep Mind and Google
4:59 got involved to try to solve destruction
5:02 try try to solve structures without the
5:05 need to go to the synchrotron to collect
5:08 the experimental data so they trained a
5:10 model to try to predict the fold of a
5:13 protein molecule and they were very
5:15 successful at doing that okay so this is
5:17 the transition when you decided to
5:19 transition from academic to back into
5:21 industry and data science you know maybe
5:25 a little bit more about that transition
5:27 and you know is this a case where AI
5:29 actually put you out of business you
5:30 know you how many years were you working
5:32 on this protein problem I had started as
5:34 an undergraduate in
5:37 1996 developing algorithms and applying
5:40 them to protein structures um I was very
5:45 interested in AI yet I realized in order
5:48 to do it and understand AI methods
5:52 coming to the Bay Area would be the best
5:55 solution for me so indeed it was a huge
5:57 transition going from academic to an
6:00 industrial environment but at the end it
6:02 was all about the mathematics and
6:05 another Advantage coming to the Bay Area
6:08 you have access to a tremendous amount
6:11 of data with that data for example what
6:14 we have at Juniper Mist we can tackle
6:17 big problems and model the situation
6:20 okay so so the the team at Google deep
6:22 mine actually were able to solve this
6:24 problem with some sort of deep learning
6:26 AI technique and eliminating the need
6:28 for the simulator the models yes you
6:30 know can you give the audience a little
6:32 bit about you know do you how much do
6:34 you know about deep mine and what type
6:36 of models were they using to you know
6:38 solve this protein problem similar to
6:40 what we see with chat gbt or these
6:42 different type of models the models are
6:44 not Transformer based but they are deep
6:47 learning they um so in the case of GPT
6:51 we have billions of data parameters for
6:54 the case of Deep Mind and their deep
6:57 learning model they have access to maybe
7:00 100,000 but they probably truncated
7:02 their training data to a bit less but
7:05 there's a great deal of information
7:07 within each of those structures and they
7:09 were able to develop a Target function
7:12 that um understood the protein folding
7:15 problem and then validate the model
7:18 since we they had access to all this
7:20 data so this was a revolution because
7:23 previously as you mentioned most people
7:25 were just trying to they considered it a
7:27 big optimization problem they weren't
7:29 looking at the vast amount of data
7:31 available and they were just thinking oh
7:33 if I optimize this problem and I search
7:35 All Through parameter space maybe I
7:38 could get to the answer they ignored all
7:40 the data that my colleagues my fellow
7:43 structural biologists had and that was a
7:46 difference that deep mind okay so so now
7:49 you're at Juniper miss you know you're
7:52 working on trying to build a solution
7:54 that can manage operate networks on par
7:57 with human domain experts you know you
8:00 know one minute you're trying to solve
8:02 aging proteins now we're overall trying
8:04 to solve build something on par of human
8:07 it domain experts similarities proteins
8:10 networking I think the key similarity is
8:14 just the scientific approach you know
8:17 you have to define a problem you have to
8:20 know the data that you have and the
8:22 limitations so in the case of juniper
8:24 Mist we had access to both zoom and
8:28 teams data
8:29 this gave us an indication of the
8:31 quality of the data but thanks to your
8:35 efforts in part we had all this data in
8:38 the cloud that we can combine with that
8:40 data so we can determine which network
8:43 parameters or client parameters are
8:45 affecting Zoom calls so we have
8:47 developed a model to do this an AI based
8:51 model and because we have access to
8:54 millions of data we're able to then
8:57 train a very accurate model and then
9:00 more importantly validate that model you
9:03 know I make a barrel of wine and you
9:04 know what I've learned is that organic
9:07 chemistry is a hell of a lot harder than
9:09 Wireless you know trying to make a good
9:11 bottle of wine wireless wireless is much
9:13 easier you know what do you think
9:15 solving the protein problem solving the
9:17 networking problem same level easier or
9:20 harder that's a great question by the
9:22 way the best approach is just to
9:25 iteratively improve those methods so at
9:28 the start of the protein folding problem
9:30 people as you mentioned were just trying
9:32 to simulate the model right they weren't
9:36 using the vast amount of data but then
9:38 they learned to use all the data um
9:42 there's still some networking problems
9:44 that exist that we will tackle in the
9:47 future we started off with getting this
9:50 label data from zoom and teams we had a
9:53 well-defined problem we had a huge data
9:56 set and then we could try to explain the
10:00 issues now the powerful thing we have
10:03 all of this expertise at Juniper mist
10:06 and they could validate that problem as
10:08 we validated whether we came up with the
10:10 correct solution or not and that's I
10:13 think the key we have the endtoend
10:16 process all the way up to validation so
10:20 I realize I might not have answered your
10:22 problem completely like what is more
10:25 difficult but you know this I think is a
10:28 big first step to having AI methods in
10:32 networking including like the post
10:35 connection problem which we can then
10:37 create even more problems in general
10:40 problems and maybe on the journey give
10:43 the audience a little bit you know you
10:45 know in terms of building a model that
10:46 can accurately predict zooming teams you
10:49 know where are you on the journey you
10:51 know can you actually accurately predict
10:52 a zoom teams user experience now or are
10:55 we still working on that we are able to
10:57 accurately predict a
11:00 zoom um client minute so for example
11:04 what do I mean by that if a client were
11:06 to run have a zoom call we can predict
11:10 for example the latency or the packet
11:13 loss that client is um experiencing or
11:17 will experience how do I know that based
11:19 on the metrics based on our validation
11:22 training data set and but what's but so
11:26 what so why what's the big deal about
11:28 predic latency and packet loss the nice
11:32 thing that comes along with AI is this
11:35 whole idea of explainability once we
11:37 have an accurate model which we know
11:39 from our statistics we can try to
11:41 explain that and that is what our
11:43 customers really like they like looking
11:46 at the feature ranking so what are the
11:48 important Network parameters so for
11:50 example is it a capacity issue is it a
11:53 coverage issue or is it a client issue
11:56 that's what our customers want and
11:58 that's uh what we're providing them so
12:01 and we validated it not only internally
12:04 but externally as well now now I've
12:06 heard you talk about shapley and you
12:08 know maybe for the data scientists in
12:10 our audience here maybe give a little
12:12 bit explanation you know Mutual
12:13 information shapley how does this
12:15 shapley help you explain the network
12:18 features responsible for the prediction
12:20 so at the core of shapley is it
12:24 takes I have this concept that I've
12:26 mentioned a few times and let me Define
12:28 it I call it a feat that's what's called
12:30 in what's used in AI what this is is
12:32 essentially a network parameter we are
12:35 using to try to predict the Model
12:37 Behavior so some example of features
12:39 that we're using are the client RSSI or
12:43 the number of clients on the AP or you
12:46 know the return trip time from a
12:49 particular AP so shapley considers every
12:53 single combination of all of those
12:55 features in order to explain which one
12:59 is dominant and that's what we want to
13:01 know which feature is dominant that's
13:04 leading to this poor Network performance
13:07 and the mathematically proven fact is
13:11 that shapley values are objective and
13:14 unbiased so we know that it can provide
13:18 this information so in contrast Mutual
13:22 information it only looks at pairwise
13:25 comparisons so you can only compare the
13:28 for example the latency from Zoom with
13:31 that particular feature you can compare
13:33 at a multivariant level okay so so it
13:36 sound like we have a we have a model we
13:38 have shapley you know maybe give the
13:40 audience a little bit how much dat how
13:42 much data did it take to train this
13:43 model we talking like terabyte is this
13:46 like chaty PT where you took the whole
13:48 internet and trained this model on so at
13:50 the moment what goes into training a
13:52 model is usually a million data points
13:55 so what I mean by a data point is that
13:58 we have the labeled latency that we're
14:02 getting from either Zoom or teams and
14:05 then we are combining that data with all
14:07 of our Juniper misted um network data so
14:11 combining that all we train the model
14:14 and we use that model to then predict
14:17 what our clients are uh experiencing you
14:21 know maybe for the audience you know an
14:23 example of how this is really helping
14:25 customers or you know the needle and the
14:27 Hast stack problem that it teams face
14:29 so we've uh uncovered a few issues but
14:32 one of the thing that we've seen that
14:34 has been quite prevalent is the idea of
14:37 a customer connected to a VPN server so
14:41 before both zoom and Microsoft teams
14:44 have identified that a VPN server can be
14:48 deleterious to a call but something that
14:51 we have discovered is that it's actually
14:53 not just the VPN server that is an issue
14:57 but actually the distance from the
14:59 client to the VPN server and if there is
15:03 a large distance say someone connecting
15:06 from India go connecting to Australia
15:10 that leads to a very poor uh video or
15:13 audio performance okay now R well I have
15:15 to say I'm starting to feel a little bad
15:17 you know solving the Aging problem
15:19 solving the network problem still not
15:20 sure I made the right decision you know
15:22 but maybe for other young professors and
15:25 data science coming out any words of
15:27 wisdom and advice on there Venture into
15:29 the data science AI world for me at
15:33 least from my experience as I started
15:35 off with I loved mathematics I found the
15:38 area that I really enjoyed doing and I
15:42 found the place that um I've always been
15:45 lucky that I found a place where I can
15:48 do this and apply it to problems which I
15:50 think are very critical to me so that's
15:54 the number one thing if you have that
15:57 passion you will be able to find
15:59 something that you enjoy doing you know
16:02 whether it's structural biology where
16:04 there's still a lot of room for
16:06 improvements whether it's networking or
16:09 you know even natural language
16:11 processing developing something like GPT
16:14 there's so many areas of AI so for I
16:19 love for example thinking about ethical
16:21 AI thinking about um the hallucination
16:25 problems of GPT how can we get around
16:27 that I mean these are all open areas and
16:30 you know there will always be room and
16:33 always be uh just keep in mind I think
16:37 the guiding principles are the
16:39 scientific uh method of
16:42 experimentation testing and validation
16:45 and then reiterating well now Raj I want
16:48 to thank you it's been a pleasure
16:49 working with you I can say and I want to
16:51 thank everyone for joining us today on
16:53 Bob Friday talks and look forward to
16:55 seeing you on the next episode