AI Data Center Networking: Fireside Chat With Fujitsu's Udo Würtz and Juniper's Raj Yavatkar
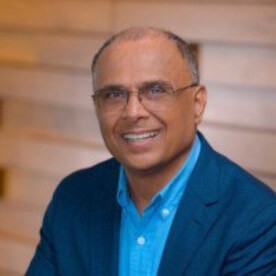
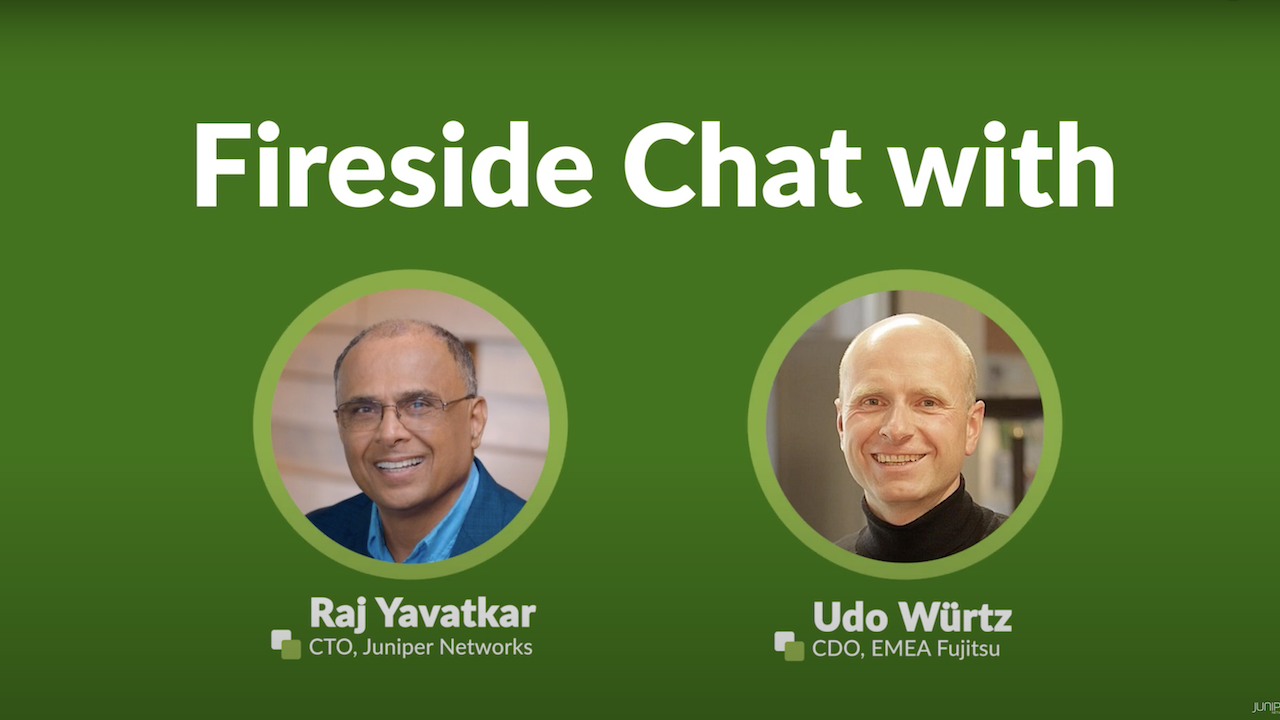
What do you really need for your AI use cases?
Watch the fireside chat between Udo Würtz, Chief Data Officer and Fellow at Fujitsu, and Raj Yavatkar, CTO of Juniper Networks, to learn about the trends, use cases, and solutions for networking the AI data center.
Learn more at our AI Data Center Networking webpage.
You’ll learn
How you can use an AI test drive to analyze how your AI infrastructure will behave prior to building
Why you may not need an expensive, custom-built AI platform for most use cases
Who is this for?
Host
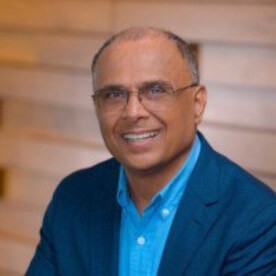
Guest speakers
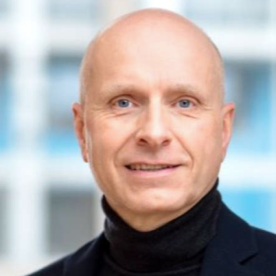
Experience More
Transcript
0:04 [Music]
0:15 most important technology trend is in
0:19 Enterprises today which are
0:22 undergoing big digital transformation
0:25 across the business processes and
0:28 artificial intelligence and machine
0:29 learning form the core components of
0:31 that digital
0:33 transformation digital transformation is
0:35 being applied across many functions in
0:37 the Enterprise such as it R&D and even
0:41 functions such as Finance HR legal and
0:45 so on today I'm very pleased to host wo
0:49 Woods Chief data officer and fellow at
0:52 Fujitsu which is our most strategic
0:54 partner at Juniper so welcome thank you
0:58 Raj and um it's really a honor to be
1:01 here and talk to all of those High
1:02 skilled people and see all the latest
1:05 Innovations from juniper so I feel very
1:08 honored to be your guest here well thank
1:10 you very happy to have you here uh now I
1:13 have talked to many Enterprise customers
1:15 who are going through such a
1:16 transformation and trying to apply
1:18 machine learning and AI but they find it
1:21 very hard to do it themselves and have a
1:25 AI Solution on Prem to be able to apply
1:28 that for this digital trans
1:30 yeah so I'm curious how are you guys
1:33 helping your customers go through that
1:35 transformation yeah that that's a good
1:38 question and I think the problem with AI
1:41 if you would like to say so is that it's
1:43 very hard to determine the right size of
1:46 an
1:46 infrastructure so it could be that your
1:49 investment is too large which means
1:52 you're spending a lot of money for
1:53 nothing at the same point in time you
1:55 can make the wrong decision to invest in
1:58 a infrastructure rure that which doesn't
2:01 fit so therefore what we have developed
2:03 at Fujitsu together with you guys with
2:05 juder is an AI test drive so which means
2:08 customers can use this free of charge um
2:12 to bring their own IE AI projects to
2:16 life and to see how the infrastructure
2:19 behaves how long does it take to do all
2:21 of those trainings with a specific
2:23 amount of data um inference time latency
2:26 and all of those stuff and this really
2:29 helps customer to make the right
2:31 decision um to invest the right money
2:34 into the right infrastructure at the end
2:36 of the day we have uh two AI test drives
2:39 available one is within the European
2:41 Union and uh at the data center in
2:44 Frankfurt and the other is located in uh
2:47 London at
2:49 ftera and this is where we are uh going
2:52 forward also demonstrating how we can
2:54 train models in different countries and
2:57 bring it then from one side to the other
2:59 and finalize the model so that we can do
3:01 all of those inference at the end of the
3:04 day and and and sharing the data between
3:06 countries so that's what we are doing
3:08 there that's very good I like the name
3:10 AI test strip it's very apt because now
3:13 customers can try it out yeah so as you
3:15 expose this AI test drive platform to
3:18 many customers yes what are the
3:20 networking requirements that you're
3:22 discovering of course it has to be fast
3:25 that is very important to make sure that
3:28 the data uh can feed into the system as
3:31 as fast as possible we are focusing on
3:34 Open Standards so this is really an very
3:38 important topic um as well um we have
3:43 different layers um for the storage for
3:47 the management layers for the uh the
3:50 user interfaces for the the training
3:53 faces and so on so um this is what we
3:56 are targeting for and really making sure
3:59 that the the Network really fits all of
4:01 those requirements I see is there any
4:04 way we can
4:05 help yeah so what we have done together
4:08 uh with juniper is we really have
4:11 implemented the the the complete Juniper
4:14 Network stack to this AI test drive and
4:18 um on one hand we have established all
4:20 the layers that we need um to what I
4:24 said um doing all the management of
4:26 those um devices to servers it's a end
4:29 it's a containerized environment right
4:31 so we are using zuu for this zuu rancha
4:35 uh we have storage units on this and of
4:38 course the uh the network stack and the
4:40 network stack is really playing a major
4:42 role as the the ports which is um
4:45 technology term um saying that we have
4:48 to provide um capabilities to those
4:51 containers which are by themselves
4:53 running the AI workloads um and those
4:58 those Technologies those infrastructure
5:00 they have to fit very perfectly together
5:02 and really making sure that AI training
5:05 can be efficient as possible um so what
5:09 we are focusing now is to enhance this
5:12 type of infrastructure with your AI
5:15 capabilities which are really remarkable
5:17 right so I have never seen a network
5:20 where I can ask the network what's going
5:22 on if something goes wrong and the
5:25 network is telling me what's where's
5:26 where's the issue and so we can fix it
5:29 very quick
5:30 and also making sure that the
5:31 configuration is almost on that level
5:34 that we have um yeah planned and and
5:37 considered when we have started with the
5:39 AI infrastructure right uh with abstra
5:42 technologies that you have and and all
5:44 of those types so this is really this is
5:46 really great to see and this is from my
5:48 perspective really unique of course we
5:50 are sitting here and talking with
5:51 juniper so of course we would say is
5:54 great but honestly it is great and this
5:56 is really outstanding it's not only a
5:59 piece of of network where you have some
6:00 cables it's really intelligent and I
6:02 think this makes the difference you know
6:04 there are a lot of switches out there in
6:05 the market but the the AI on top of it
6:08 really makes the difference makes the
6:10 difference also to companies with
6:12 respect to the lack of stuff and skills
6:14 right so we see this everywhere I'm from
6:16 Europe and in Europe we have those big
6:18 issues um and even if you would like to
6:21 hire all of those Specialists they're
6:22 simply not available on the market not
6:24 enough skills yeah and honestly they
6:27 will not be available over the next
6:28 years and therefore you must have
6:31 Intelligence on your system doesn't
6:33 matter as the service the storage but
6:35 especially also for the network layer
6:37 which is super complex and where an
6:40 issue may have an significant impact to
6:43 your production right and I think this
6:46 is really where you are on a on a
6:48 extremely good way no thank you I think
6:50 you pointed out really well that as part
6:53 of providing the networking
6:55 infrastructure for artificial
6:56 intelligence and ml workloads we're also
6:58 applying it by by providing this
7:00 conversational interface you can
7:02 communicate with the network
7:03 infrastructure in natural language ask
7:05 the questions and get responses
7:06 including troubleshooting that's a very
7:08 good point like Che chpt so to speak
7:10 that's right apply generative AI in that
7:13 St so um going back to the AI test drive
7:17 platform you mentioned um can you share
7:20 a little bit more about how your
7:22 customers are using that platform yes so
7:25 um of course we have uh a lot of
7:27 customer projects meanwhile
7:29 um we have a customer um which is
7:33 operating highways as an example and
7:35 where we have in the European Union the
7:37 so-called Aria which is alternative fuel
7:40 infrastructure regulation so which means
7:42 you have to think about infrastructure
7:45 to um recharge electric vehicles as an
7:48 example and and much more uh but to do
7:51 this first of all you have to identify
7:54 those vehicles and then think about
7:56 where charging stations should be
7:58 implemented at at the same point in time
8:00 those use cases um will also end in a
8:04 situation where you have to bring
8:06 additional services on the table it
8:08 starts with surveys but also what about
8:11 bookings of hotels restaurants and
8:13 whatever it could be right and also uh
8:15 changing exchanging data in with other
8:18 countries maybe that's that's assumption
8:20 right now therefore right now they are
8:22 focusing on on on on one country um but
8:26 um this is really where where we have a
8:29 clear understanding when we are doing
8:31 testings in this example we have um a
8:34 close collaboration with Intel and when
8:36 we have done the first considerations
8:38 for this customer um what should be the
8:41 right infrastructure the customer should
8:43 Target for without having any data from
8:45 the customer it was really about the
8:47 technology itself we have determined the
8:50 um and estimated the right size of the
8:53 infrastructure at the II test drive and
8:55 this has worked and Intel gave us
8:57 support from the HQ in Santa Clara and
9:00 also from Portland and we have done all
9:02 of those testings and uh we achieved
9:06 significant improvements also in the
9:08 detection as an example we have done a
9:11 seon 3 test on the platform 30
9:13 detections per second which is okayish
9:16 but now with the c on 4 we have achieved
9:19 more than 5,000 detections per second
9:21 and we have done those testings on the
9:23 AI test drive together with the Intel
9:25 guys and this was really clear
9:27 demonstration how can improve AI with
9:30 those capabilities uh we are now
9:33 focusing a use case on the healthc care
9:35 field um to detect people in an
9:38 emergency situation this is a in a very
9:41 early phase right now this is together
9:43 with a partner where we are focusing on
9:46 how we can go ahead with this use case
9:49 um how it should look like what we also
9:51 do in the uh with the AI test drive is
9:54 having um applications where customers
9:57 can play with so sentiment analyzes as
10:00 an example you're receiving a feedback
10:02 from a customer and you have to judge is
10:04 this positive negative in case it's
10:06 negative somebody has to take care of
10:07 this um but also um analyzes of calls as
10:11 an example think about a call center you
10:13 would like to improve the quality um
10:16 when in conversation so that you can
10:17 really identify this was positive this
10:20 was negative this is how we could
10:21 improve this and so on so it's a mixture
10:24 so to speak projects and also
10:27 playgrounds so to speak for customers
10:29 where they can do the first steps with
10:31 AI that's very impressive now just to
10:33 switch gears I want to go back to
10:35 networking requirements there's a lot of
10:38 debate right now in the industry whether
10:40 we should use ethernet infin band for
10:43 this uh machine learning workload uh
10:45 clusters do you have what is your take
10:47 on that I'm a big fan of Open
10:50 Standards sorry to say and honestly um
10:54 so which means ethernet right so and and
10:57 honestly um in my
10:59 opinion um the markets are moving in so
11:02 different ways now we are facing large
11:06 language models um because of cost and
11:10 and maybe
11:11 also discussions regarding where's the
11:14 data and public cloud service Etc a lot
11:16 of customers are focusing on pre-trained
11:18 models in uh on-prem environment in a
11:21 hybrid environment and um we never have
11:25 we're facing an issue with the existing
11:28 infrastru structure uh to the opposite
11:32 it's super fast so we have 100 Gig
11:35 connectivity between all the service in
11:38 our cluster which is a kubernetes
11:40 cluster steered by sou Rancher uh we
11:43 have net app storage in the background
11:45 with 200 gig connectivity it's really
11:47 goes like this do this and um this is
11:50 really perfect so and what I would like
11:54 to say is you don't know what will be
11:56 the workload tomorrow and then you all
11:59 of a sudden you have focused on specific
12:01 technology you invested a huge amount of
12:03 money and for the time when you have
12:06 done this of course the performance was
12:09 perfect but maybe tomorrow something
12:11 will change and all of a sudden you
12:13 realize that the infrastructure of
12:15 yesterday is probably okay but maybe a
12:19 different one might be better so
12:21 therefore I'm really focusing on on Open
12:24 Standards um that you can connect with
12:26 the existing infrastructure of your your
12:29 company where you don't have to think
12:30 about how to bring the data from here to
12:32 here and so on and this is um really
12:35 what what what I could but I would
12:37 recommend no that's good I think because
12:39 you pointed out very important thing uh
12:42 ethernet is open so you can Source from
12:44 multiple vendors technolog is constantly
12:46 evolving ethernet has been around for so
12:48 long now it continues to evolve with new
12:50 functionality new speeds and feeds uh we
12:53 are also finding out to meet the
12:55 requirements of large language model
12:58 training that you mentioned yes ethernet
13:00 can provide non-blocking High throughput
13:02 yeah and we can do lots of techniques
13:05 based on existing ethernet standards so
13:08 really looking forward to continue to
13:10 push this open technology because we
13:12 believe in open ecosystem yeah and in
13:14 our discussions in 42% of all our
13:17 customer meetings customers are talking
13:18 about large language models and in the
13:21 uh we we had the chat before right um
13:24 and we had last week The Tech
13:27 Community in Europe and we have
13:30 demonstrated how to train an llm even on
13:35 a on a workstation which is not a big
13:38 thing right um of course it could be
13:41 more complex and so on and then you need
13:43 maybe some servers to do the job but
13:45 what I would like to say is you also
13:47 have to think about what's the
13:49 performance that you really need for
13:51 your use case right not everybody wants
13:53 to build a software for self-driving
13:55 cars a lot of companies that I know they
13:57 would like to do quality Assurance as an
14:00 example or Thro detection or autom ML
14:04 and all of those Technologies and in I
14:06 would say more than 90% of the cases you
14:09 don't need this High sophisticated
14:11 high-end infrastructure with all those
14:13 very expensive components um those
14:17 customers they have really and this is
14:19 the majority they can really focus on
14:21 Open Standards so apart from the
14:23 performance right another thing that
14:24 customers worry about is uh cost of
14:27 operational experience so how do we how
14:30 do you think we can lower the cost of
14:33 operational expenses when it comes to
14:36 AI yeah that's a good topic um so we see
14:41 a lot of
14:43 automation um anable as an example right
14:46 so where you guys also have all the
14:48 connections so we can steer the overall
14:51 infrastructure stack from an AI
14:52 perspective as well as from a from a
14:54 network perspective um this is really
14:57 playing a major role in this respect
15:00 what we have done on our platform is we
15:03 have implemented additional modules as
15:04 an example Cube flow uh Cube flow is um
15:08 a tool where you can Implement a lot of
15:11 processes
15:12 workflows um where you can bring the
15:15 Daily Business of a data scientist into
15:18 the system making sure even if you have
15:20 teams of data scientists and they are
15:22 doing trainings with a huge amount of
15:24 data you can separate those data against
15:27 each other so cannot see the data one
15:29 team cannot see the data from the other
15:31 team as an example which is important uh
15:33 they can play with the data they can um
15:36 uh they can train models they can bring
15:38 it to production they can push the
15:40 button and then it's published to the
15:42 edge where we are doing the inference
15:44 and then grabbing the data back and then
15:46 doing the training of the model again
15:48 and um I think this is really also
15:50 important for customers right because
15:52 it's not only the single use case that
15:54 you have and for one use case you need
15:57 additional external specialist for
15:59 another one you have internal specialist
16:02 and this is where you need also a
16:03 management layer on on top of AI again
16:06 here I'm a big fan of of Open Standards
16:08 open source um the software is open
16:11 source we give recommendations to
16:13 customers how to install it and in case
16:15 they are going for reference
16:17 architecture such as AI test drive they
16:19 will get it for free from us and they
16:21 can do the installation by their own and
16:23 then they know how to do Ai and how to
16:25 bring this to life well this has been
16:28 wonderful so to close can you uh uh say
16:31 something about how our partnership
16:33 could evolve and how can we continue to
16:36 help you yeah it's simply great so I
16:40 know when we had the first meeting here
16:43 and I said oh well Juniper will we we
16:47 will see the latest switches or
16:49 something like this and it was it was
16:53 really mindblowing in the sense that you
16:55 started talking about Ai and how the
16:58 network is steered with AI and all of
17:00 those Technologies and I said oh wait a
17:02 minute so you don't want to count ports
17:05 anymore or something like this and we
17:07 had two days and it was not enough and
17:10 uh we have really a great collaboration
17:14 um I'm from Europe and and doing the
17:15 European business um it's in Europe it's
17:18 perfect we have um Here regular meetings
17:22 in the US so we are getting all the
17:23 insights of the latest Technologies
17:26 which helps us a lot also giving the
17:28 right recommendations to customers what
17:30 kind of invest uh they should do and
17:33 really making sure they spent the money
17:35 wisely uh to the right infrastructure so
17:38 this is this is really great and from my
17:41 personal perspective I think junipa is
17:43 really at the Forefront of Technology
17:45 especially when it comes to Ai and
17:48 steering the overall stack with AI and
17:51 also one comment here in this respect
17:53 let's face it um you guys have done a
17:55 great job with the network uh um we are
17:59 doing the same thing with Service and
18:01 Storage everything everywhere they comes
18:03 together Soo thank you for joining us
18:07 and sharing your insights this has been
18:09 a wonderful chat thank you again yeah
18:12 thank you rash and uh it's it's really
18:14 always as I said a honor to be here and
18:17 I'm really looking forward for the next
18:19 year what's what's new on the table so
18:22 this year was perfect and um yeah
18:24 looking forward next time thank you
18:26 thank you we look forward to
18:35 thanks